What Are the Most Common Python Basic Interview Questions?
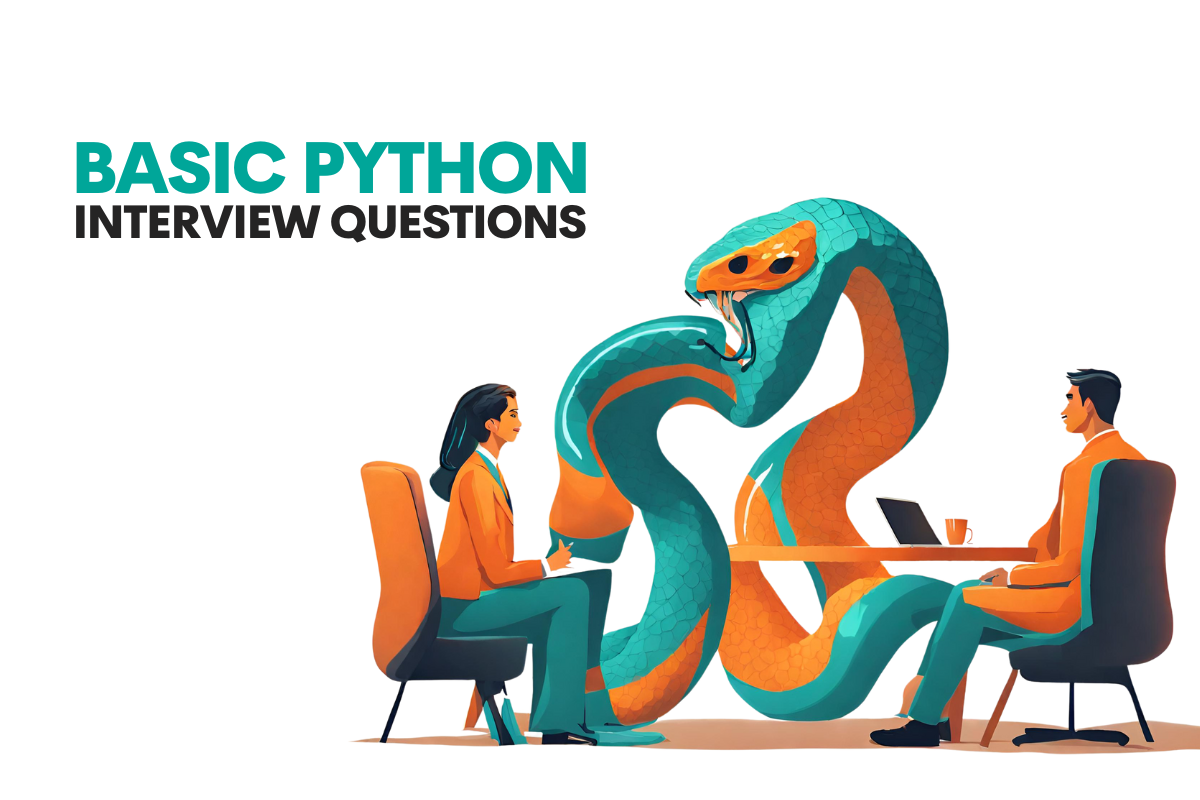
Categories
This article covers key Python interview questions for beginners, focusing on basics and data handling in Python. Let's dive in!
Did you know that Python is now the most used programming language? As of October 2022, more people use Python than C or Java. This fact comes from the TIOBE Index, a famous ranking for programming languages.
Another fact that, Python's popularity keeps growing fast. Every year, it gets 22% more users. By 2022, over four million developers were using Python on GitHub.
In this article, we will talk about the most common Python questions in job interviews, especially for beginners. We will look at basic things and also how to work with data in Python, buckle up and let’s get started!
Basic Python Interview Question #1: Find out search details for apartments designed for a sole-person stay
This question asks us to identify the search details for apartments that are suitable for just one person to stay in by Airbnb.
Find the search details made by people who searched for apartments designed for a single-person stay.
Link to the question: https://platform.stratascratch.com/coding/9615-find-out-search-details-for-apartments-designed-for-a-sole-person-stay
Let’s see our data.
