The Ultimate Guide to SQL Window Functions
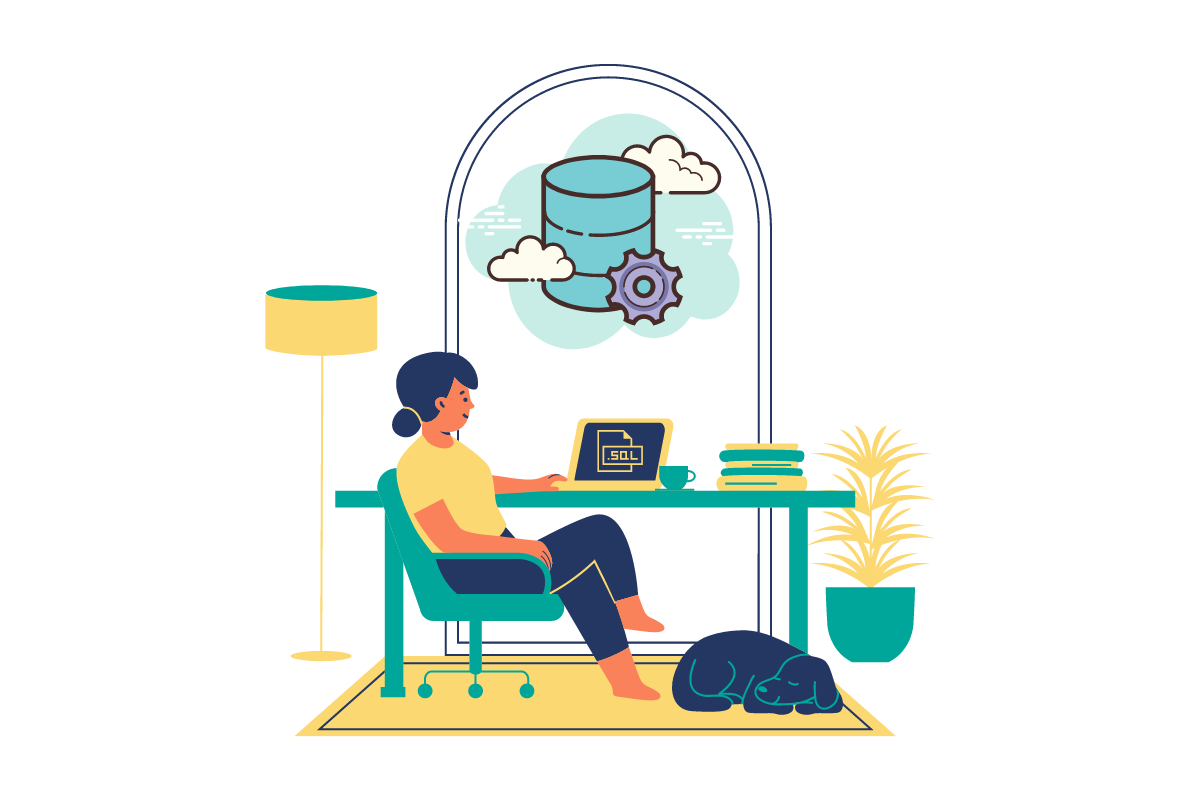
Categories
This ultimate guide is a complete overview of the types of SQL window functions, their syntax and real-life examples of how to use them in queries.
Because of how efficient SQL window functions are compared to standard SQL functions and operators, interviewers at most of the companies ask questions about them and expect the candidates to use them in their solutions. The SQL window functions are also frequently used in everyday work by data scientists and data analysts, especially when querying particularly large datasets. Their usage can not only make the SQL code faster but also clearer and easier to understand by others. However, because of the complicated syntax, arguably less intuitive than with other SQL constructions, it takes practice to master using SQL window functions in queries.
Even though SQL was first formalized in 1986, the window functions in SQL weren't added until 2003. Formally speaking, window functions use values from multiple rows to produce values for each row separately. What distinguishes window from other SQL functions, namely aggregate and scalar functions, is the keyword OVER, used to define a portion of rows the function should consider as inputs to produce an output. This portion of rows is called a ‘window’, hence the name of this family of functions.
This guide starts by introducing the 3 main types of SQL window functions, namely:
- Aggregate window functions;
- Ranking window functions;
- Value window functions.
It then lists the specific functions together with examples of their usage. After that, the guide describes some more advanced syntax concepts regarding SQL window functions such as using the EXCLUDE and FILTER clauses, frame boundaries and window chaining.
List of SQL Window Functions
There is no official division of the SQL window functions into categories but nevertheless, they are frequently being divided into two or three types. The most basic classification splits the SQL window functions into aggregate and built-in functions.
The main characteristic of the aggregate window functions is that they reuse the existing simple aggregate functions (such as COUNT() or SUM()) while changing the way in which the aggregation is defined and the format of the results. Meanwhile, the built-in functions have new names and cannot be used in another context.
The built-in SQL window functions are then sometimes divided into two different types: the ranking and value functions. The ranking window functions are used to simply assign numbers to the existing rows according to some requirements. On the other hand, the value window functions are used to copy values from other rows to other rows within the defined windows.
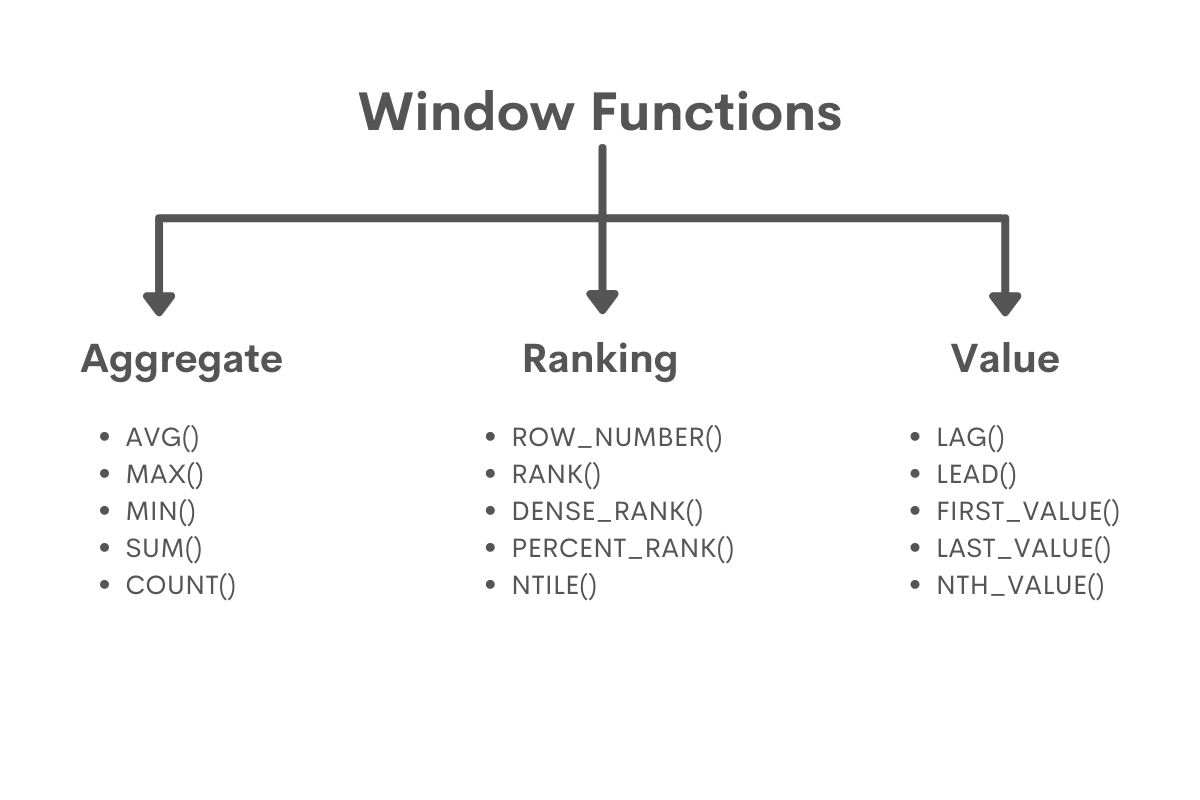
Aggregate Window Functions in SQL
As mentioned, the aggregate window functions are exactly the same as standard aggregate functions, with the most popular being AVG(), MAX(), MIN(), SUM() and COUNT(). When used in normal circumstances, these functions either apply to the entire dataset (e.g. AVG() return the mean from all the values in a column) or go pair-in-pair with a GROUP BY statement so that the function is applied to subsets or rows, depending on another variable.
