Python Libraries for Data Clean-Up
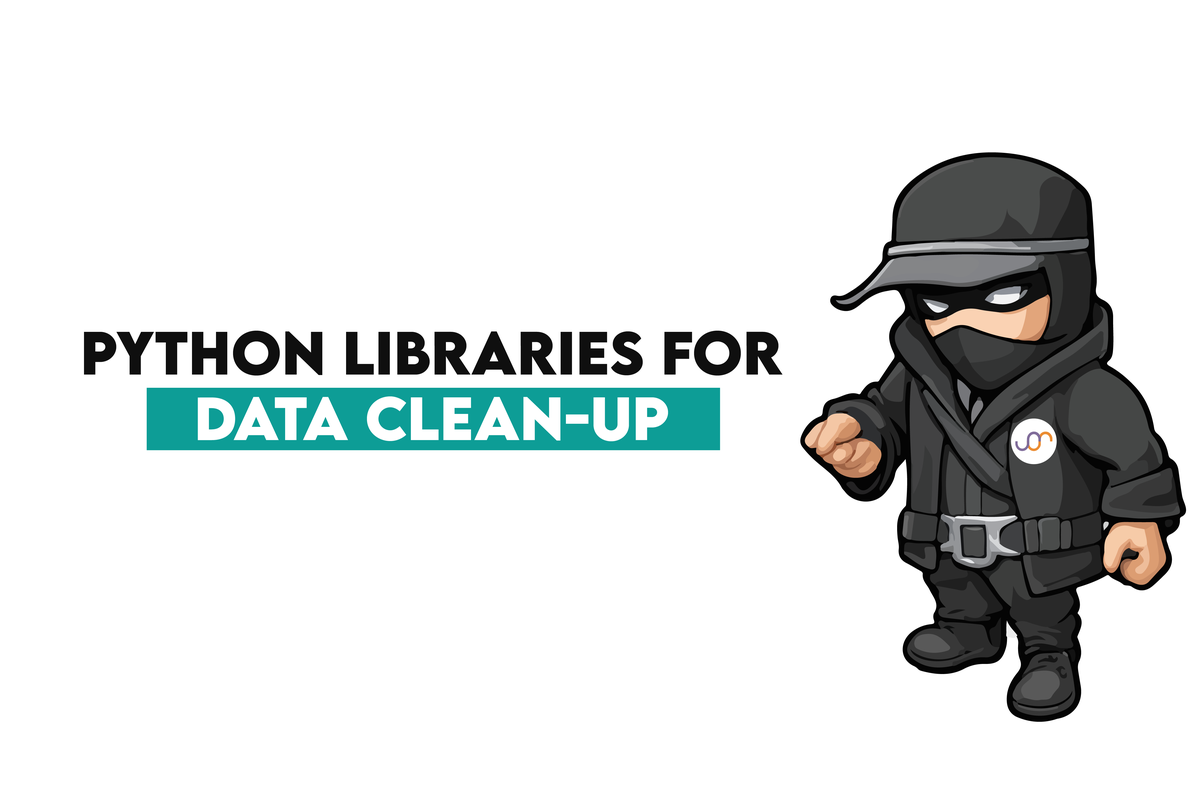
- Written by:
Nathan Rosidi
Data cleaning is an integral part of every data science project. This tedious but essential task can be much easier if you start using these Python libraries.
In today’s article, we'll examine a crucial part of any data science project: data cleanup.
Before you do anything with it, you need to make sure your data is squeaky clean. Let’s explore why this is so important, and then we’ll talk about some of the best Python libraries out there to help you tidy up your data.
Why is Data Cleaning Important?
Raw data is often messy and full of missing values, duplicates, outliers, and inconsistencies. If you don't clean your data, it can lead to inaccurate analyses and poor decision-making. Clean data means reliable outputs of your data science project.
Python Libraries to Use for Data Cleaning
We’ll now introduce you to these eight Python libraries great for data cleaning.

We’ll show you several essential features of each library, together with a code snapshot showing practical use.
1. Pandas
Pandas, a powerful Python library for data manipulation and analysis, is a crowd favorite. It's great for handling tabular data with its DataFrame structure. Generally speaking, pandas is versatile and integrates seamlessly with other data science libraries like NumPy and SciPy, making it a staple for any data scientist.
You can use its features for many data cleaning operations, including these.

1. Handling Missing Values: Use df.dropna() to remove missing values.
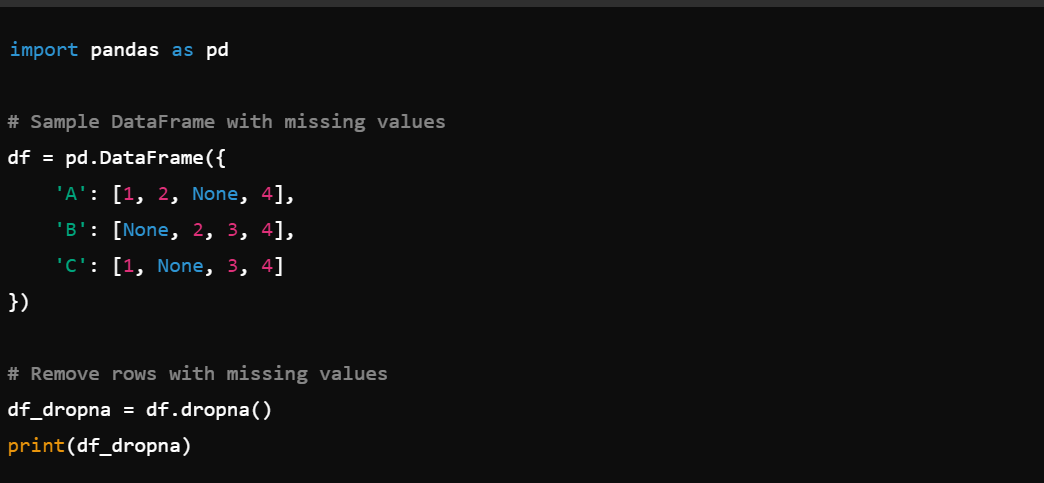
Also, you can use df.fillna() to replace missing values.

2. Removing Duplicates: The df.drop_duplicates() function helps in removing duplicate rows.
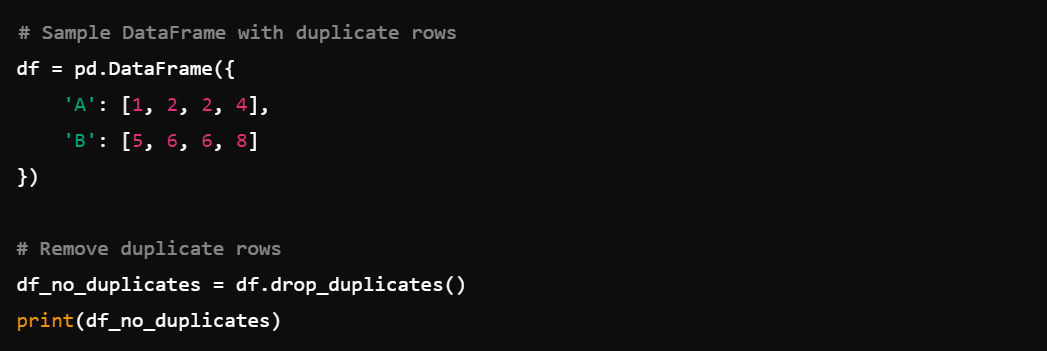
3. Data Transformation: Pandas makes it easy to apply functions to your data with df.apply().
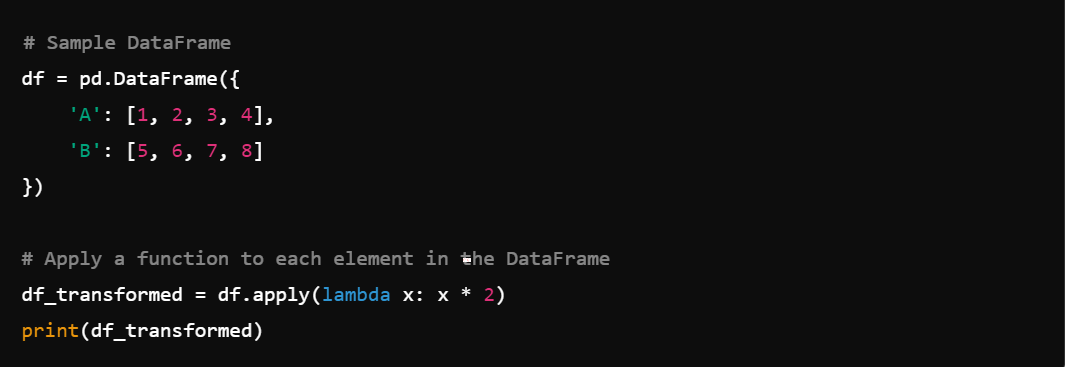
4. Filtering Data: To filter data, you can use df.query()
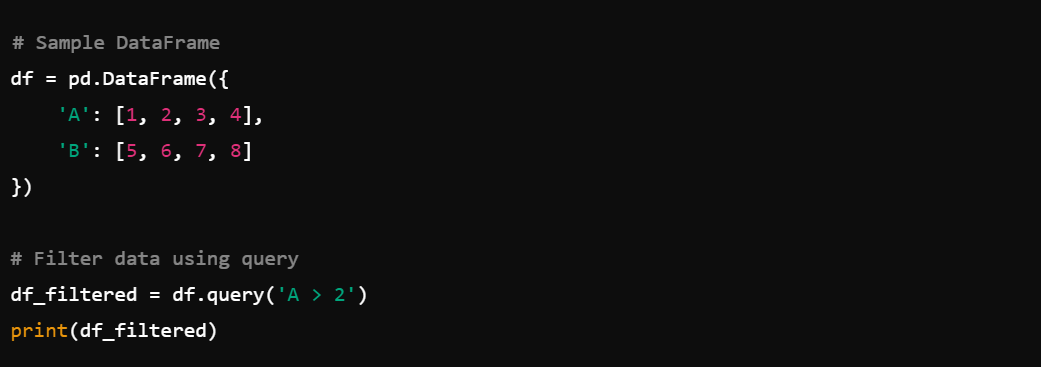
or df[df['column'] > value].