id | price | property_type | room_type | amenities | accommodates | bathrooms | bed_type | cancellation_policy | cleaning_fee | city | host_identity_verified | host_response_rate | host_since | neighbourhood | number_of_reviews | review_scores_rating | zipcode | bedrooms | beds |
---|---|---|---|---|---|---|---|---|---|---|---|---|---|---|---|---|---|---|---|
12513361 | 555.68 | Apartment | Entire home/apt | {TV,"Wireless Internet","Air conditioning","Smoke detector","Carbon monoxide detector",Essentials,"Lock on bedroom door",Hangers,Iron} | 2 | 1 | Real Bed | flexible | FALSE | NYC | t | 89% | 2015-11-18 | East Harlem | 3 | 87 | 10029 | 0 | 1 |
7196412 | 366.36 | Cabin | Private room | {"Wireless Internet",Kitchen,Washer,Dryer,"Smoke detector","First aid kit","Fire extinguisher",Essentials,"Hair dryer","translation missing: en.hosting_amenity_49","translation missing: en.hosting_amenity_50"} | 2 | 3 | Real Bed | moderate | FALSE | LA | f | 100% | 2016-09-10 | Valley Glen | 14 | 91 | 91606 | 1 | 1 |
16333776 | 482.83 | House | Private room | {TV,"Cable TV",Internet,"Wireless Internet",Kitchen,"Free parking on premises","Pets live on this property",Dog(s),"Indoor fireplace","Buzzer/wireless intercom",Heating,Washer,Dryer,"Smoke detector","Carbon monoxide detector","First aid kit","Safety card","Fire extinguisher",Essentials,Shampoo,"24-hour check-in",Hangers,"Hair dryer",Iron,"Laptop friendly workspace","translation missing: en.hosting_amenity_49","translation missing: en.hosting_amenity_50","Self Check-In",Lockbox} | 2 | 1 | Real Bed | strict | TRUE | SF | t | 100% | 2013-12-26 | Richmond District | 117 | 96 | 94118 | 1 | 1 |
1786412 | 448.86 | Apartment | Private room | {"Wireless Internet","Air conditioning",Kitchen,Heating,"Suitable for events","Smoke detector","Carbon monoxide detector","First aid kit","Fire extinguisher",Essentials,Shampoo,"Lock on bedroom door",Hangers,"translation missing: en.hosting_amenity_49","translation missing: en.hosting_amenity_50"} | 2 | 1 | Real Bed | strict | TRUE | NYC | t | 93% | 2010-05-11 | Williamsburg | 8 | 86 | 11211 | 1 | 1 |
14575777 | 506.89 | Villa | Private room | {TV,Internet,"Wireless Internet","Air conditioning",Kitchen,"Free parking on premises",Essentials,Shampoo,"translation missing: en.hosting_amenity_49","translation missing: en.hosting_amenity_50"} | 6 | 2 | Real Bed | strict | TRUE | LA | t | 70% | 2015-10-22 | 2 | 100 | 90703 | 3 | 3 |
We are looking at information about apartments made for one person. We use two tools, pandas and numpy, which are like helpers for managing and understanding data.
- First, we focus on the data that shows apartments for one person. We check where 'accommodates' is equal to 1.
- Then, we also want these apartments to be of a specific type - 'Apartment'. So, we look for where 'property_type' says 'Apartment'.
- By combining these two conditions, we get details only for apartments perfect for one person.
- We store this specific information in a new place called 'result'.
In simple words, we are just picking out the apartment searches that match two things: meant for one person and are apartments. Let’s see the code.
import pandas as pd
import numpy as np
result = airbnb_search_details[(airbnb_search_details['accommodates'] == 1) & (airbnb_search_details['property_type'] == 'Apartment')]
Here is the expected output.
id | price | property_type | room_type | amenities | accommodates | bathrooms | bed_type | cancellation_policy | cleaning_fee | city | host_identity_verified | host_response_rate | host_since | neighbourhood | number_of_reviews | review_scores_rating | zipcode | bedrooms | beds |
---|---|---|---|---|---|---|---|---|---|---|---|---|---|---|---|---|---|---|---|
5059214 | 431.75 | Apartment | Private room | {TV,"Wireless Internet","Air conditioning",Kitchen,"Free parking on premises",Breakfast,Heating,"Smoke detector","Carbon monoxide detector","First aid kit","Fire extinguisher",Essentials,Shampoo,"Lock on bedroom door",Hangers,"Laptop friendly workspace","Private living room"} | 1 | 3 | Real Bed | strict | FALSE | NYC | f | 2014-03-14 00:00:00 | Harlem | 0 | 10030 | 2 | 1 | ||