As it turns out, the GROUP BY statement can be replaced by using an aggregate window function instead and specifying the aggregation conditions in the OVER clause instead. The main difference is that while a standard aggregate function reduces the number of rows to match the number of categories to which the data are aggregated, the window function does not change the number of rows, instead assigns the correct value to each row of the dataset, even if these values are the same.
To better understand the difference and the syntax of the SQL window functions, let’s consider this interview question from Twitch:
Interview Question Date: February 2021
Calculate the average session duration for each session type?
Link to the question: https://platform.stratascratch.com/coding/2011-session-type-duration
It comes with a simple table containing data about streaming sessions of various users with the session_type being either ‘streamer’ or ‘viewer’.
This question can be solved rather easily by taking the average of the differences between session end and start times, and then aggregating the results by session type which can be achieved with a GROUP BY statement.
SELECT session_type,
avg(session_end - session_start) AS duration
FROM twitch_sessions
GROUP BY session_type
This solution reduces the number of rows to match the number of distinct session types. Each row is then assigned the correct average value.
session_type | duration |
---|---|
viewer | 986 |
streamer | 411 |
But since normal aggregate functions, such as the AVG() in this case, can be replaced with window aggregate functions, let’s explore the alternative solution to this interview question. To construct a window function, the whole expression avg(session_end - session_start) can be left unchanged. To this, the keyword OVER can be added that defines the window function.
SELECT *,
avg(session_end -session_start) OVER () AS duration
FROM twitch_sessions
user_id | session_start | session_end | session_id | session_type | duration |
---|---|---|---|---|---|
0 | 2020-08-11 05:51:31 | 2020-08-11 05:54:45 | 539 | streamer | 698.5 |
2 | 2020-07-11 03:36:54 | 2020-07-11 03:37:08 | 840 | streamer | 698.5 |
3 | 2020-11-26 11:41:47 | 2020-11-26 11:52:01 | 848 | streamer | 698.5 |
1 | 2020-11-19 06:24:24 | 2020-11-19 07:24:38 | 515 | viewer | 698.5 |
2 | 2020-11-14 03:36:05 | 2020-11-14 03:39:19 | 646 | viewer | 698.5 |
Note the lack of GROUP BY clause in the query and how the window function does not reduce the number of rows, instead adds the average value to each row. The solution from above is still far from correct because the value added to each row is 698 - it is the average value between the session_end and session_start but for the entire dataset.
The next step therefore will be to define in what way the rows should be aggregated. This can be done within the OVER() clause that for now is empty. The expression will be identical as the GROUP BY statement, with the exception that another keyword, PARTITION BY, needs to be used within the OVER clause. The PARTITION BY clause is the equivalent to GROUP BY in the SQL window functions and allows to aggregate the results by another variable. Hence, the AVG() window function will look as follows:
SELECT *,
avg(session_end -session_start) OVER (PARTITION BY session_type)
AS duration
FROM twitch_sessions
user_id | session_start | session_end | session_id | session_type | duration |
---|---|---|---|---|---|
0 | 2020-08-11 05:51:31 | 2020-08-11 05:54:45 | 539 | streamer | 411 |
2 | 2020-07-11 03:36:54 | 2020-07-11 03:37:08 | 840 | streamer | 411 |
3 | 2020-11-26 11:41:47 | 2020-11-26 11:52:01 | 848 | streamer | 411 |
0 | 2020-03-11 03:01:40 | 2020-03-11 03:01:59 | 782 | streamer | 411 |
1 | 2020-11-20 06:59:57 | 2020-11-20 07:20:11 | 907 | streamer | 411 |
Note the lack of GROUP BY clause in the query and how the window function does not reduce the number of rows. Instead, it adds the correct average, different for ‘streamer’ sessions and ‘viewer’ sessions, to each row. However, the interview question asks to return an average value for each session type, thus this format of results isn’t the best for this purpose. To generate the correct output for this question, only two columns need to be selected in combination with the DISTINCT keyword to still reduce the number of rows.
SELECT DISTINCT session_type,
avg(session_end -session_start) OVER (PARTITION BY session_type)
AS duration
FROM twitch_sessions
session_type | duration |
---|---|
viewer | 986 |
streamer | 411 |
The same approach can be used with any aggregate functions. Let’s now consider this interview question from Microsoft.
Interview Question Date: November 2020
We have a table with employees and their salaries, however, some of the records are old and contain outdated salary information. Find the current salary of each employee assuming that salaries increase each year. Output their id, first name, last name, department ID, and current salary. Order your list by employee ID in ascending order.
Link to the question: https://platform.stratascratch.com/coding/10299-finding-updated-records
In other words, the task is to find a maximum salary for each employee. The standard solution to this question looks as follows:
SELECT id,
first_name,
last_name,
department_id,
max(salary)
FROM ms_employee_salary
GROUP BY id,
first_name,
last_name,
department_id
This solution uses the MAX() function and involves aggregation by four variables. This can all still be achieved using the window function.
SELECT DISTINCT id,
first_name,
last_name,
department_id,
max(salary) OVER(PARTITION BY id, first_name, last_name,
department_id)
FROM ms_employee_salary
However, in many cases when using the aggregate functions, the feature of the GROUP BY clause automatically reducing the number of rows and showing a simple summary of aggregated results becomes useful. So in which situation does the fact the window functions do not change the number of rows come in handy? The answer is: when the individual values need to be compared to aggregated values.
Let’s consider this interview question from Salesforce on average salaries.
Interview Question Date: May 2019
Compare each employee's salary with the average salary of the corresponding department. Output the department, first name, and salary of employees along with the average salary of that department.
Link to the question: https://platform.stratascratch.com/coding/9917-average-salaries
When using a standard approach with the GROUP BY clause, these questions would require two separate queries and wouldn’t be efficient. Instead, the window function can solve it easily:
SELECT department,
first_name,
salary,
AVG(salary) over (PARTITION BY department)
FROM employee;
department | first_name | salary | avg |
---|---|---|---|
Audit | Michale | 700 | 950 |
Audit | Shandler | 1100 | 950 |
Audit | Jason | 1000 | 950 |
Audit | Celine | 1000 | 950 |
Management | Allen | 200000 | 175000 |
Now, in case the next task was to calculate the difference between each employee’s salary and the department’s average, it can easily be done, even within the same query. This is the situation when aggregate window functions prove especially useful.