5. Merging and Joining: Combine DataFrames with df.merge()
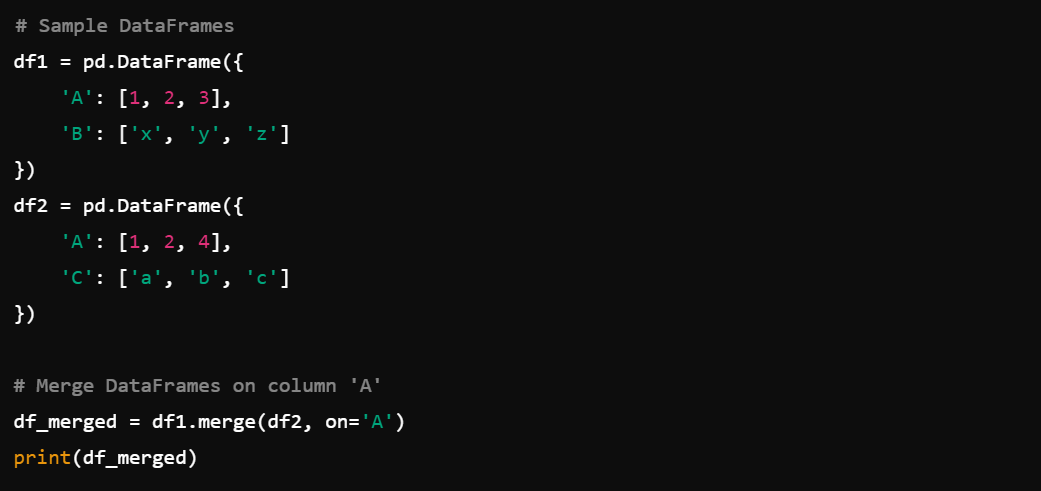
and df.join().
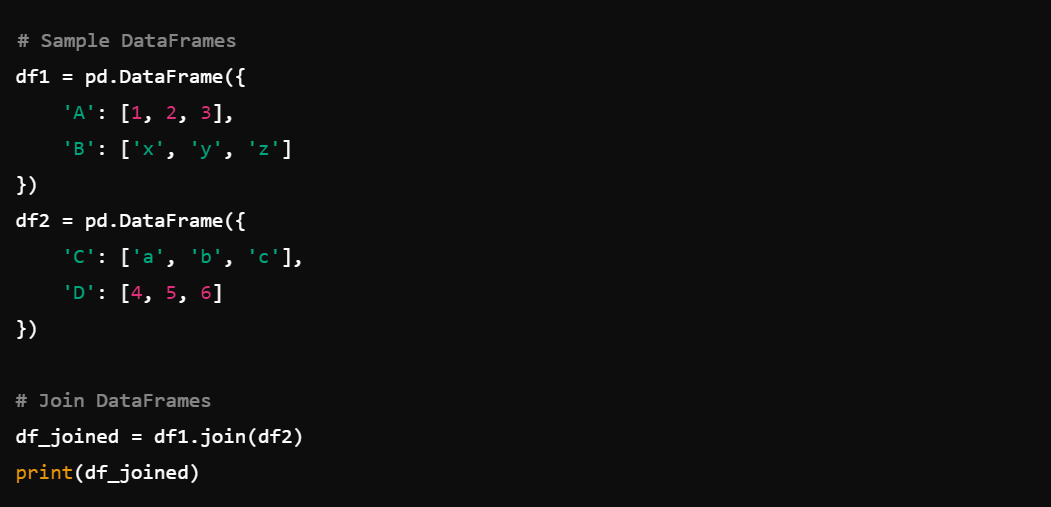
6. Grouping and Aggregating: For grouping and aggregating data, use df.groupby() followed by aggregation functions like sum(), mean(), count().
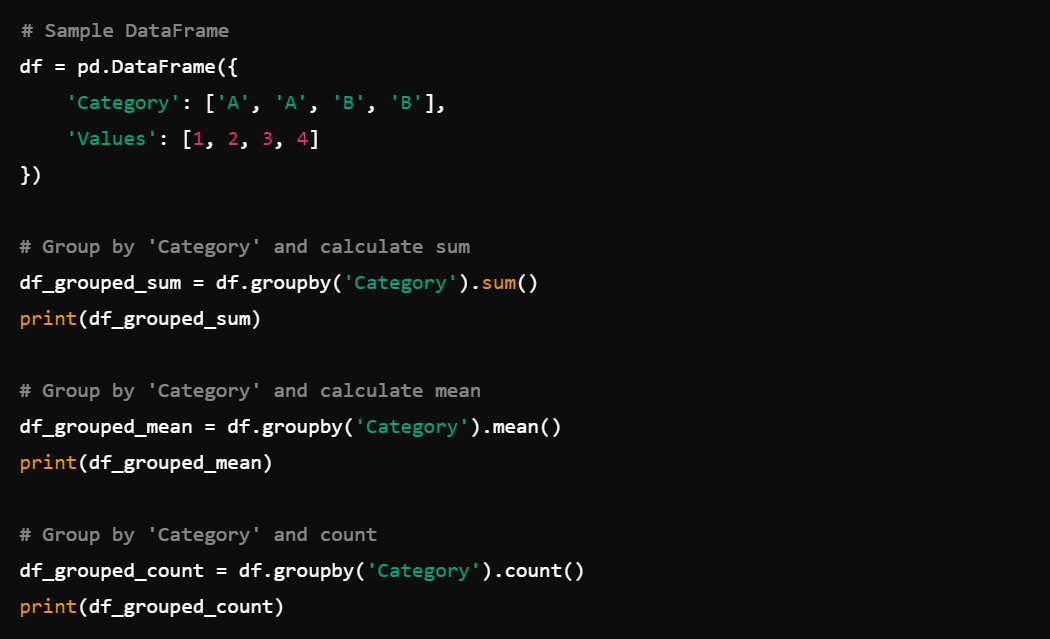
7. Pivot Tables: Create pivot tables with pd.pivot_table().
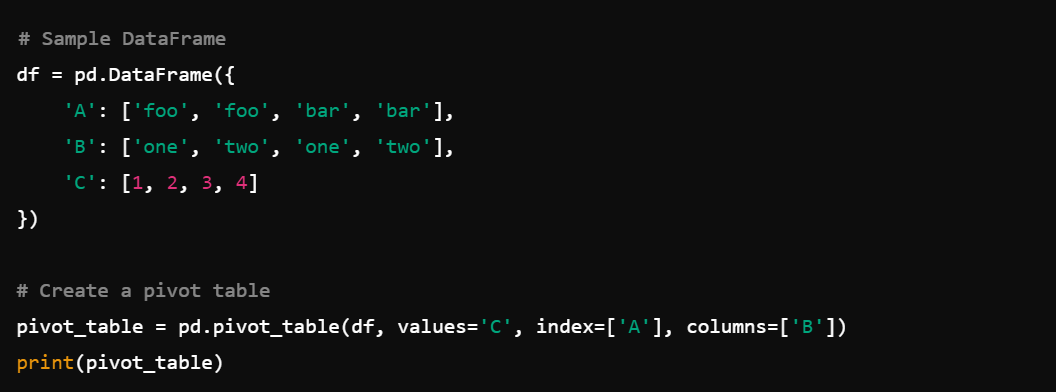
8. Datetime Conversion: Convert strings to datetime with pd.to_datetime().
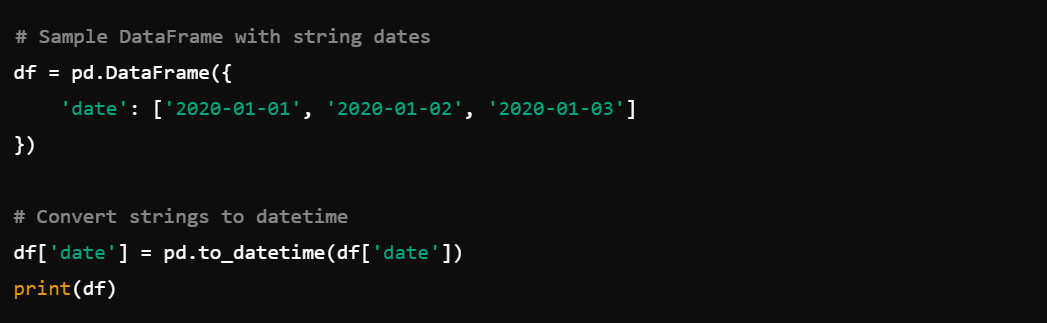
9. String Operations: Perform string operations like df['column'].str.contains(), e.g., to check if column A contains the string 'ba'.