10923708 | 340.12 | Apartment | Private room | {TV,Internet,"Wireless Internet","Air conditioning",Kitchen,"Pets live on this property",Cat(s),"Buzzer/wireless intercom",Heating,"Family/kid friendly",Washer,"Smoke detector","Carbon monoxide detector","First aid kit","Fire extinguisher",Essentials} | 1 | 1 | Real Bed | strict | FALSE | NYC | t | 100% | 2014-06-30 00:00:00 | Harlem | 166 | 91 | 10031 | 1 | 1 |
1077375 | 400.73 | Apartment | Private room | {"Wireless Internet",Heating,"Family/kid friendly","Smoke detector","Carbon monoxide detector","Fire extinguisher",Essentials,Shampoo,Hangers,Iron,"Laptop friendly workspace","translation missing: en.hosting_amenity_50"} | 1 | 1 | Real Bed | moderate | TRUE | NYC | t | 2015-04-04 00:00:00 | Sunset Park | 1 | 100 | 11220 | 1 | 1 | |
13121821 | 501.06 | Apartment | Private room | {TV,"Cable TV",Internet,"Wireless Internet","Air conditioning",Kitchen,Heating,"Smoke detector","First aid kit",Essentials,Hangers,"Hair dryer",Iron,"Laptop friendly workspace"} | 1 | 1 | Real Bed | flexible | FALSE | NYC | f | 2014-09-20 00:00:00 | Park Slope | 0 | 11215 | 1 | 1 | ||
19245819 | 424.85 | Apartment | Private room | {Internet,"Wireless Internet",Kitchen,"Pets live on this property",Dog(s),Washer,Dryer,"Smoke detector","Fire extinguisher"} | 1 | 1 | Real Bed | moderate | FALSE | SF | t | 2010-03-16 00:00:00 | Mission District | 12 | 90 | 94110 | 1 | 1 |
Basic Python Interview Question #2: Users Activity Per Month Day
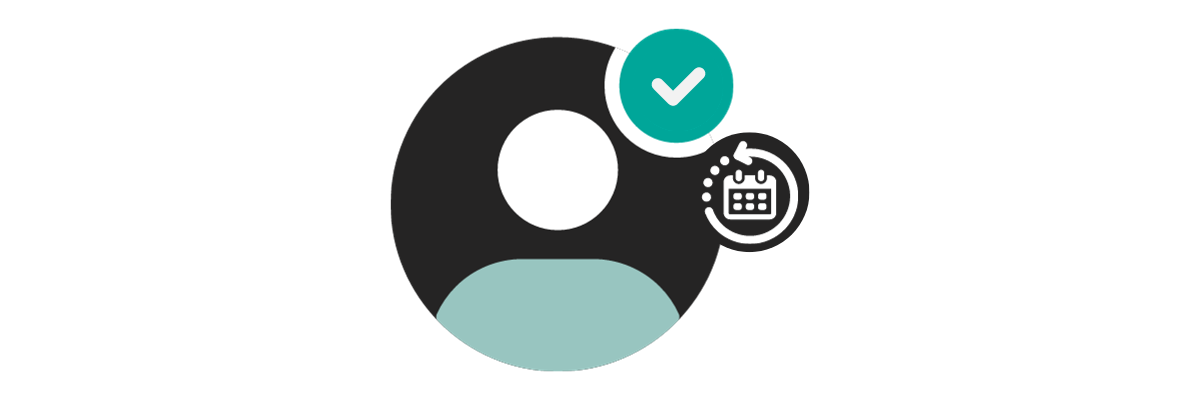
This question is about figuring out how active users are on different days of the month on Facebook. Specifically, it asks for a count of how many posts are made each day, asked by Meta/Facebook.
Interview Question Date: January 2021
Return a distribution of users activity per day of the month. By distribution we mean the number of posts per day of the month.
Link to the question: https://platform.stratascratch.com/coding/2006-users-activity-per-month-day
Let’s see our data.
post_id | poster | post_text | post_keywords | post_date |
---|---|---|---|---|
0 | 2 | The Lakers game from last night was great. | [basketball,lakers,nba] | 2019-01-01 |
1 | 1 | Lebron James is top class. | [basketball,lebron_james,nba] | 2019-01-02 |
2 | 2 | Asparagus tastes OK. | [asparagus,food] | 2019-01-01 |
3 | 1 | Spaghetti is an Italian food. | [spaghetti,food] | 2019-01-02 |
4 | 3 | User 3 is not sharing interests | [#spam#] | 2019-01-01 |
We are analyzing how often users post on Facebook during different days of the month. We use pandas, a tool for data handling, to do this.
- First, we change the post dates into a format that's easy to work with.
- Then, we look at these dates and focus on the day part of each date.
- For each day, we count how many posts were made.
- We then make a new table called 'user_activity' to show these counts.
- Finally, we make sure this table is easy to read by resetting its layout.
Simply, we are counting Facebook posts for each day of the month and presenting it in a clear table. Let’s see the code.
import pandas as pd
result = facebook_posts.groupby(pd.to_datetime(facebook_posts['post_date']).dt.day)['post_id'].count().to_frame('user_activity').reset_index()
Here is the expected output.
post_date | user_activity |
---|---|
1 | 3 |
2 | 3 |
Basic Python Interview Question #3: Customers Who Purchased the Same Product
This question involves finding customers who bought the same furniture items, asked by Meta. It asks for details like the furniture's product ID, brand name, the unique customer IDs who bought each item, and how many different customers bought each item.
The final list should start with the furniture items bought by the most customers
Interview Question Date: February 2023
In order to improve customer segmentation efforts for users interested in purchasing furniture, you have been asked to find customers who have purchased the same items of furniture.
Output the product_id
, brand_name
, unique customer ID's who purchased that product, and the count of unique customer ID's who purchased that product. Arrange the output in descending order with the highest count at the top.