Ranking Window functions in SQL
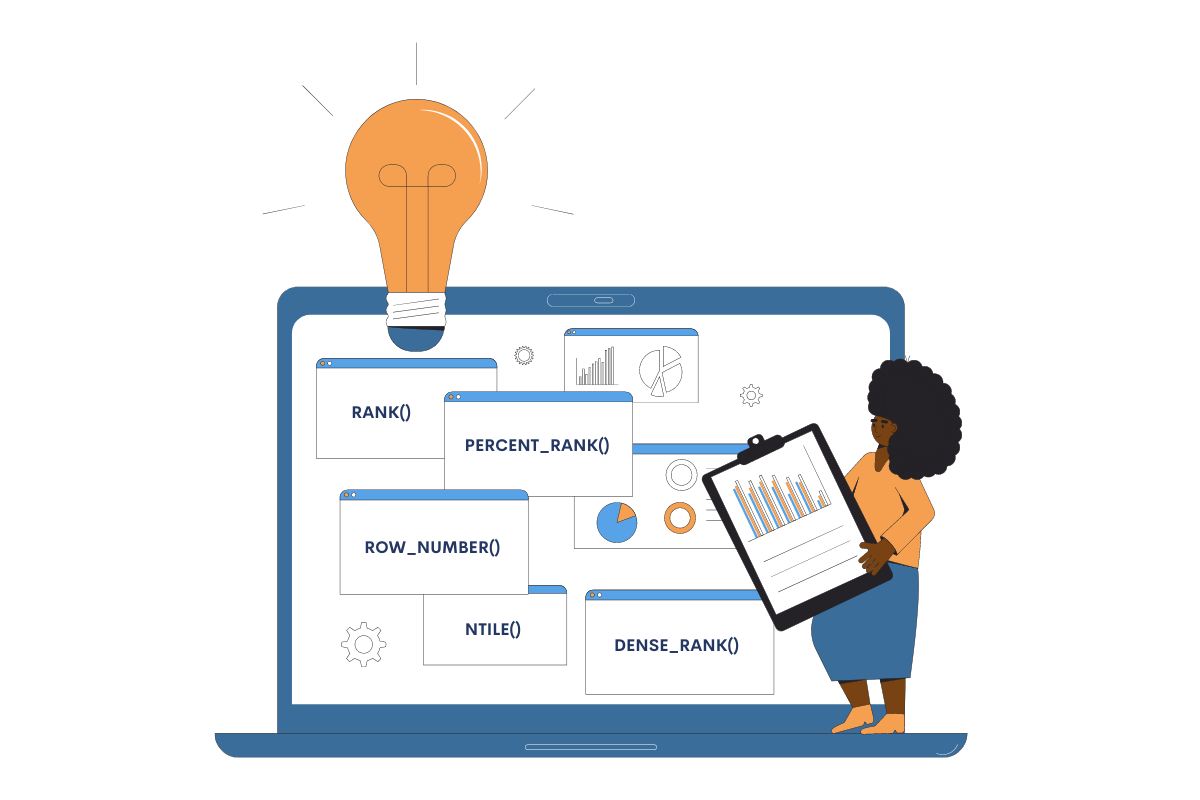
The ranking window functions are used to assign numbers to rows depending on some defined ordering. Unlike the aggregate window functions, the ranking functions have no obvious equivalents that don’t use windows. What’s more, attempting to solve for the same results but without using analytical functions would require multiple nested queries and would be largely inefficient. Therefore, the ranking window functions in SQL are the most commonly used out of the entire family. These include:
- ROW_NUMBER()
- RANK()
- DENSE_RANK()
- PERCENT_RANK()
- NTILE()
ROW_NUMBER()
The ROW_NUMBER() function is the simplest of the ranking window functions in SQL. It assigns consecutive numbers starting from 1 to all rows in the table. The order of the rows needs to be defined using an ORDER BY clause inside the OVER clause. This is, in fact, the necessary condition for all the ranking window functions: they don’t require the PARTITION BY clause but the ORDER BY clause must always be there.
Let’s consider this question from Google on SQL functions.
Interview Question Date: July 2021
Find the email activity rank for each user. Email activity rank is defined by the total number of emails sent. The user with the highest number of emails sent will have a rank of 1, and so on. Output the user, total emails, and their activity rank. Order records by the total emails in descending order. Sort users with the same number of emails in alphabetical order. In your rankings, return a unique value (i.e., a unique rank) even if multiple users have the same number of emails. For tie breaker use alphabetical order of the user usernames.
Link to the question: https://platform.stratascratch.com/coding/10351-activity-rank
Because of this last sentence the task becomes simply assigning the consecutive numbers to users based on the number of sent emails.
The solution to this question may start like this, by counting the number of emails:
SELECT from_user,
COUNT(*) as total_emails
FROM google_gmail_emails
GROUP BY from_user
ORDER BY 2 DESC
from_user | total_emails |
---|---|
32ded68d89443e808 | 19 |
ef5fe98c6b9f313075 | 19 |
5b8754928306a18b68 | 18 |
91f59516cb9dee1e88 | 16 |
55e60cfcc9dc49c17e | 16 |
Since the question instructs to assign different values to users with the same number of emails, the ranking can be assigned using the ROW_NUMBER() function. To use it, the ORDER BY statement from the previous query can simply be moved inside the OVER clause like this:
SELECT from_user,
COUNT(*) as total_emails,
ROW_NUMBER() OVER ( ORDER BY count(*) desc, from_user asc)
FROM google_gmail_emails
GROUP BY from_user
from_user | total_emails | row_number |
---|---|---|
32ded68d89443e808 | 19 | 1 |
ef5fe98c6b9f313075 | 19 | 2 |
5b8754928306a18b68 | 18 | 3 |
55e60cfcc9dc49c17e | 16 | 4 |
91f59516cb9dee1e88 | 16 | 5 |
As expected, the function simply assigned the consecutive numbers to the rows, without repeating or skipping any numbers, and it did it according to the defined order: it sorts the data by total_emails in descending order.
RANK()
The RANK() window function is more advanced than ROW_NUMBER() and is probably the most commonly used out of all SQL window functions. Its task is rather simple: to assign ranking values to the rows according to the specified ordering. Then, how is it different from the ROW_NUMBER() function?
The key difference between RANK() and ROW_NUMBER() is how the first one handles the ties, i.e. cases when multiple rows have the same value by which the dataset is sorted. The rank function will always assign the same value to rows with the same values and will skip some values to keep the ranking consistent with the number of preceding rows.
To visualize it, let’s use the same interview question from Google using RANK() about the email activity rank but this time let’s switch ROW_NUMBER() in the solution to RANK().