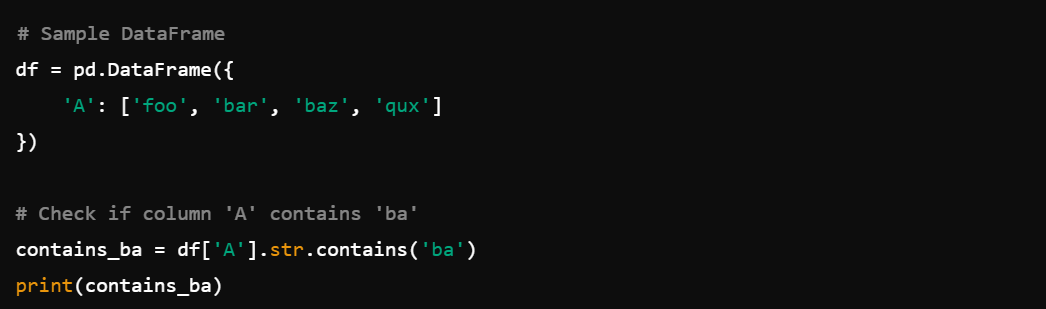
or df['column'].str.replace(), e.g., to replace 'foo' with 'boo' in column A.

10. Sorting Data: Sort data with df.sort_values(), like the example below sorts by column A.
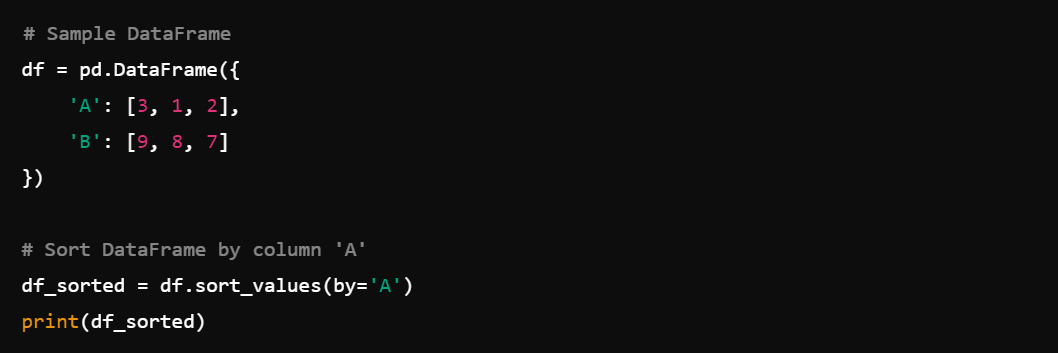
11. Reshaping Data: Reshape data with melt()
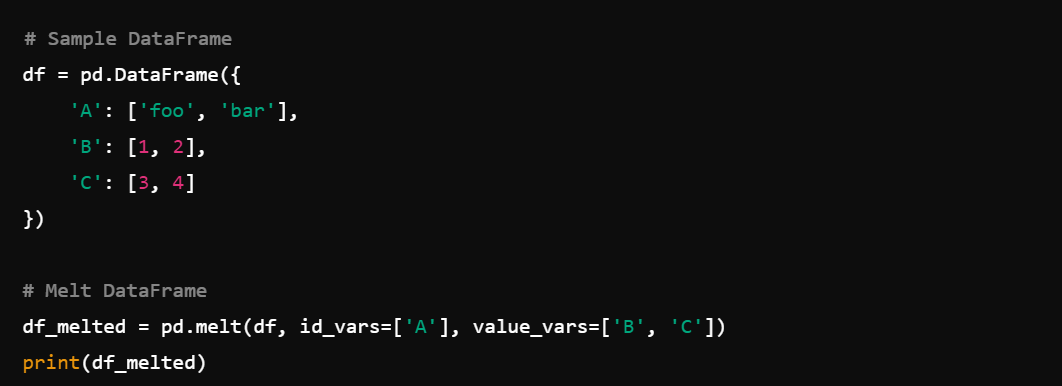
and pivot().
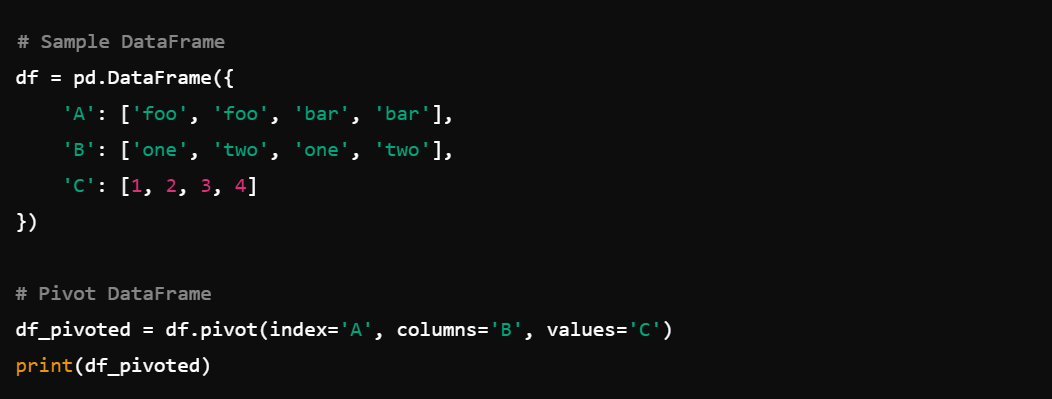
12. Handling Categorical Data: Use pd.Categorical() for handling categorical data, e.g., to convert column A to categorical.
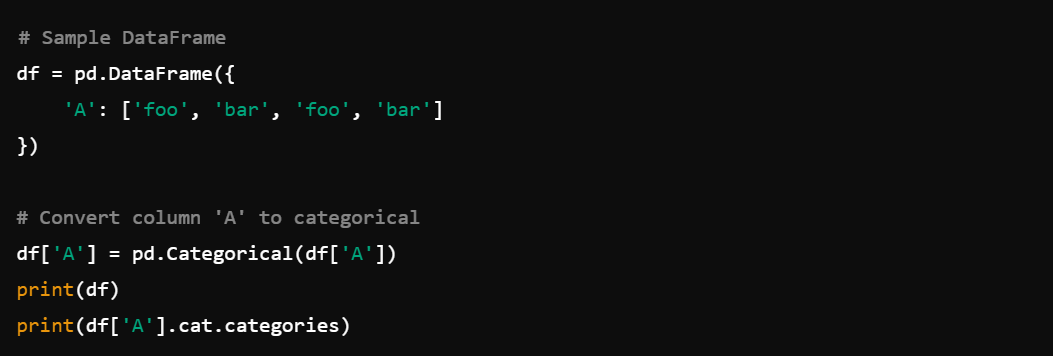
13. Rolling Statistics: Compute rolling statistics like df.rolling(window=3).mean(), which calculates the rolling average with a window of 3.
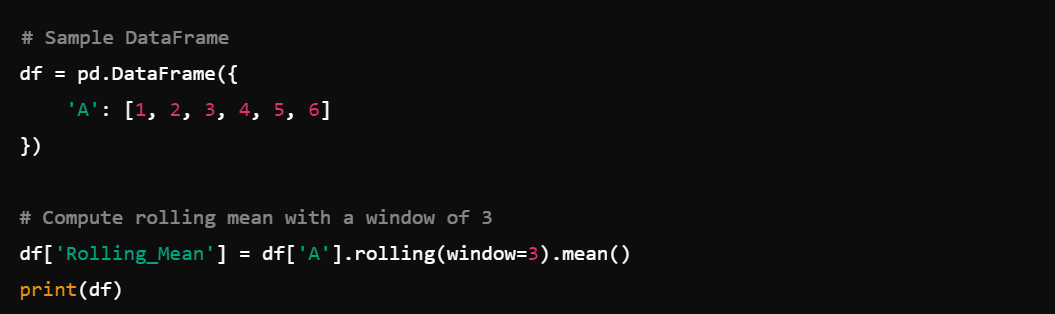
2. NumPy
NumPy is another essential library in the Python ecosystem, primarily known for numerical computations. However, NumPy also offers several functionalities for data cleaning.

1. Handling Missing Data: Use np.nan and np.isnan() to identify and handle missing values.
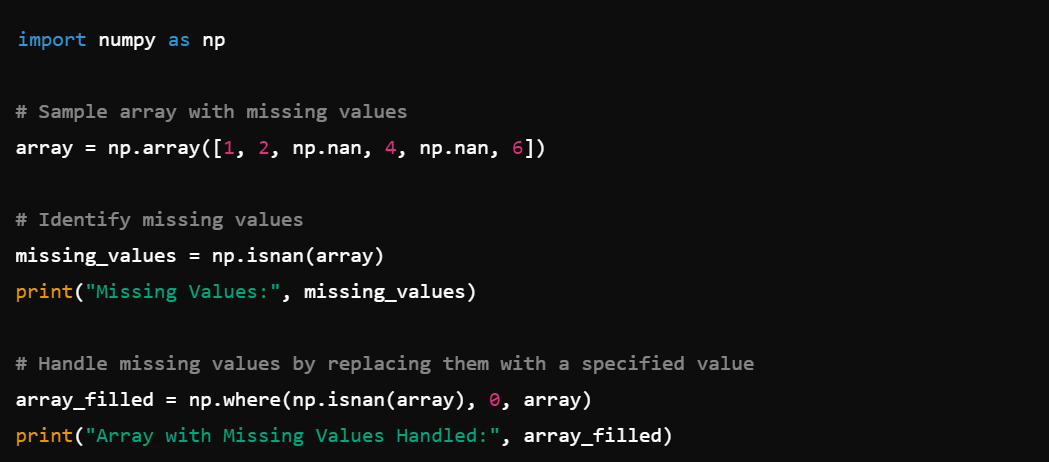
2. Array Operations: Efficiently perform element-wise operations such as np.add(), np.subtract(), np.multiply(), and np.divide().