Link to the question: https://platform.stratascratch.com/coding/2150-customers-who-purchased-the-same-product
Let’s see our data.
product_id | promotion_id | cost_in_dollars | customer_id | date | units_sold |
---|---|---|---|---|---|
1 | 1 | 2 | 1 | 2022-04-01 | 4 |
3 | 3 | 6 | 3 | 2022-05-24 | 6 |
1 | 2 | 2 | 10 | 2022-05-01 | 3 |
1 | 2 | 3 | 2 | 2022-05-01 | 9 |
2 | 2 | 10 | 2 | 2022-05-01 | 1 |
product_id | product_class | brand_name | is_low_fat | is_recyclable | product_category | product_family |
---|---|---|---|---|---|---|
1 | ACCESSORIES | Fort West | N | N | 3 | GADGET |
2 | DRINK | Fort West | N | Y | 2 | CONSUMABLE |
3 | FOOD | Fort West | Y | N | 1 | CONSUMABLE |
4 | DRINK | Golden | Y | Y | 3 | CONSUMABLE |
5 | FOOD | Golden | Y | N | 2 | CONSUMABLE |
We are focusing on customers who are interested in buying furniture. We use pandas and numpy, which help us organize and analyze data.
- We start by combining two sets of data: one with order details (online_orders) and the other with product details (online_products). We match them using 'product_id'.
- Then, we only keep the data that is about furniture.
- We simplify this data to show only product ID, brand name, and customer ID, removing any duplicates.
- Next, we count how many different customers bought each product.
- We create a new table showing these counts along with product ID, brand name, and customer ID.
- Lastly, we arrange this table so the products with the most unique buyers are at the top.
In short, we are finding and listing furniture items based on how popular they are with different customers, showing the most popular first. Let’s see the code.
import pandas as pd
import numpy as np
merged = pd.merge(online_orders, online_products, on="product_id", how="inner")
merged = merged.loc[merged["product_class"] == "FURNITURE", :]
merged = merged[["product_id", "brand_name", "customer_id"]].drop_duplicates()
unique_cust = (
merged.groupby(["product_id"])["customer_id"]
.nunique()
.to_frame("unique_cust_no")
.reset_index()
)
result = pd.merge(merged, unique_cust, on="product_id", how="inner").sort_values(
by="unique_cust_no", ascending=False
)
Here is the expected output.
product_id | brand_name | customer_id | unique_cust_no |
---|---|---|---|
10 | American Home | 2 | 3 |
10 | American Home | 1 | 3 |
10 | American Home | 3 | 3 |
8 | Lucky Joe | 3 | 1 |
11 | American Home | 1 | 1 |
Basic Python Interview Question #4: Sorting Movies By Duration Time
This basic Python interview question requires sorting a list of movies based on how long they last, with the longest movies shown first, asked by Google.
Interview Question Date: May 2023
You have been asked to sort movies according to their duration in descending order.
Your output should contain all columns sorted by the movie duration in the given dataset.
Link to the question: https://platform.stratascratch.com/coding/2163-sorting-movies-by-duration-time
Let’s see our data.
show_id | title | release_year | rating | duration |
---|---|---|---|---|
s1 | Dick Johnson Is Dead | 2020 | PG-13 | 90 min |
s95 | Show Dogs | 2018 | PG | 90 min |
s108 | A Champion Heart | 2018 | G | 90 min |
s163 | Marshall | 2017 | PG-13 | 118 min |
s174 | Snervous Tyler Oakley | 2015 | PG-13 | 83 min |
We need to organize movies based on their duration, from longest to shortest. We use pandas, a tool for handling data, to do this.
- We start by focusing on the movie duration. We extract the duration in minutes from the 'duration' column.
- We change these duration values into numbers so that we can sort them.
- Next, we sort the whole movie catalogue based on these duration numbers, putting the longest movies at the top.
- After sorting, we remove the column with the duration in minutes since we don't need it anymore.
In simple terms, we are putting the movies in order from the longest to the shortest based on their duration. Let’s see the code.
import pandas as pd
movie_catalogue["movie_minutes"] = (
movie_catalogue["duration"].str.extract("(\d+)").astype(float)
)
result = movie_catalogue.sort_values(by="movie_minutes", ascending=False).drop(
"movie_minutes", axis=1
)
Here is the expected output.
show_id | title | release_year | rating | duration |
---|---|---|---|---|
s8083 | Star Wars: Episode VIII: The Last Jedi | 2017 | PG-13 | 152 min |
s6201 | Avengers: Infinity War | 2018 | PG-13 | 150 min |
s6326 | Black Panther | 2018 | PG-13 | 135 min |
s8052 | Solo: A Star Wars Story | 2018 | PG-13 | 135 min |
s8053 | Solo: A Star Wars Story (Spanish Version) | 2018 | PG-13 | 135 min |
Basic Python Interview Question #5: Find the date with the highest opening stock price
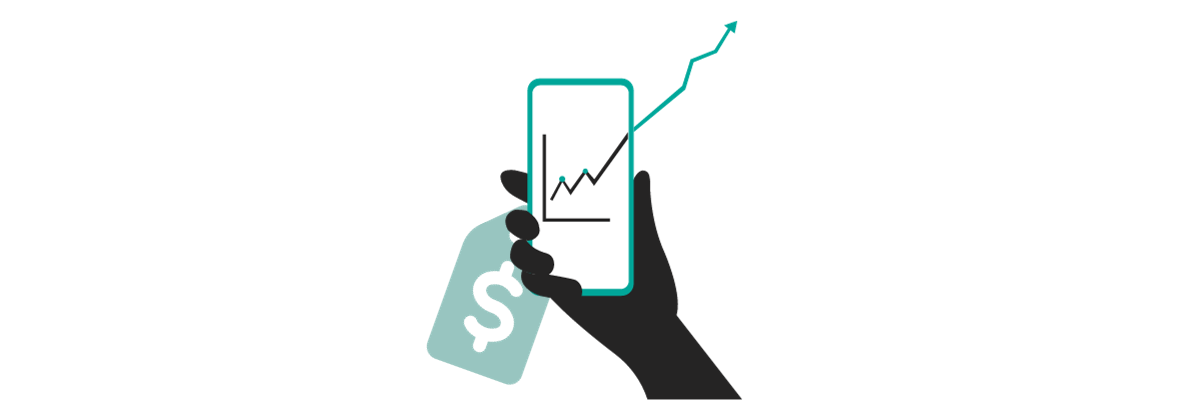
This question asks us to identify the date when a stock (presumably Apple's, given the dataframe name) had its highest opening price, by Apple.