SELECT from_user,
COUNT(*) as total_emails,
RANK() OVER (ORDER BY count(*) desc)
FROM google_gmail_emails
GROUP BY from_user
from_user | total_emails | rank |
---|---|---|
32ded68d89443e808 | 19 | 1 |
ef5fe98c6b9f313075 | 19 | 1 |
5b8754928306a18b68 | 18 | 3 |
91f59516cb9dee1e88 | 16 | 4 |
55e60cfcc9dc49c17e | 16 | 4 |
The values in the third column are completely different than previously, even though the task remains similar: assigning consecutive values according to some ordering. In this case, the data are again sorted by total_emails in descending order. The different values are all the result of ties in the data. For instance, the first two users both have 19 total_emails. Therefore, when ranking them, it’s impossible to tell which one is the first and which one the second unless another condition (e.g. ordering alphabetically by user ID) is specified. The RANK() function solves this issue by assigning the same value to both rows.
At the same time, the function still assigns the value 3 to the third row because there are 2 other rows before it and its total_emails is different from theirs. This rule stays valid even if there are more than two rows with the same value. Note how all users with 15 total_emails have the ranking 6 but the users with 14 total_emails suddenly get a ranking value of 10. That’s because of how many users with 15 total_emails there are and because there are exactly 9 rows before the first user with 14 total_emails.
The RANK() function is especially useful when the task is to output the rows with the highest or the lowest values. This can easily be done by adding an outer query with a WHERE condition specifying that the rank value should be equal to 1. For example, the code below returns the users with the highest email activity rank. Note that it would be impossible with the ROW_NUMBER() function - it would only return one row which is not a correct answer.
SELECT from_user,
total_emails
FROM
(SELECT from_user,
COUNT(*) AS total_emails,
RANK() OVER (
ORDER BY count(*) DESC) rnk
FROM google_gmail_emails
GROUP BY from_user) a
WHERE rnk = 1
from_user | total_emails |
---|---|
32ded68d89443e808 | 19 |
ef5fe98c6b9f313075 | 19 |
DENSE_RANK()
The DENSE_RANK() function is very similar to the RANK() function with one key difference - if there are ties in the data and two rows are assigned the same ranking value, the DENSE_RANK() will not skip any numbers and will assign the consecutive value to the next row. To visualize it, here is the solution to the interview question from Google, using DENSE_RANK():
SELECT from_user,
COUNT(*) as total_emails,
DENSE_RANK() OVER (ORDER BY count(*) desc)
FROM google_gmail_emails
GROUP BY from_user
from_user | total_emails | dense_rank |
---|---|---|
32ded68d89443e808 | 19 | 1 |
ef5fe98c6b9f313075 | 19 | 1 |
5b8754928306a18b68 | 18 | 2 |
91f59516cb9dee1e88 | 16 | 3 |
55e60cfcc9dc49c17e | 16 | 3 |
Since there are two users with 19 total_emails, they are assigned the same ranking value because this is still a ranking function. However, while the RANK() function would assign a value of 3 to the third row, DENSE_RANK() assigns the ranking of 2 because it does not skip values and the number 2 hasn’t been used before.
The DENSE_RANK() is most useful when solving tasks in which several highest values need to be shown. For example, if the assignment is to output the users with top 2 total_email counts, the DENSE_RANK() can be used because it ensures that the users with the second highest total_emails will be assigned the ranking value of 2.
SELECT from_user,
total_emails
FROM
(SELECT from_user,
COUNT(*) AS total_emails,
DENSE_RANK() OVER (
ORDER BY count(*) DESC) rnk
FROM google_gmail_emails
GROUP BY from_user) a
WHERE rnk <= 2
from_user | total_emails |
---|---|
32ded68d89443e808 | 19 |
ef5fe98c6b9f313075 | 19 |
5b8754928306a18b68 | 18 |
The rule of thumb is to use RANK() when the task is to output rows with the highest or lowest ranking values and DENSE_RANK() when there is a need to output rows with several ranking values. But when the question is to simply add a ranking to data, it is not clear which function should be used but the more obvious choice is the RANK() function since it is considered the default one. However, if an ambiguity like that appears at an interview, it is best to ask an interviewer for a clarification. What’s more, some questions use certain keywords to point to one of the functions. For instance, a sentence ‘Avoid gaps in the ranking calculation’, like in this question from Deloitte, suggests using a DENSE_RANK() function.
PERCENT_RANK()
The PERCENT_RANK() function is another one from the ranking functions family that in reality uses a RANK() function to calculate the final ranking. The ranking values in case of PERCENT_RANK are calculated using the following formula: (rank - 1)/(rows - 1). Because of this, all the ranking values are scaled by the number of rows and stay between 0 and 1. Additionally, the rows with the first value are always assigned the ranking value of 0.
When applied to the interview question from Google, the PERCENT_RANK() results in the following ranking. Note that this table has 25 rows and while not all of them are shown, the ranking continues all the way to 1. This also explains the ranking values - for instance, the third row is assigned RANK() of 3 and since there are 25 rows, the formula becomes (3-1)/(25-1) = 2/24 = 0.083.
SELECT from_user,
COUNT(*) as total_emails,
PERCENT_RANK() OVER (ORDER BY count(*) desc)
FROM google_gmail_emails
GROUP BY from_user
from_user | total_emails | percent_rank |
---|---|---|
32ded68d89443e808 | 19 | 0 |
ef5fe98c6b9f313075 | 19 | 0 |
5b8754928306a18b68 | 18 | 0.083 |
91f59516cb9dee1e88 | 16 | 0.125 |
55e60cfcc9dc49c17e | 16 | 0.125 |
NTILE()
The NTILE() is the last major ranking window function in SQL but it is not very commonly used. In short, it works analogically to the ROW_NUMBER function but instead of assigning consecutive numbers to the next rows, it assigns consecutive numbers to the buckets of rows. The bucket is a collection of several consecutive rows and the number of buckets is set as a parameter of the NTILE() function - for example, NTILE(10) means that the dataset will be divided into 10 buckets.
When NTILE(10) is applied to the interview question from Google, it divides the dataset into buckets. The table has 25 rows, so each bucket should have 2,5 rows but since the bucket size needs to be an integer, this value gets rounded up to 3. Because of that each set of 3 rows will be given the next number from 1 to 10 (because of 10 buckets).
SELECT from_user,
COUNT(*) as total_emails,
NTILE(10) OVER (ORDER BY count(*) desc)
FROM google_gmail_emails
GROUP BY from_user
from_user | total_emails | ntile |
---|---|---|
32ded68d89443e808 | 19 | 1 |
ef5fe98c6b9f313075 | 19 | 1 |
5b8754928306a18b68 | 18 | 1 |
91f59516cb9dee1e88 | 16 | 2 |
55e60cfcc9dc49c17e | 16 | 2 |
Since there are 25 rows and they are divided into buckets of size 3, the last bucket, the one assigned the value of 10, will only have 2 rows. What’s more the number of buckets should always be less than the number of rows in the table, otherwise the number of rows per bucket would need to be less than 1 and since that’s not possible, each bucket would have exactly 1 row and the result would be the same as with using the ROW_NUMBER() function.
The final remark regarding NTILE() is that it should not be used to calculate percentiles. That’s because it only splits the results in the equal-sized buckets and it may happen, as in the example, that rows with different values will be assigned the same ranking. Because of that, when dealing with percentiles, it is advisable to use PERCENT_RANK() instead.