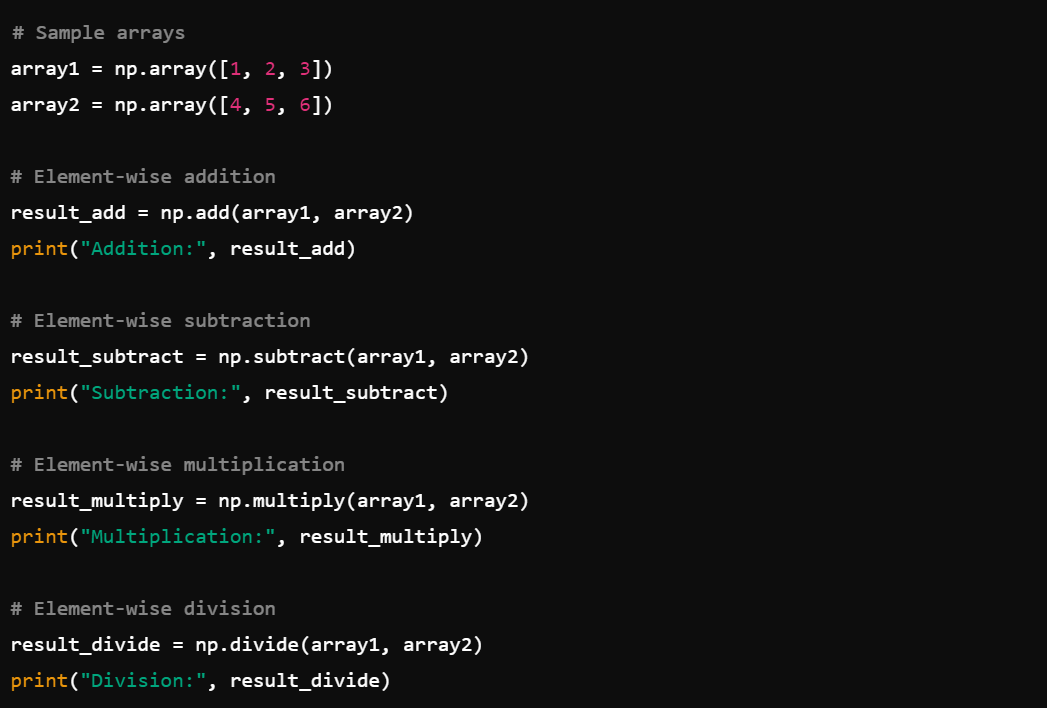
3. Sorting and Searching: Use np.sort()
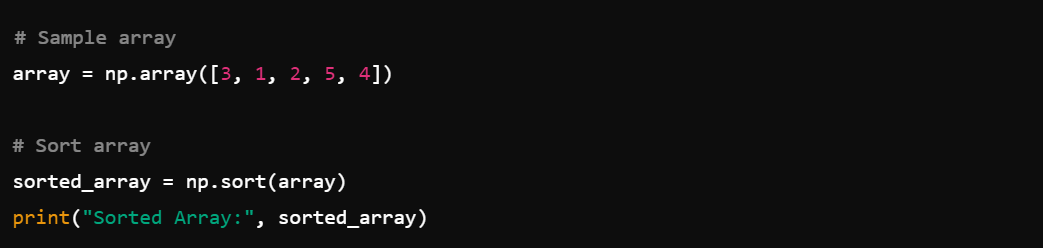
and np.searchsorted() for sorting and searching operations.
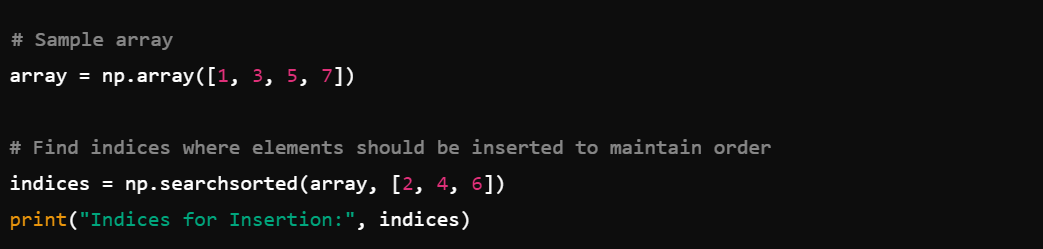
4. Boolean Indexing: Use boolean arrays for filtering data, e.g., array[array > value]. In the example below, it is used to filter values greater than three.
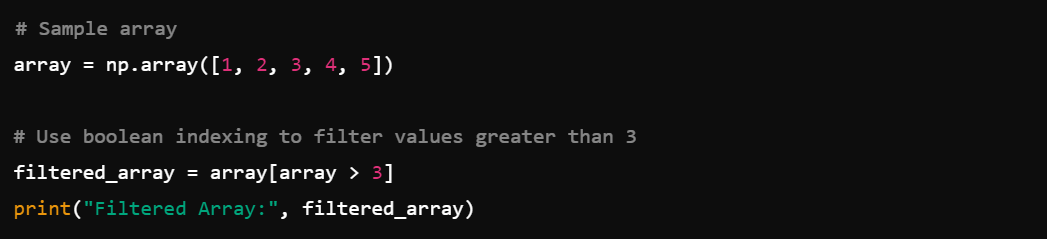
5. Statistical Functions: Calculate mean, median, standard deviation, etc., using functions like np.mean(), np.median(), np.std().