Find the date when Apple's opening stock price reached its maximum
Link to the question: https://platform.stratascratch.com/coding/9613-find-the-date-with-the-highest-opening-stock-price
Let’s see our data.
date | year | month | open | high | low | close | volume | id |
---|---|---|---|---|---|---|---|---|
2012-12-31 | 2012 | 12 | 510.53 | 506.5 | 509 | 532.17 | 23553255 | 273 |
2012-12-28 | 2012 | 12 | 510.29 | 506.5 | 508.12 | 509.59 | 12652749 | 274 |
2012-12-27 | 2012 | 12 | 513.54 | 506.5 | 504.66 | 515.06 | 16254240 | 275 |
2012-12-26 | 2012 | 12 | 519 | 506.5 | 511.12 | 513 | 10801290 | 276 |
2012-12-24 | 2012 | 12 | 520.35 | 506.5 | 518.71 | 520.17 | 6276711 | 277 |
We are looking to find the day when a specific stock had its highest starting price. We use pandas and numpy, tools for data analysis, and handle dates with datetime and time.
- We start with the stock price data, named 'aapl_historical_stock_price'.
- Then, we adjust the dates to a standard format ('YYYY-MM-DD').
- Next, we search for the highest opening price in the data. The 'open' column shows us the starting price of the stock on each day.
- Once we find the highest opening price, we look for the date(s) when this price occurred.
- The result shows us the date or dates with this highest opening stock price.
In summary, we are identifying the date when the stock started trading at its highest price. Let’s see the code.
import pandas as pd
import numpy as np
import datetime, time
df = aapl_historical_stock_price
df['date'] = df['date'].apply(lambda x: x.strftime('%Y-%m-%d'))
result = df[df['open'] == df['open'].max()][['date']]
Here is the expected output.
date |
---|
2012-09-21 |
Basic Python Interview Question #6: Low Fat and Recyclable
This question wants us to calculate what proportion of all products are both low fat and recyclable by Meta/Facebook.
Interview Question Date: October 2021
What percentage of all products are both low fat and recyclable?
Link to the question: https://platform.stratascratch.com/coding/2067-low-fat-and-recyclable
Let’s see our data.
product_id | product_class | brand_name | is_low_fat | is_recyclable | product_category | product_family |
---|---|---|---|---|---|---|
1 | ACCESSORIES | Fort West | N | N | 3 | GADGET |
2 | DRINK | Fort West | N | Y | 2 | CONSUMABLE |
3 | FOOD | Fort West | Y | N | 1 | CONSUMABLE |
4 | DRINK | Golden | Y | Y | 3 | CONSUMABLE |
5 | FOOD | Golden | Y | N | 2 | CONSUMABLE |
We need to find out how many products are both low in fat and can be recycled. We use pandas for data analysis.
- First, we look at the products data and pick out only those that are marked as low fat ('Y' in 'is_low_fat') and recyclable ('Y' in 'is_recyclable').
- We then count how many products meet both these conditions.
- Next, we compare this number to the total number of products in the dataset.
- We calculate the percentage by dividing the number of low fat, recyclable products by the total number of products and multiplying by 100.
Simply put, we are figuring out the fraction of products that are both healthy (low fat) and environmentally friendly (recyclable) and expressing it as a percentage, let’s see the code.
df = facebook_products[(facebook_products.is_low_fat == 'Y') & (facebook_products.is_recyclable == 'Y')]
result = len(df) / len(facebook_products) * 100.0
Here is the expected output.
Basic Python Interview Question #7: Products with No Sales
This question asks us to find products that have not been sold at all by Amazon. We need to list the ID and market name of these unsold products.
Interview Question Date: May 2022
Write a query to get a list of products that have not had any sales. Output the ID and market name of these products.