Value Window Functions in SQL
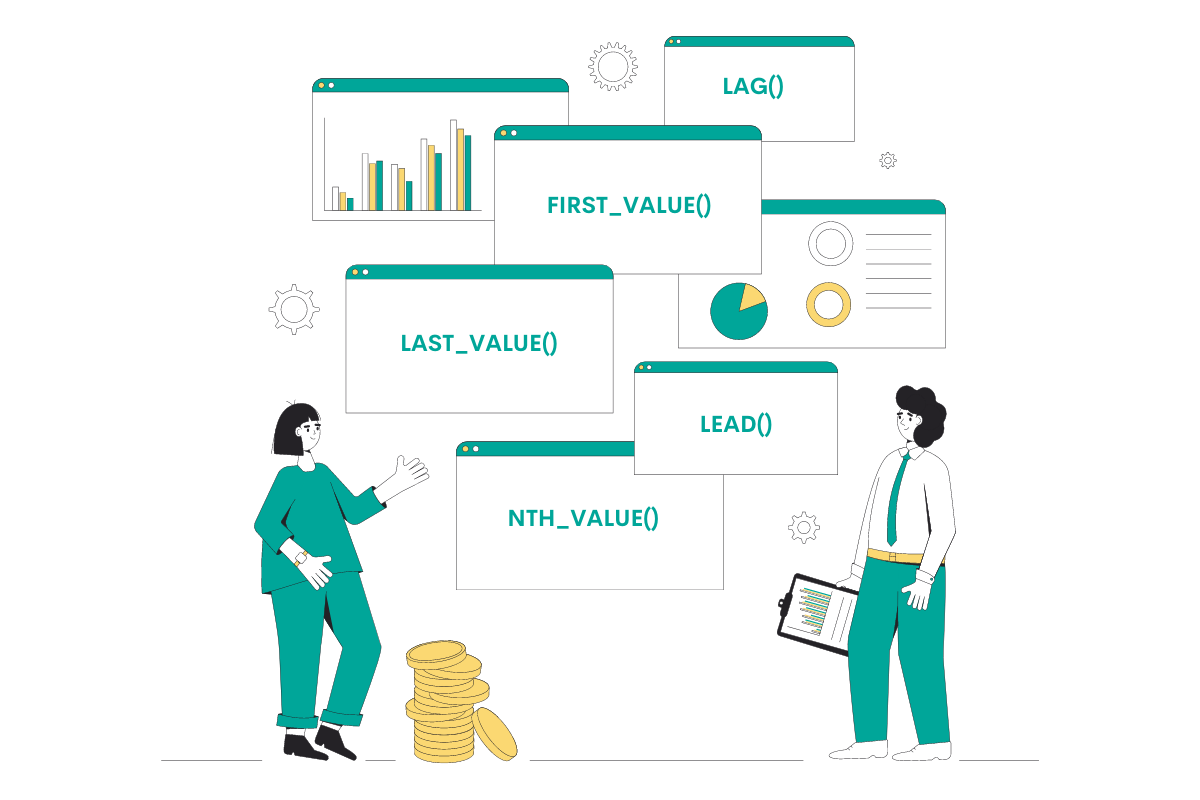
The value window functions in SQL are used to assign to rows values from other rows. Similarly to the ranking window functions and unlike the aggregate functions, the value functions have no obvious equivalents that don’t use windows. However, it is usually possible to replicate the results of these functions using two nested queries, hence, the value window functions are not that commonly used as the ranking functions. There are following value window functions in SQL:
- LAG()
- LEAD()
- FIRST_VALUE()
- LAST_VALUE()
- NTH_VALUE()
LAG()
The LAG() function is by far the most popular out of the value window functions but at the same time is rather simple. What it does is, it assigns to each row a value that normally belongs to the previous row. In other words, it allows to shift any column by one row down and allows to perform queries using this shift of values. Naturally, the ordering of the rows matters also in this case, hence, the window function will most commonly include the ORDER BY clause within its OVER( clause.
To give an example of how the LAG() function can be used, let’s consider this interview question from the City of San Francisco. It asks:
Interview Question Date: May 2018
Determine the change in the number of daily violations by calculating the difference between the count of current and previous violations by inspection date. Output the inspection date and the change in the number of daily violations. Order your results by the earliest inspection date first.
Link to the question: https://platform.stratascratch.com/coding/9740-daily-violation-counts
To answer this interview question, there are three details that need to be extracted from the original dataset: the date, the number of violations for each date, and for each date, the number of violations that were detected on the previous day. The first two are rather simple to extract with the following query:
SELECT inspection_date::DATE,
COUNT(violation_id)
FROM sf_restaurant_health_violations
GROUP BY 1
The other task is to get the number of violations that were detected on the previous day. To achieve this, the results of the query above need to be sorted by date, so that the previous inspection date is always one row above, and the column with the COUNT() values needs to be shifted by one row down. This is what the LAG() function has been created to solve.
SELECT inspection_date::DATE,
COUNT(violation_id),
LAG(COUNT(violation_id)) OVER(ORDER BY inspection_date::DATE)
FROM sf_restaurant_health_violations
GROUP BY 1
inspection_date | count | lag |
---|---|---|
2015-09-08 | 1 | |
2015-09-15 | 0 | 1 |
2015-09-18 | 0 | 0 |
2015-09-23 | 0 | 0 |
2015-09-28 | 1 | 0 |
As it can be seen, the LAG() function easily shifted the values from the second column by one row. For instance, the second row means that on September 15th 2015, there were 0 violations (second column) but on the previous inspection date (September 8th), there was 1 violation (third column). Note that because of the specificity of this dataset, not all dates exist in the table because inspections do not occur every day.
Another interesting detail to realise is that when using the LAG() function, the first row in the dataset or in a partition to which the function is applied, will always be assigned the NULL value. This may cause issues in some cases and is something that the solution should account for.
To complete the solution for this example interview question, one more column needs to be added, namely, the difference between the count of current and previous violations by inspection date. This can be achieved either in an outer query or even in the same query by subtracting the value in the third column from the value in the second column.
We’ll remove two columns from the previous step, as they are not required by the question.
SELECT inspection_date::DATE,
COUNT(violation_id) - LAG(COUNT(violation_id)) OVER(
ORDER BY inspection_date::DATE)
diff
FROM sf_restaurant_health_violations
GROUP BY 1;
inspection_date | diff |
---|---|
2015-09-08 | |
2015-09-15 | -1 |
2015-09-18 | 0 |
2015-09-23 | 0 |
2015-09-28 | 1 |
LEAD()
The LEAD() window function is the exact opposite of the LAG() function because while LAG() returns for each row the value of the previous row, the LEAD() function will return the value of the following row. In other words, as LAG() shifts the values 1 row down, LEAD() shifts them 1 row up. Otherwise, the functions are identical in how they are called or how the order is defined.
If the interview question from the above asked to calculate the difference between the count of current and next violations by inspection date, it could be solved using the LEAD() function as follows:
SELECT inspection_date::DATE,
COUNT(violation_id),
LEAD(COUNT(violation_id)) OVER(
ORDER BY inspection_date::DATE),
COUNT(violation_id) - LEAD(COUNT(violation_id)) OVER(
ORDER BY inspection_date::DATE)
diff
FROM sf_restaurant_health_violations
GROUP BY 1
inspection_date | count | lead | diff |
---|---|---|---|
2015-09-08 | 1 | 0 | 1 |
2015-09-15 | 0 | 0 | 0 |
2015-09-18 | 0 | 0 | 0 |
2015-09-23 | 0 | 1 | -1 |
2015-09-28 | 1 | 5 | -4 |
In this case, the first row of the table is assigned a value because there exists a row that comes right after that. But at the same time, in case of LEAD() function, the last row of the table or a partition to which the function is applied is always assigned the NULL value because there is no row afterwards.