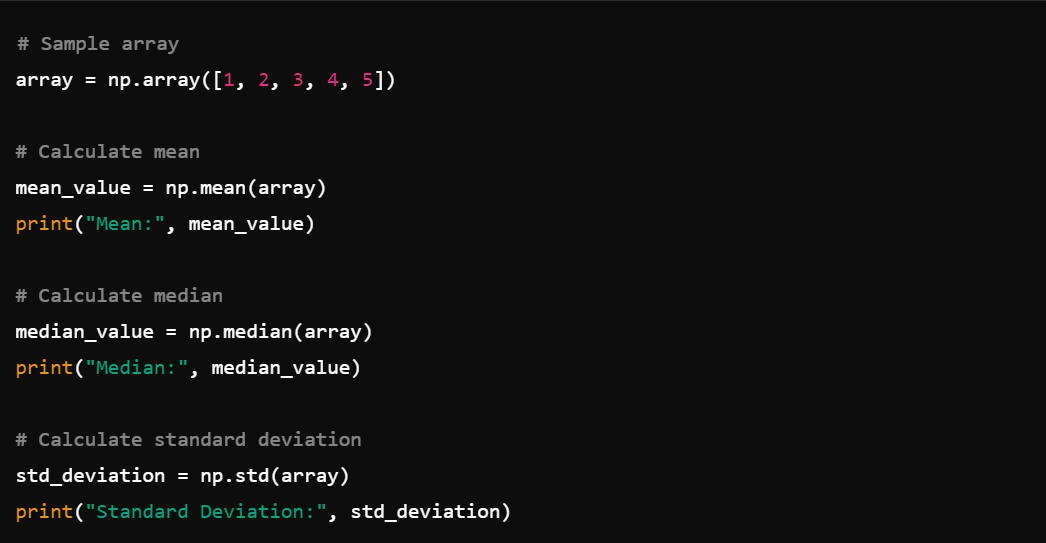
6. Data Type Conversion: Convert data types with astype(), e.g., array.astype(np.float64).
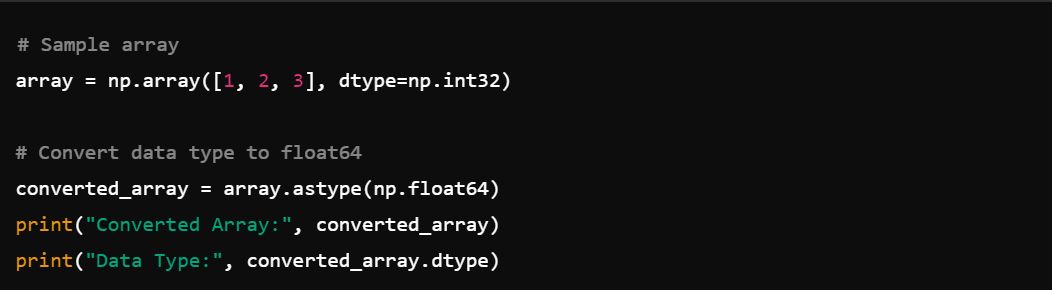
7. Broadcasting: Also, you can perform operations on arrays of different shapes.
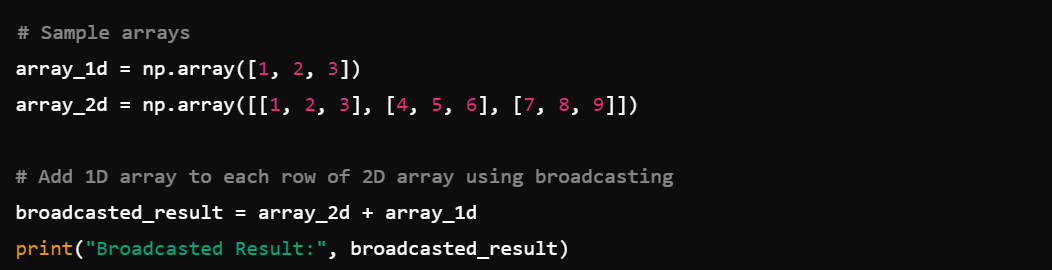
8. Matrix Operations: Use np.dot(), np.matmul(), np.linalg.inv() for matrix operations.
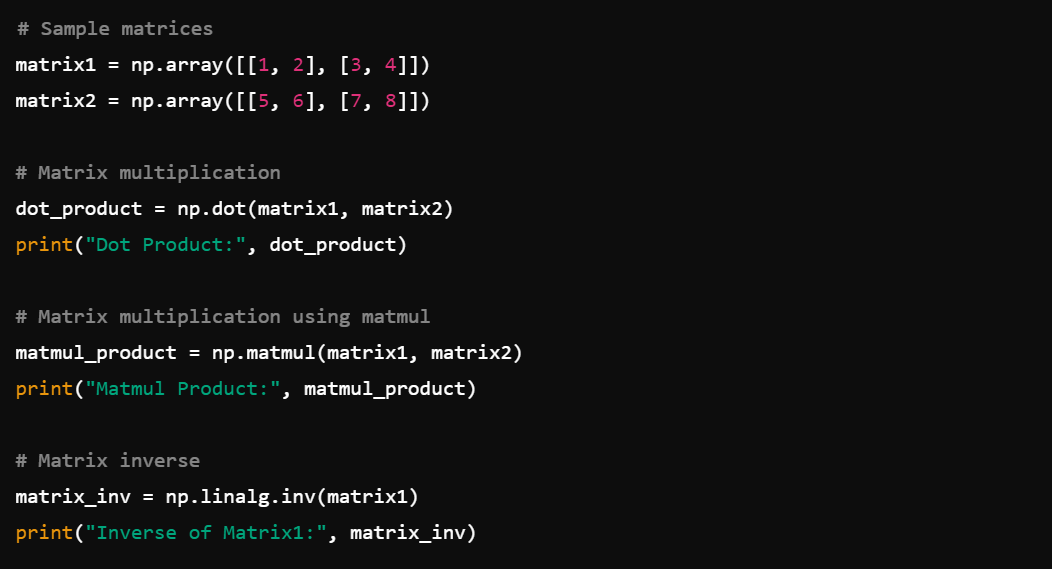
9. Random Sampling: Generate random samples with np.random.rand(), np.random.randint().
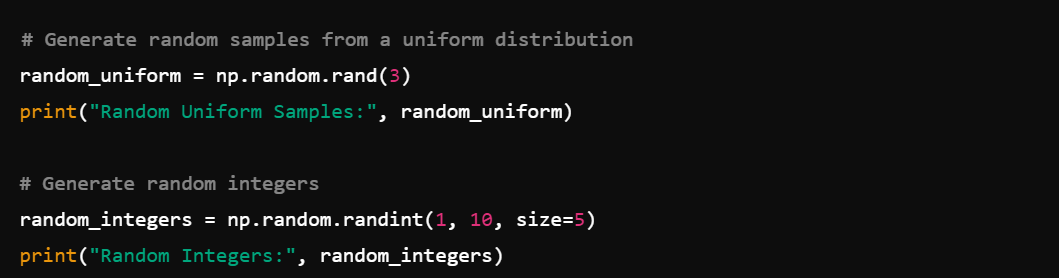
10. Array Reshaping: Reshape arrays with reshape(), ravel(), flatten().
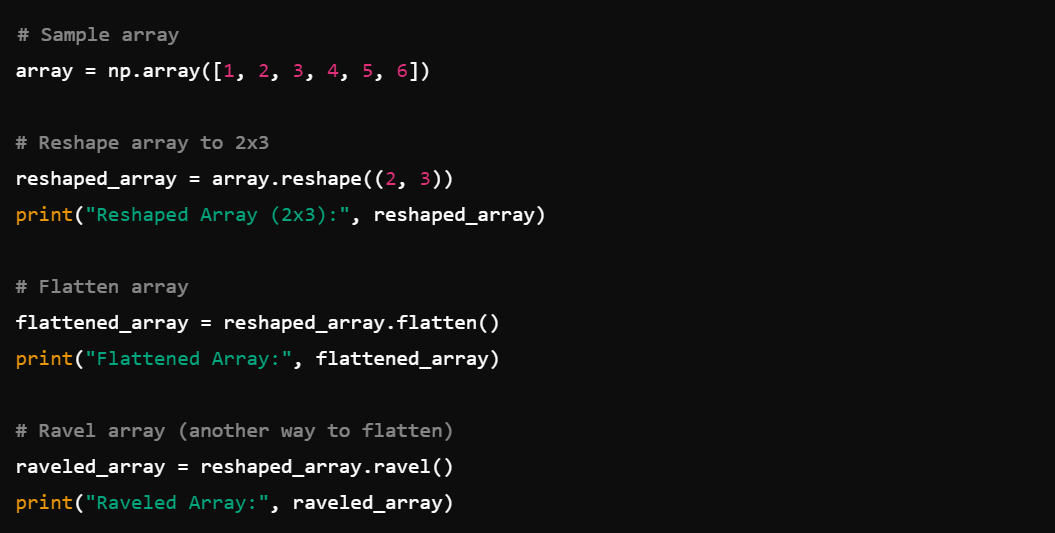
11. Clipping Values: Limit the values in an array with np.clip().
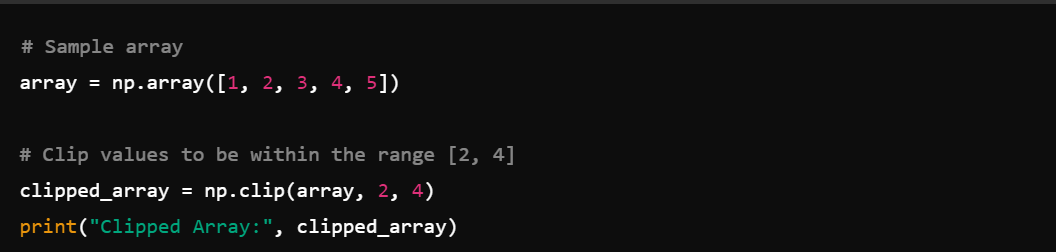
12. Unique Values: Find unique elements in an array with np.unique().
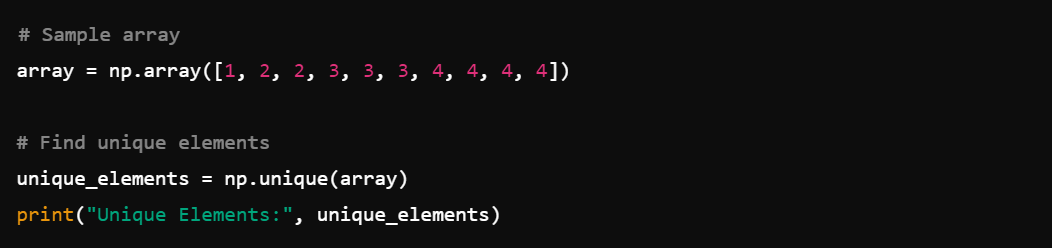
With all these functions, NumPy is essential for any data science project involving numerical data.
3. SciPy
Next, we have SciPy, a Python library building on NumPy. SciPy extends NumPy's capabilities and offers additional functionalities for data cleaning.

SciPy is a powerful tool for more advanced data cleaning tasks and integrates seamlessly with NumPy and pandas.