Link to the question: https://platform.stratascratch.com/coding/2109-products-with-no-sales
Let’s see our data.
cust_id | prod_sku_id | order_date | order_value | order_id |
---|---|---|---|---|
C274 | P474 | 2021-06-28 | 1500 | O110 |
C285 | P472 | 2021-06-28 | 899 | O118 |
C282 | P487 | 2021-06-30 | 500 | O125 |
C282 | P476 | 2021-07-02 | 999 | O146 |
C284 | P487 | 2021-07-07 | 500 | O149 |
prod_sku_id | prod_sku_name | prod_brand | market_name |
---|---|---|---|
P472 | iphone-13 | Apple | Apple IPhone 13 |
P473 | iphone-13-promax | Apple | Apply IPhone 13 Pro Max |
P474 | macbook-pro-13 | Apple | Apple Macbook Pro 13'' |
P475 | macbook-air-13 | Apple | Apple Makbook Air 13'' |
P476 | ipad | Apple | Apple IPad |
We are looking for products that haven't been sold yet. We use a merge function, a way of combining two sets of data, for this task.
- We start by joining two data sets: 'fct_customer_sales' (which has sales details) and 'dim_product' (which has product details). We link them using 'prod_sku_id', which is like a unique code for each product.
- We then look for products that do not have any sales. We do this by checking for missing values in the 'order_id' column. If 'order_id' is missing, it means the product wasn't sold.
- After finding these products, we create a list showing their ID ('prod_sku_id') and market name ('market_name').
In simple words, we are identifying products that have never been sold and listing their ID and the market they are associated with, let’s see the code.
sales_and_products = fct_customer_sales.merge(dim_product, on='prod_sku_id', how='right')
result = sales_and_products[sales_and_products['order_id'].isna()][['prod_sku_id', 'market_name']]
Here is the expected output.
prod_sku_id | market_name |
---|---|
P473 | Apply IPhone 13 Pro Max |
P481 | Samsung Galaxy Tab A |
P483 | Dell XPS13 |
P488 | JBL Charge 5 |
Basic Python Interview Question #8: Most Recent Employee Login Details
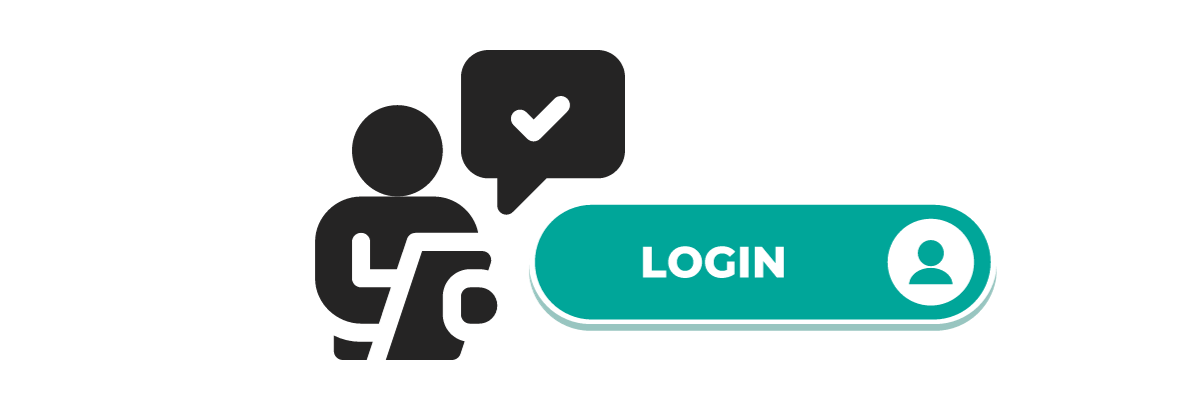
This question is about finding the latest login information for each employee at Amazon's IT department.
Interview Question Date: December 2022
Amazon's information technology department is looking for information on employees' most recent logins.
The output should include all information related to each employee's most recent login.
Link to the question: https://platform.stratascratch.com/coding/2141-most-recent-employee-login-details
Let’s see our data.
id | worker_id | login_timestamp | ip_address | country | region | city | device_type |
---|---|---|---|---|---|---|---|
0 | 1 | 2021-12-14 09:01:00 | 65.111.191.14 | USA | Florida | Miami | desktop |
1 | 4 | 2021-12-18 10:05:00 | 46.212.154.172 | Norway | Viken | Skjetten | desktop |
2 | 3 | 2021-12-15 08:55:00 | 80.211.248.182 | Poland | Mazovia | Warsaw | desktop |
3 | 5 | 2021-12-19 09:55:00 | 10.2.135.23 | France | North | Roubaix | desktop |
4 | 6 | 2022-01-03 11:55:00 | 185.103.180.49 | Spain | Catalonia | Alcarras | desktop |
We need to identify when each employee last logged in and gather all the details about these logins. We use pandas and numpy for data management and analysis.
- We start with the 'worker_logins' data, which records employees' login times.
- For each employee ('worker_id'), we find the most recent ('max') login time.
- We then create a new table ('most_recent') that shows the latest login time for each employee.
- Next, we merge this table with the original login data. This helps us match each employee's most recent login time with their other login details.