FIRST_VALUE()
The FIRST_VALUE() function is not that commonly used but is also a rather interesting value window function in SQL. It does exactly what its name suggests - for all the rows, it assigns the first value of the table or the partition to which it is applied, according to some ordering that determines which row comes as a first one. Moreover, the variable from which the first value should be returned needs to be defined as the parameter of the function.
This function is not frequently appearing in interview questions, hence, to illustrate it with the example, let’s consider a question from Microsoft which uses FIRST_VALUE() and change it slightly.
Interview Question Date: March 2021
Write a query that returns the number of unique users per client per month
Link to the question: https://platform.stratascratch.com/coding/2024-unique-users-per-client-per-month
The original question asks to write a query that returns the number of unique users per client per month. But let’s assume that there is another task to calculate the difference of unique users of each client between the current month and the first month when the data is available.
The first part of this question can easily be solved with the following query:
SELECT client_id,
EXTRACT(month from time_id) as month,
count(DISTINCT user_id) as users_num
FROM fact_events
GROUP BY 1,2
client_id | month | users_num |
---|---|---|
desktop | 2 | 13 |
desktop | 3 | 16 |
desktop | 4 | 11 |
mobile | 2 | 9 |
mobile | 3 | 14 |
There are three months of data and two clients with 3 users each. To solve the second part of the question, the task will be to subtract the value in each month from the value for February of each account. For instance, for the client ‘desktop’, the value for February which is 13 will be subtracted from values for February (13), March (16) and April (11). Same for the other client. This can be computed using two queries: the inner one outputting the value for the first month and the outer query performing the calculation. But it can also be solved easier using the FIRST_VALUE() function. When the function is applied to the entire dataset, it looks as follows:
The second SQL code snapshot stays the same as in the article. But use the same code to show the output below it, which is not the case currently. Here’s the code to use in the widget:
SELECT client_id,
EXTRACT(month from time_id) as month,
count(DISTINCT user_id) as users_num,
FIRST_VALUE(count(DISTINCT user_id)) OVER(
ORDER BY client_id, EXTRACT(month from time_id))
FROM fact_events
GROUP BY 1,2
client_id | month | users_num | first_value |
---|---|---|---|
desktop | 2 | 13 | 13 |
desktop | 3 | 16 | 13 |
desktop | 4 | 11 | 13 |
mobile | 2 | 9 | 13 |
mobile | 3 | 14 | 13 |
mobile | 4 | 9 | 13 |
Given the defined ordering of the rows, the first value of the second column is 13, so the FIRST_VALUE() function assigns it to all the rows. Notice how the expression count(DISTINCT user_id) is also a parameter of the FIRST_ROW() function to indicate which variable should be used. However, the result above is not anywhere near the solution to this question because the results should be grouped by the client_id. This can be achieved using the PARTITION BY clause.
SELECT client_id,
EXTRACT(month from time_id) as month,
count(DISTINCT user_id) as users_num,
FIRST_VALUE(count(DISTINCT user_id)) OVER(
PARTITION BY client_id
ORDER BY EXTRACT(month from time_id))
FROM fact_events
GROUP BY 1,2
Now, the first value that is assigned to each row is not the first value of the entire dataset but of a partition to which a particular row belongs. This can be used to answer the second part of the interview question by simply subtracting the value in the fourth column from the value in the third column.
LAST_VALUE()
The LAST_VALUE() function is the exact opposite of the FIRST_VALUE() function and, as can be deduced from the name, returns the value from the last row of the dataset or the partition to which it is applied.
To give an example, if the second task in the interview question from Microsoft was not to calculate the difference between the current month and the first month when the data is available, but between the current month and the last month when the data is available, the query could include the LAST_ROW() function:
SELECT client_id,
EXTRACT(month from time_id) as month,
count(DISTINCT user_id) as users_num,
LAST_VALUE(count(DISTINCT user_id)) OVER(
PARTITION BY client_id)
FROM fact_events
GROUP BY 1,2
NTH_VALUE()
Finally, the NTH_VALUE() function is very similar to both the FIRST_VALUE() and the LAST_VALUE(). The difference is that while the other functions output the value of either the first or the last row of a window, the NTH_VALUE() allows the user to define which value from the order should be assigned to other rows. This function takes an additional parameter denoting which value should be returned.
Below is the example of this function based on the interview question from Microsoft and assuming that the difference is now calculated from the number of users in the second available month of data:
SELECT client_id,
EXTRACT(month from time_id) as month,
count(DISTINCT user_id) as users_num,
NTH_VALUE(count(DISTINCT user_id), 2) OVER(
PARTITION BY client_id)
FROM fact_events
GROUP BY 1,2
Advanced Windowing Syntax in SQL
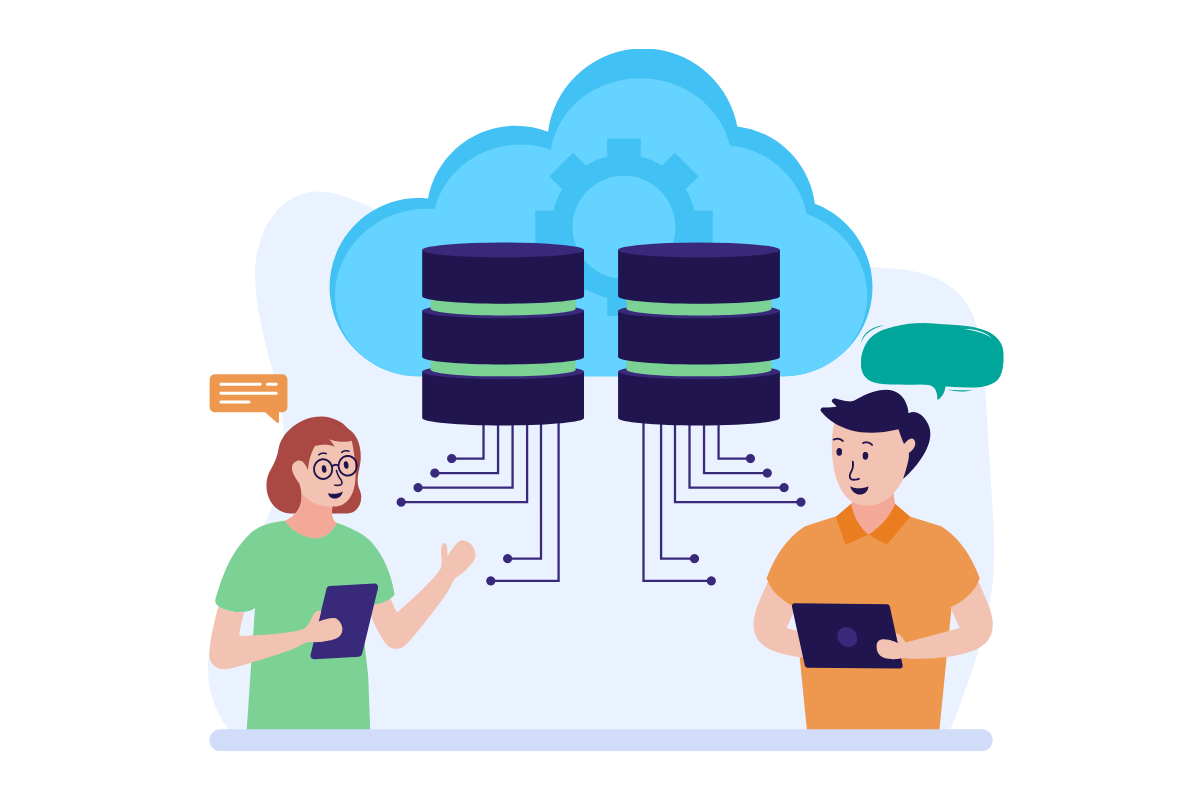
In most cases, SQL window functions use the ORDER BY clause to define the correct sorting to which a function should be applied, as well as the PARTITION BY clause when there is a need to aggregate the rows before applying the function. Both of these clauses have been covered repeatedly in this guide. However, the SQL window functions have even more potential and can be used in even more specific ways using the more advanced syntax, such as:
- Frame Specifications;
- EXCLUDE clause;
- FILTER clause;
- Window chaining.