1. Interpolation: Use scipy.interpolate to fill in missing values, e.g., interpolate.interp1d().
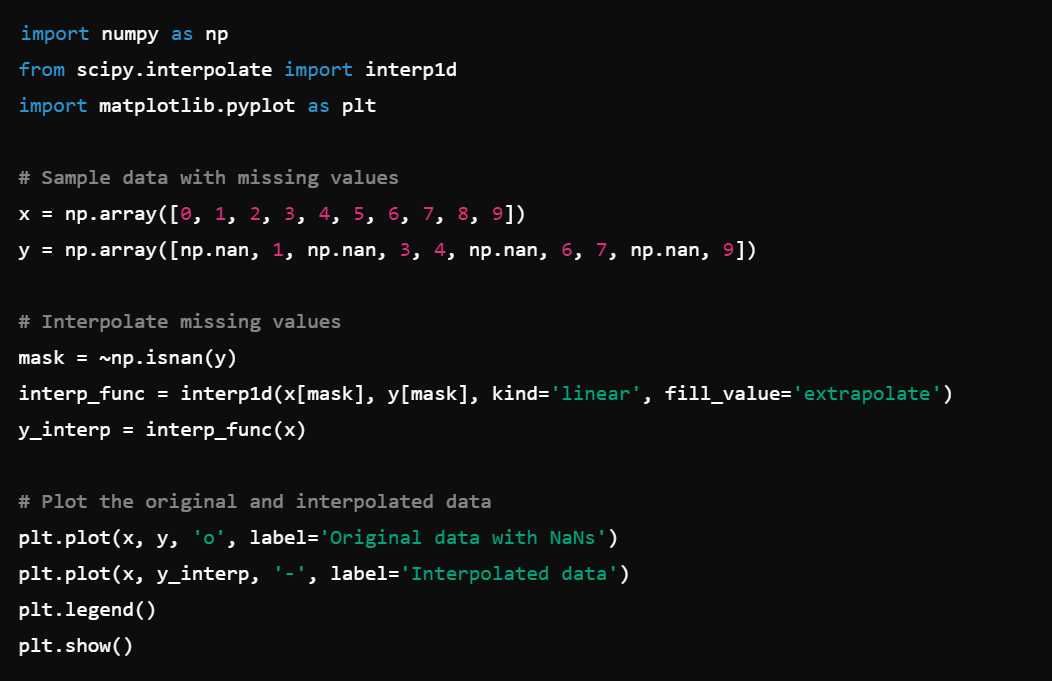
2. Signal Processing: Apply filters and smooth data using scipy.signal, e.g., signal.savgol_filter().
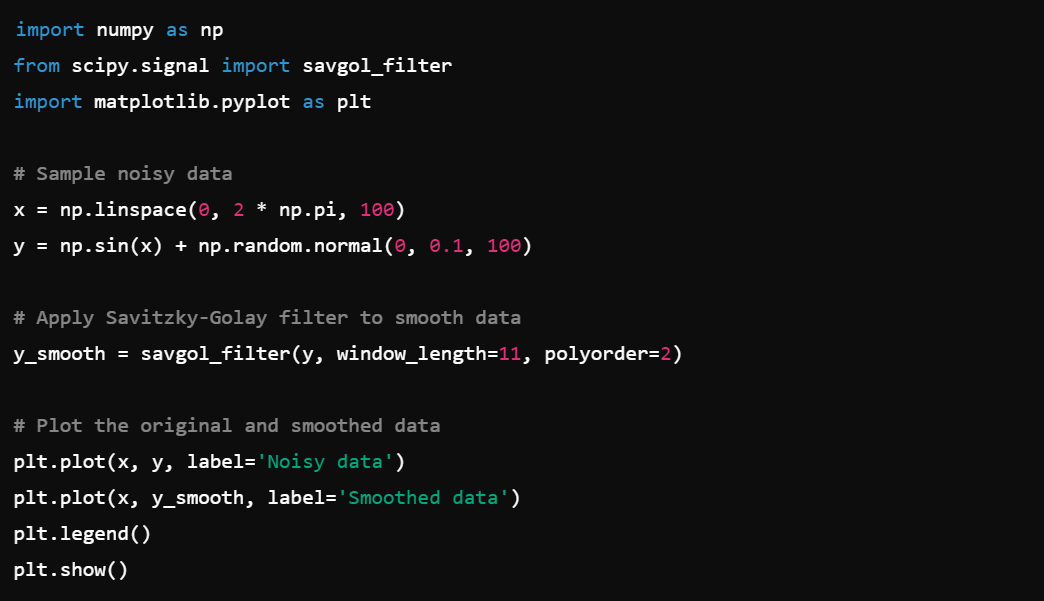
3. Statistics: Use scipy.stats to handle outliers and perform statistical data cleaning, e.g., stats.zscore().
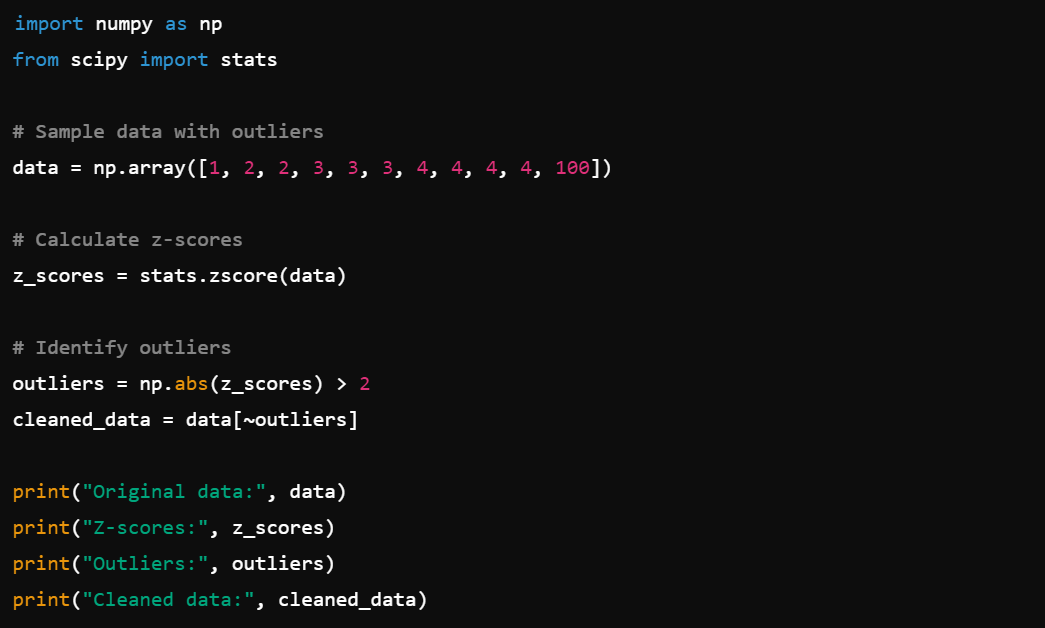
4. Optimization: Optimize data cleaning processes with scipy.optimize, e.g., optimize.minimize().
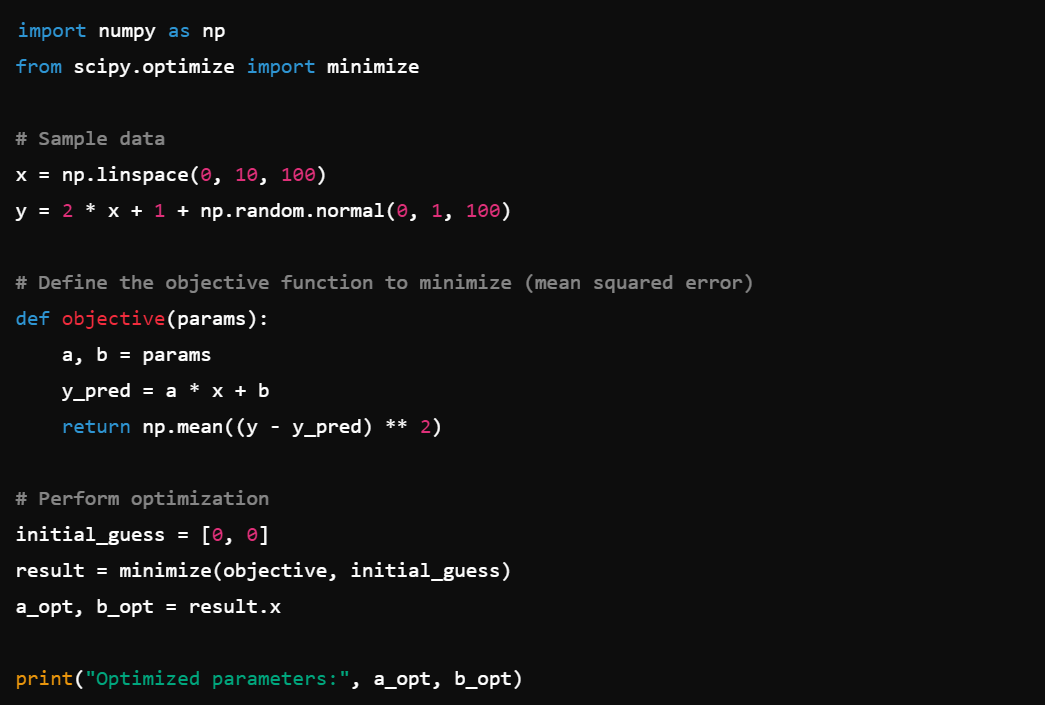
5. Sparse Matrices: Efficiently handle large, sparse datasets with scipy.sparse, e.g., sparse.csr_matrix().
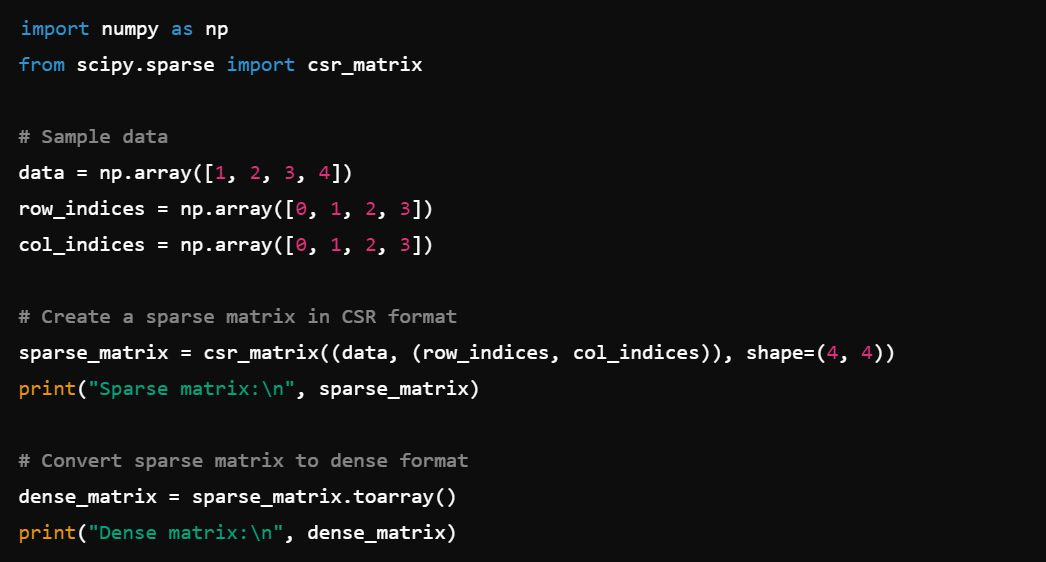
6. Linear Algebra: Perform advanced linear algebra operations with scipy.linalg, e.g., linalg.eig().