- We ensure that we're combining the data based on both employee ID and their last login time.
- Finally, we remove the 'last_login' column from the result as it's no longer needed.
In short, we are sorting out the most recent login for each employee and displaying all related information about that login, let’s see the code.
import pandas as pd
import numpy as np
most_recent = (
worker_logins.groupby(["worker_id"])["login_timestamp"]
.max()
.to_frame("last_login")
)
result = pd.merge(
most_recent,
worker_logins,
how="inner",
left_on=["worker_id", "last_login"],
right_on=["worker_id", "login_timestamp"],
).drop(columns=['last_login'])
Here is the expected output.
worker_id | id | login_timestamp | ip_address | country | region | city | device_type |
---|---|---|---|---|---|---|---|
1 | 20 | 2022-01-26 08:58:00 | 65.111.191.14 | USA | Florida | Miami | desktop |
2 | 14 | 2022-01-10 09:52:00 | 66.68.93.191 | USA | Texas | Austin | desktop |
3 | 16 | 2022-01-25 08:58:00 | 80.211.248.182 | Poland | Mazovia | Warsaw | desktop |
4 | 15 | 2022-01-24 08:48:00 | 46.212.154.172 | Norway | Viken | Skjetten | desktop |
5 | 3 | 2021-12-19 09:55:00 | 10.2.135.23 | France | North | Roubaix | desktop |
Basic Python Interview Question #9: Customer Consumable Sales Percentages
This Python question requires us to compare different brands based on the percentage of unique customers who bought consumable products from them, following a recent advertising campaign, asked by Meta/Facebook.
Interview Question Date: February 2023
Following a recent advertising campaign, you have been asked to compare the sales of consumable products across all brands.
Compare the brands by finding the percentage of unique customers (among all customers in the dataset) who purchased consumable products from each brand.
Your output should contain the brand_name
and percentage_of_customers
rounded to the nearest whole number and ordered in descending order.
Link to the question: https://platform.stratascratch.com/coding/2149-customer-consumable-sales-percentages
Let’s see our data.
product_id | promotion_id | cost_in_dollars | customer_id | date | units_sold |
---|---|---|---|---|---|
1 | 1 | 2 | 1 | 2022-04-01 | 4 |
3 | 3 | 6 | 3 | 2022-05-24 | 6 |
1 | 2 | 2 | 10 | 2022-05-01 | 3 |
1 | 2 | 3 | 2 | 2022-05-01 | 9 |
2 | 2 | 10 | 2 | 2022-05-01 | 1 |
product_id | product_class | brand_name | is_low_fat | is_recyclable | product_category | product_family |
---|---|---|---|---|---|---|
1 | ACCESSORIES | Fort West | N | N | 3 | GADGET |
2 | DRINK | Fort West | N | Y | 2 | CONSUMABLE |
3 | FOOD | Fort West | Y | N | 1 | CONSUMABLE |
4 | DRINK | Golden | Y | Y | 3 | CONSUMABLE |
5 | FOOD | Golden | Y | N | 2 | CONSUMABLE |
We are comparing brands to see how popular their consumable products are with customers. We use pandas for data handling.
- We begin by combining two data sets: one with customer orders (online_orders) and another with product details (online_products). We link them using 'product_id'.
- Then, we focus on consumable products by filtering the data to include only items in the 'CONSUMABLE' product family.
- For each brand, we count how many different customers bought their consumable products.
- We then calculate the percentage of these unique customers out of all customers in the dataset.
- We round these percentages to the nearest whole number for simplicity.
- Finally, we arrange the brands so that those with the highest percentage of unique customers are listed first.
In short, we are finding out which brands had the most unique customers for their consumable products, and presenting this information in an easy-to-understand percentage form, ordered from most to least popular, let’s see the code.
import pandas as pd
merged = pd.merge(online_orders, online_products, on="product_id", how="inner")
consumable_df = merged.loc[merged["product_family"] == "CONSUMABLE", :]
result = (
consumable_df.groupby("brand_name")["customer_id"]
.nunique()
.to_frame("pc_cust")
.reset_index())
unique_customers = merged.customer_id.nunique()
result["pc_cust"] = (100.0 * result["pc_cust"] / unique_customers).round()
result
Here is the expected output.
brand_name | pc_cust |
---|---|
Fort West | 80 |
Golden | 80 |
Lucky Joe | 20 |
Basic Python Interview Question #10: Unique Employee Logins
This question asks by Meta/Facebook us to identify the worker IDs of individuals who logged in during a specific week in December 2021, from the 13th to the 19th inclusive.
Interview Question Date: March 2023
You have been tasked with finding the worker IDs of individuals who logged in between the 13th to the 19th inclusive of December 2021.
In your output, provide the unique worker IDs for the dates requested.