Frame Specifications
The frame specifications determine which rows are taken as inputs by SQL window functions but while the ORDER BY and PARTITION BY clauses define the input based on the values of the dataset, the frame specifications allow defining the input from the perspective of the current row.
Before diving into the details, let’s consider the most common usage of the frame specifications in this interview question from Amazon on revenue.
Interview Question Date: December 2020
Find the 3-month rolling average of total revenue from purchases given a table with users, their purchase amount, and date purchased. Do not include returns which are represented by negative purchase values. Output the year-month (YYYY-MM) and 3-month rolling average of revenue, sorted from earliest month to latest month.
A 3-month rolling average is defined by calculating the average total revenue from all user purchases for the current month and previous two months. The first two months will not be a true 3-month rolling average since we are not given data from last year. Assume each month has at least one purchase.
Link to the question: https://platform.stratascratch.com/coding/10314-revenue-over-time
The task is to find the 3-month rolling average of total revenue from purchases given a table with users, their purchase amount, and date purchased. The question also specifies that a 3-month rolling average is defined by calculating the average total revenue from all user purchases for the current month and previous two months.
The first step is to compute the total revenue for each month based on the given table, which can be achieved using the following query:
SELECT to_char(created_at::date, 'YYYY-MM') AS MONTH,
sum(purchase_amt) AS monthly_revenue
FROM amazon_purchases
WHERE purchase_amt>0
GROUP BY to_char(created_at::date, 'YYYY-MM')
ORDER BY to_char(created_at::date, 'YYYY-MM')
month | monthly_revenue |
---|---|
2020-01 | 26292 |
2020-02 | 20695 |
2020-03 | 29620 |
2020-04 | 21933 |
2020-05 | 24700 |
Then, the results of this query can be used to calculate the rolling average. The most popular and efficient way to do it is by using the aggregate window function AVG() as follows.
We’ll also remove the monthly_revenue column because the question doesn’t require it.
SELECT t.month,
AVG(t.monthly_revenue) OVER(
ORDER BY t.month ROWS BETWEEN 2 PRECEDING
AND CURRENT ROW) AS avg_revenue
FROM
(SELECT to_char(created_at::date, 'YYYY-MM') AS MONTH,
sum(purchase_amt) AS monthly_revenue
FROM amazon_purchases
WHERE purchase_amt>0
GROUP BY to_char(created_at::date, 'YYYY-MM')
ORDER BY to_char(created_at::date, 'YYYY-MM')) t;
month | avg_revenue |
---|---|
2020-01 | 26292 |
2020-02 | 23493.5 |
2020-03 | 25535.667 |
2020-04 | 24082.667 |
2020-05 | 25417.667 |
Learn more about this interview question in our recent video:
In the query above, the construction within the OVER() clause of the window function ‘ROWS BETWEEN 2 PRECEDING AND CURRENT ROW’ is called a frame specification. This means that except for considering the ordering of the dataset, the SQL window function will compute the average only based on the 3 rows: the 2 preceding ones and the current row. This means that the input rows of the window function will be different for each row that is considered. For the 6th row, the input from which the average is calculated includes only the rows 4, 5 and 6. But for the 7th row, the input will be composed of rows 5, 6 and 7.
There are more keywords that can be used to define the frame. Firstly, the frame type may be ROWS as in the example, but it can also be GROUPS or RANGE. GROUPS refers to a set of rows that all have equivalent values for all terms of the window ORDER BY clause. RANGE is a more complicated and rarely used construction based on the ORDER BY clause that only has one term.
Then the frame boundary is defined after the BETWEEN keyword. It can use keywords such as CURRENT ROW, UNBOUNDED PRECEDING (everything before), UNBOUNDED FOLLOWING (everything after), PRECEDING and FOLLOWING. The last two keywords need to be used in combination with the number referring to how many rows, groups or ranges before or after should be considered.
EXCLUDE
The EXCLUDE clause is something that can be added to the frame specification to further change the input rows for a SQL window function. The default value is EXCLUDE NO OTHERS and does not impact the query in any way. The other possibilities are EXCLUDE CURRENT ROW, EXCLUDE GROUP or EXCLUDE TIES. These exclude from the frame respectively the current row, the whole group based on the current row, or all rows with the same value as the current row, except the current row.
To give an example, let’s assume that the rolling average in the interview question from Amazon is calculated by taking the average from the previous and following month. Namely, the value for April 2020 would be calculated by taking the mean from the revenues in March 2020 and May 2020. This can be solved with the following query:
SELECT t.month,
monthly_revenue,
AVG(t.monthly_revenue) OVER(
ORDER BY t.month ROWS BETWEEN 1 PRECEDING
AND 1 FOLLOWING EXCLUDE CURRENT ROW)
AS avg_revenue
FROM
(SELECT to_char(created_at::date, 'YYYY-MM') AS MONTH,
sum(purchase_amt) AS monthly_revenue
FROM amazon_purchases
WHERE purchase_amt>0
GROUP BY to_char(created_at::date, 'YYYY-MM')
ORDER BY to_char(created_at::date, 'YYYY-MM')) t
month | monthly_revenue | avg_revenue |
---|---|---|
2020-01 | 26292 | 20695 |
2020-02 | 20695 | 27956 |
2020-03 | 29620 | 21314 |
2020-04 | 21933 | 27160 |
2020-05 | 24700 | 24810 |
The expression within the OVER() clause of the window functions now became ’ROWS BETWEEN 1 PRECEDING AND 1 FOLLOWING EXCLUDE CURRENT ROW’ to reflect the new definition of the rolling average. See how the value for April 2020 is equal to the mean from the revenues in March 2020 and May 2020: (29620+24700)/2 = 27160.
FILTER
Another possible clause that can be used in combination with the SQL window functions is the FILTER clause. It allows defining additional conditions to exclude certain rows from the input of a function based on their values. The FILTER clause is placed between the window function and the OVER keyword and it contains a WHERE clause as its argument.