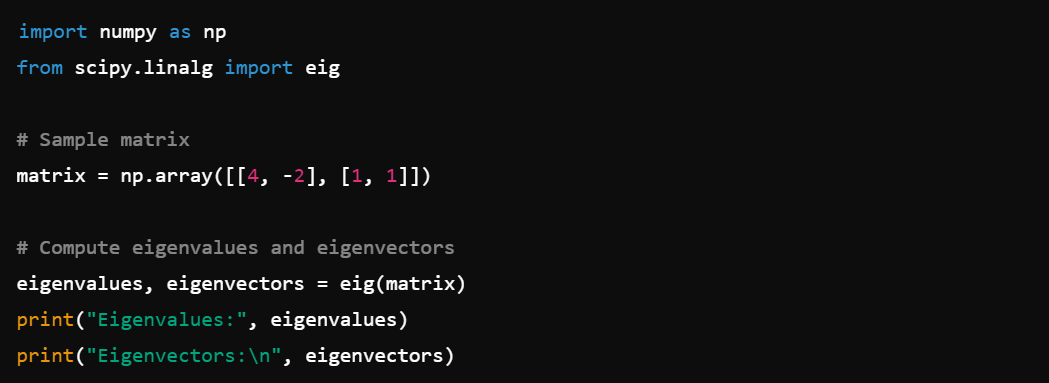
7. Clustering: Cluster data with scipy.cluster, e.g., cluster.hierarchy.
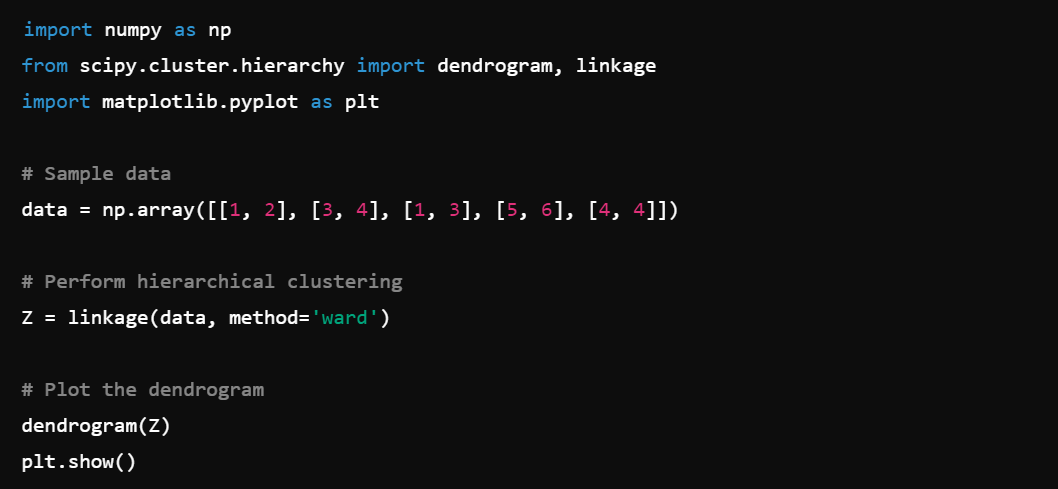
8. Integration: Perform numerical integration with scipy.integrate, e.g., integrate.quad().
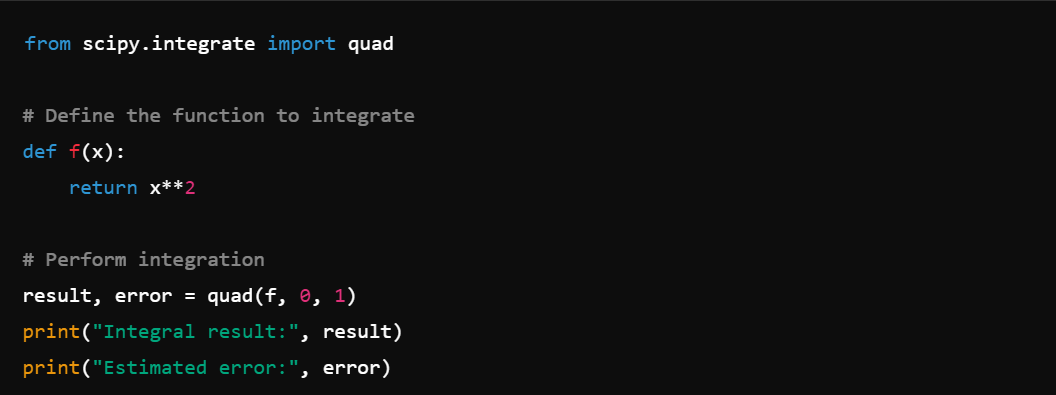
9. Special Functions: Use special mathematical functions from scipy.special, e.g., special.gamma().
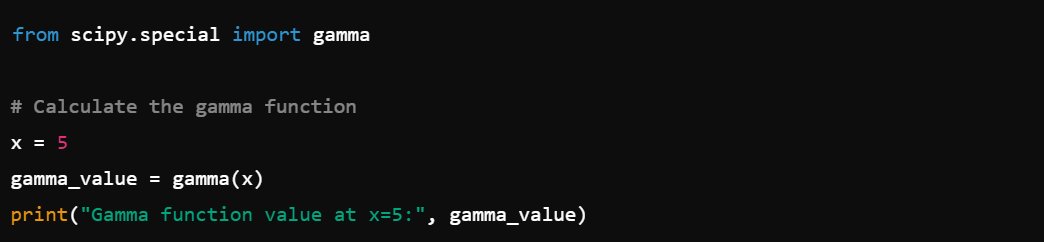
10. Image Processing: Use scipy.ndimage for multidimensional image processing, e.g., ndimage.gaussian_filter().
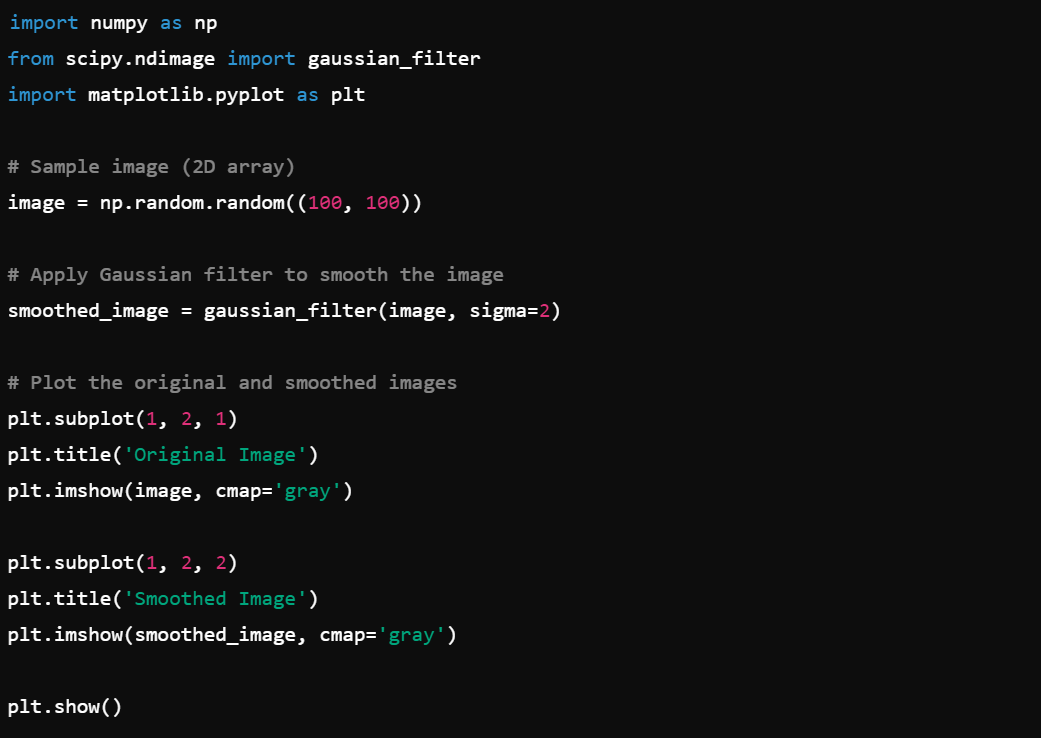
11. Root Finding: Solve equations with scipy.optimize.root().
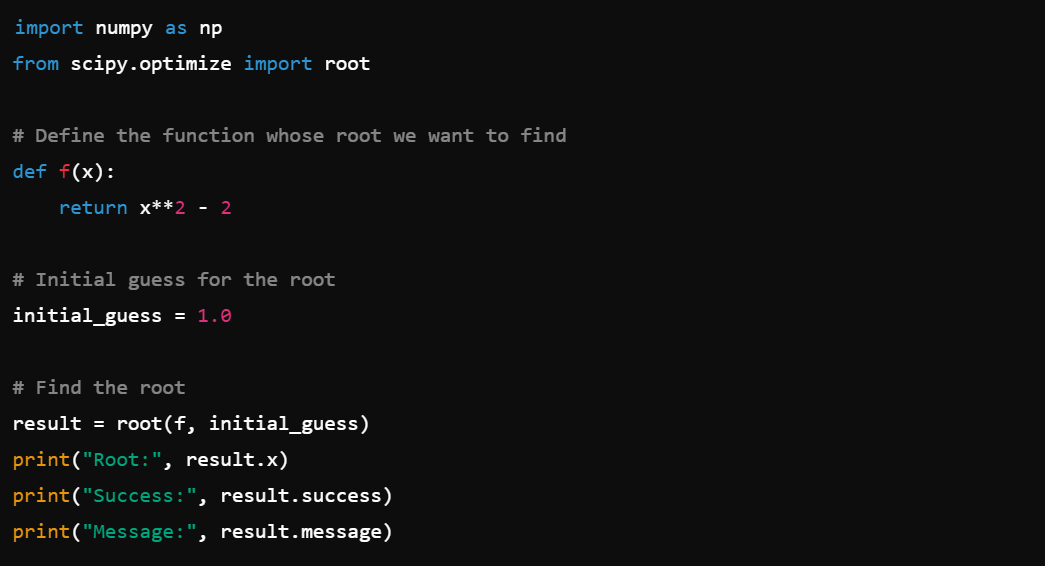
4. Pyjanitor
This library builds on top of pandas to provide additional data cleaning functionalities.