Link to the question: https://platform.stratascratch.com/coding/2156-unique-employee-logins
Let’s see our data.
id | worker_id | login_timestamp | ip_address | country | region | city | device_type |
---|---|---|---|---|---|---|---|
0 | 1 | 2021-12-14 09:01:00 | 65.111.191.14 | USA | Florida | Miami | desktop |
1 | 4 | 2021-12-18 10:05:00 | 46.212.154.172 | Norway | Viken | Skjetten | desktop |
2 | 3 | 2021-12-15 08:55:00 | 80.211.248.182 | Poland | Mazovia | Warsaw | desktop |
3 | 5 | 2021-12-19 09:55:00 | 10.2.135.23 | France | North | Roubaix | desktop |
4 | 6 | 2022-01-03 11:55:00 | 185.103.180.49 | Spain | Catalonia | Alcarras | desktop |
We are searching for the IDs of workers who logged in between the 13th and 19th of December 2021. We use pandas, a tool for managing data, and datetime for handling dates.
- We start with the 'worker_logins' data, which has records of when workers logged in.
- First, we make sure the login timestamps are in a date format that's easy to use.
- Then, we find the logins that happened between the 13th and 19th of December 2021. We use the 'between' function for this.
- From these selected logins, we gather the unique worker IDs.
- The result will be a list of worker IDs who logged in during this specific time period.
Simply put, we are pinpointing which workers logged in during a certain week in December 2021 and listing their IDs, let’s see the code.
import pandas as pd
import datetime as dt
worker_logins["login_timestamp"] = pd.to_datetime(worker_logins["login_timestamp"])
dates_df = worker_logins[
worker_logins["login_timestamp"].between("2021-12-13", "2021-12-19")
]
result = dates_df["worker_id"].unique()
Here is the expected output.
0 |
---|
1 |
4 |
3 |
5 |
Final Thoughts
So, we've explored some of the most common basic Python interview questions. From basic syntax to complex data manipulation, we've covered topics that mirror real-world scenarios, and asked by the big tech companies.
Practice is the key to becoming not just good, but great at data science. Theory is important, but the real learning happens when you apply what you've learned. If you want to see more, here are the python interview questions.
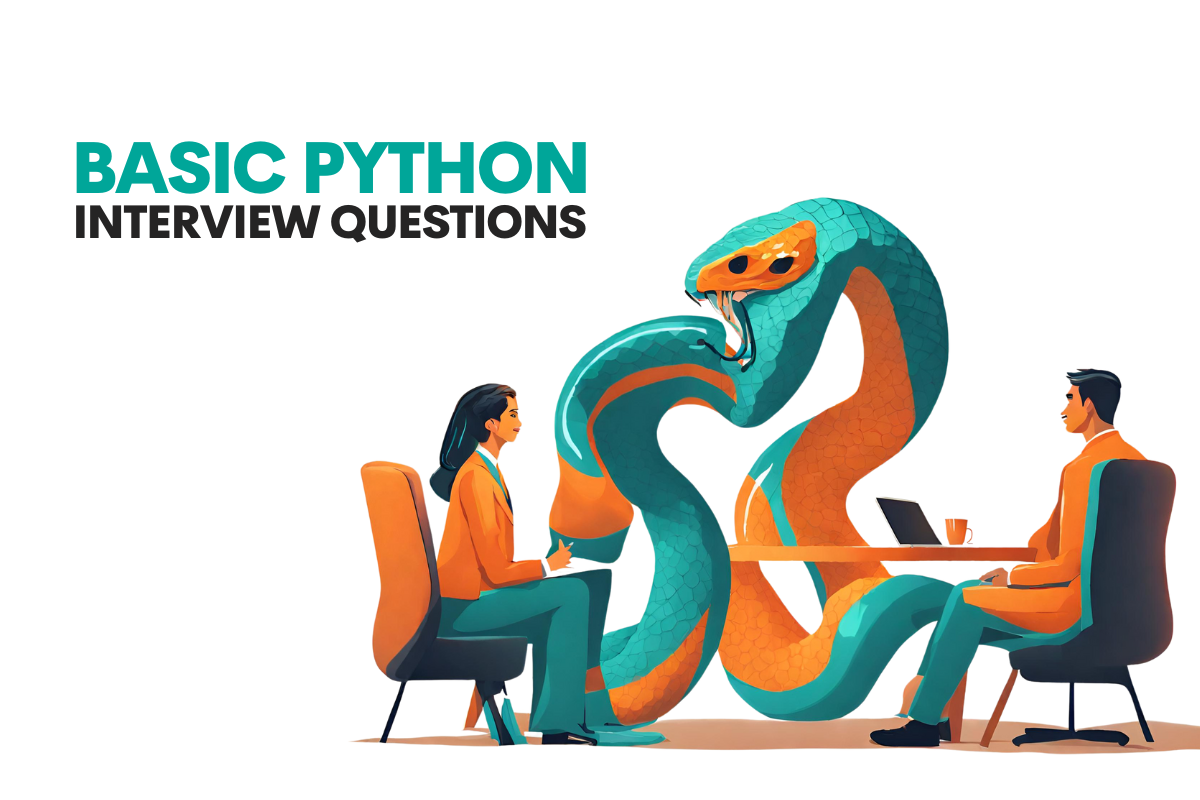