To give an example, let’s assume that the rolling average in the question from Amazon is still calculated based on the revenue from the previous and following month but only for months where the revenue has been higher than 25000. This condition can be added by using the FILTER clause like that:
SELECT t.month,
monthly_revenue,
AVG(t.monthly_revenue)
FILTER(WHERE monthly_revenue > 25000)
OVER(ORDER BY t.month ROWS BETWEEN 1 PRECEDING AND 1
FOLLOWING EXCLUDE CURRENT ROW) AS avg_revenue
FROM
(SELECT to_char(created_at::date, 'YYYY-MM') AS MONTH,
sum(purchase_amt) AS monthly_revenue
FROM amazon_purchases
WHERE purchase_amt>0
GROUP BY to_char(created_at::date, 'YYYY-MM')
ORDER BY to_char(created_at::date, 'YYYY-MM')) t
month | monthly_revenue | avg_revenue |
---|---|---|
2020-01 | 26292 | |
2020-02 | 20695 | 27956 |
2020-03 | 29620 | |
2020-04 | 21933 | 29620 |
2020-05 | 24700 | 27687 |
The query works properly because for some months the values are missing and for some the averages are only based on one month. For example, the rolling average for March 2020 cannot be computed because the revenues in both February and April are less than 25000.
Window Chaining
Window chaining is a technique that allows defining the window that can be used by window functions. Such a window needs to only be defined once but then can be reused by multiple SQL window functions. The concept is similar to the Common Table Expressions that allow defining and reusing a query. Similarly, the window can be declared separately, given an alias and reused several times by only inserting the alias in the right places.
As an example, let’s consider this interview question from Amazon with window chaining.
Interview Question Date: December 2020
Given a table of purchases by date, calculate the month-over-month percentage change in revenue. The output should include the year-month date (YYYY-MM) and percentage change, rounded to the 2nd decimal point, and sorted from the beginning of the year to the end of the year. The percentage change column will be populated from the 2nd month forward and can be calculated as ((this month's revenue - last month's revenue) / last month's revenue)*100.
Link to the question: https://platform.stratascratch.com/coding/10319-monthly-percentage-difference
The task is to, given a table of purchases by date, calculate the month-over-month percentage change in revenue. It is also said that the percentage change column will be populated from the 2nd month forward and can be calculated as ((this month's revenue - last month's revenue) / last month's revenue)*100.
This question can be solved with the following query containing two very long and unclear LAG() window functions combined in a single expression:
SELECT to_char(created_at::date, 'YYYY-MM') AS year_month,
round(((sum(value) - lag(sum(value), 1) OVER (ORDER BY to_char
(created_at::date,
'YYYY-MM'))) / (lag(sum(value), 1) OVER (ORDER BY to_char
(created_at::date, 'YYYY-MM')))) * 100, 2) AS revenue_diff_pct
FROM sf_transactions
GROUP BY year_month
ORDER BY year_month ASC
year_month | revenue_diff_pct |
---|---|
2019-01 | |
2019-02 | -28.56 |
2019-03 | 23.35 |
2019-04 | -13.84 |
2019-05 | 13.49 |
Note how the inside of the OVER clause is the same for both functions: ORDER BY to_char(created_at::date, 'YYYY-MM'). This messy query can be made clearer and easier to read by using the window chaining. The window can be defined as follows and given an alias ‘w’: WINDOW w AS (ORDER BY to_char(created_at::date, 'YYYY-MM')). Then, any time a window function should be applied to this window, the OVER clause will simply contain the alias ‘w’. The query from above then becomes:
SELECT to_char(created_at::date, 'YYYY-MM') AS year_month,
round(((sum(value) - lag(sum(value), 1) OVER w) /
(lag(sum(value), 1) OVER w)) * 100, 2) AS revenue_diff_pct
FROM sf_transactions
GROUP BY year_month
WINDOW w AS (ORDER BY to_char(created_at::date, 'YYYY-MM'))
ORDER BY year_month ASC
To practice more SQL questions, you can check out this ultimate guide to SQL interview questions. We have also covered topics like SQL JOIN Interview Questions and SQL Scenario Based Interview Questions.
Conclusion
This ultimate guide has covered all aspects of the SQL window functions, starting from listing all the functions and their categories, explaining the usage of the common ORDER BY and PARTITION BY keywords, as well as outlining the more advanced syntax. As shown using the numerous examples, the notions of the SQL window functions appear frequently in interview questions asked by top tech companies. The SQL window functions are also often used in the everyday work of data scientists and data analysts.
The SQL window functions are very powerful and efficient and their syntax, though rich and slightly different from the standard SQL, is quite logical. However, using SQL window functions comfortably requires a lot of practice, for example, solving the interview questions from top companies.
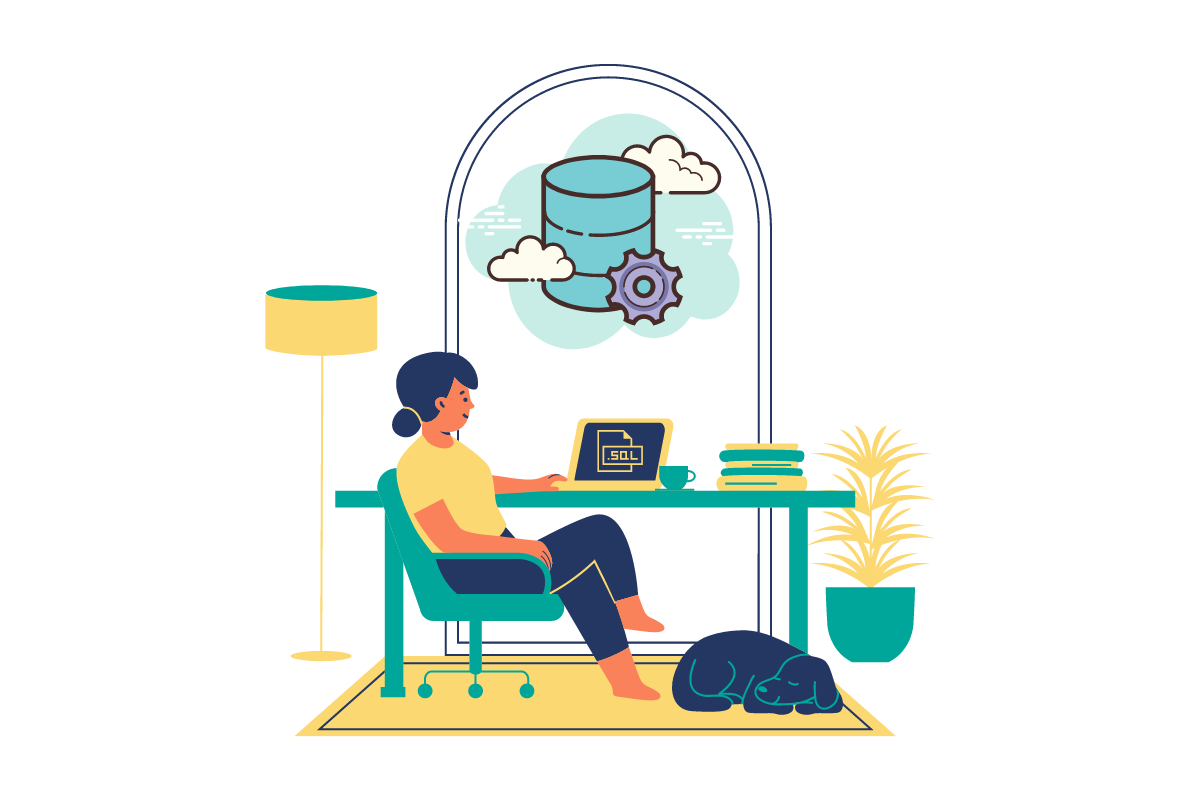