Pyjanitor is a great addition to your toolkit if you’re already familiar with pandas and want to streamline your data cleaning process.
1. Chaining Methods: Clean data using method chaining for readability, e.g., df.clean_names().remove_empty().
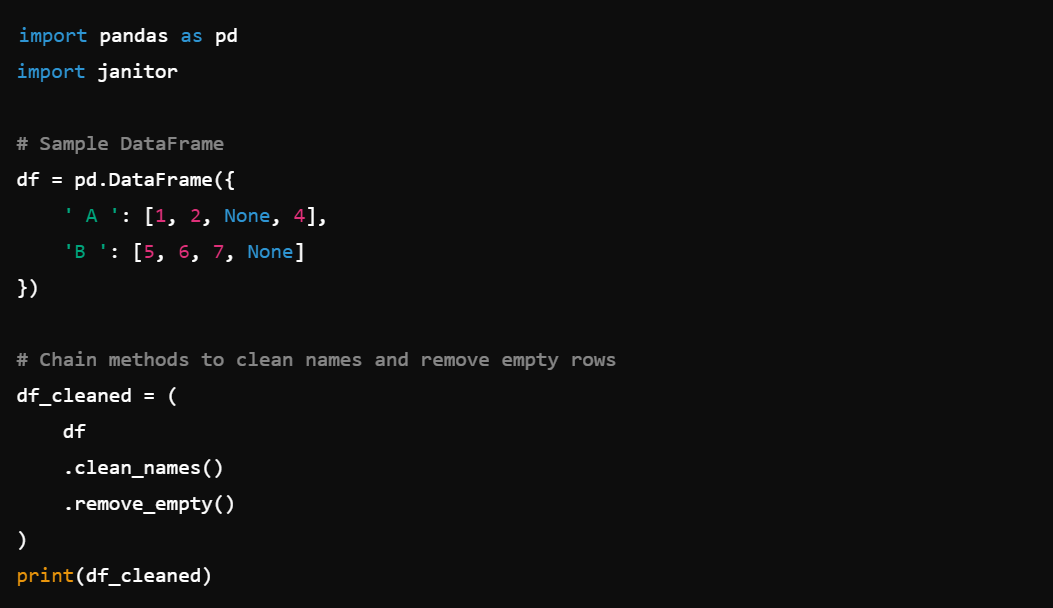
2. Cleaning Column Names: Easily clean column names with df.clean_names().
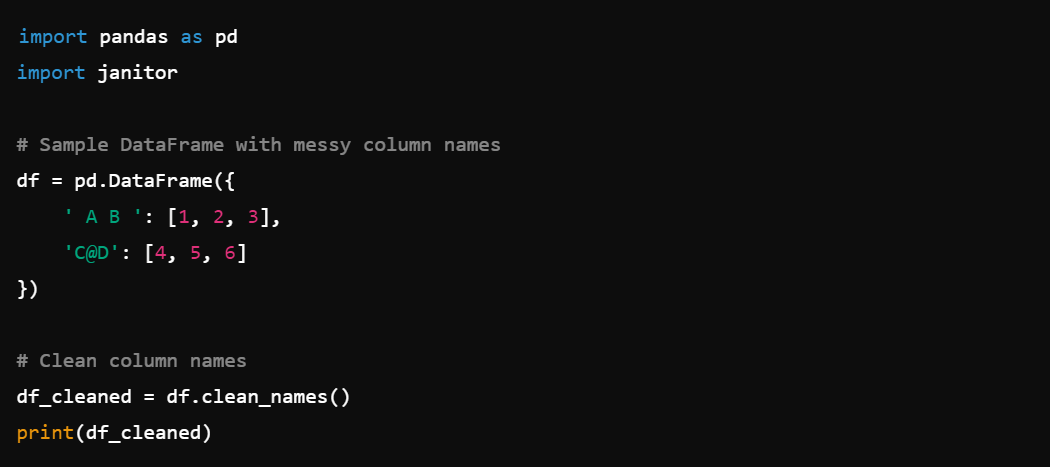
3. Removing Outliers: Use df.remove_outliers() to handle outliers effectively.
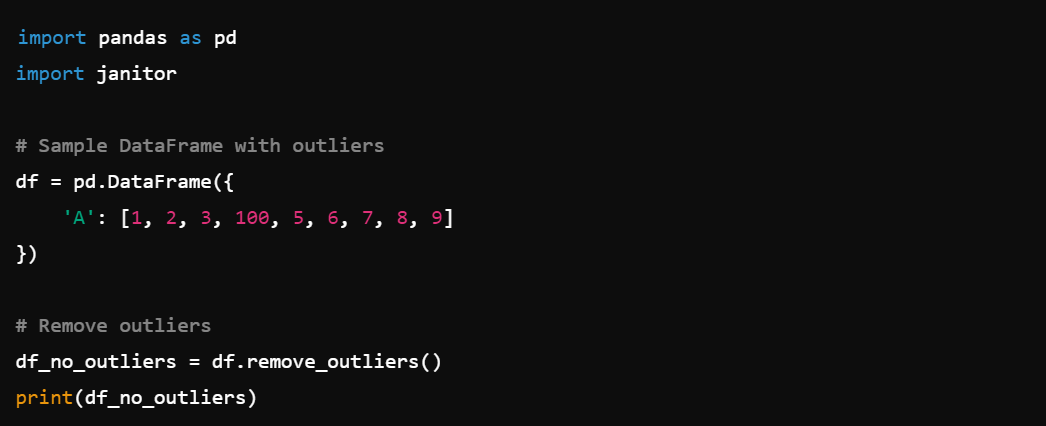
4. Encoding Categorical Data: Encode categorical data with df.encode_categorical().
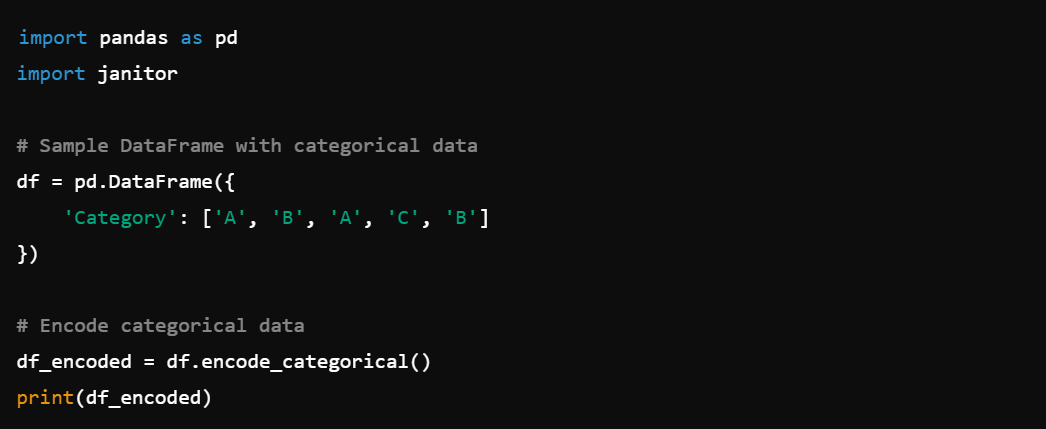
5. Data Imputation: Impute missing values with df.impute().
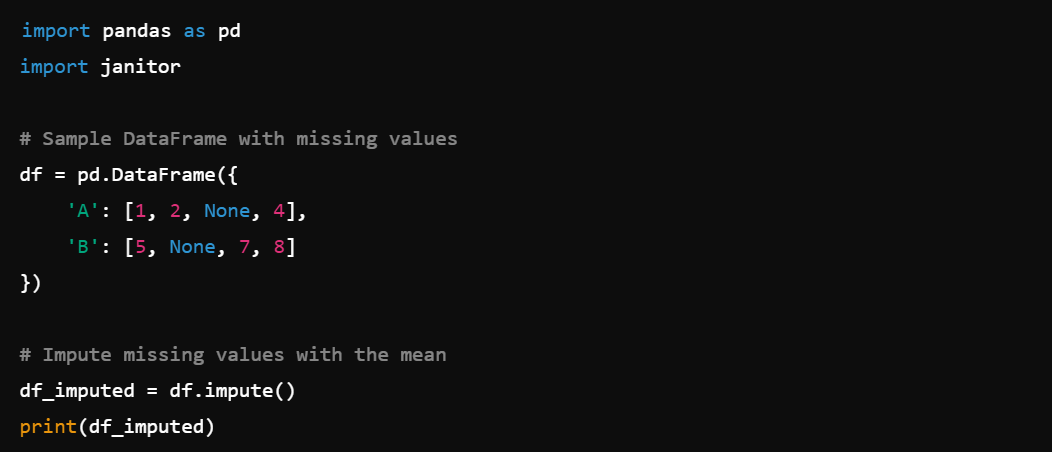
6. Expanding DataFrames: Expand lists in cells into separate rows with df.expand_column().
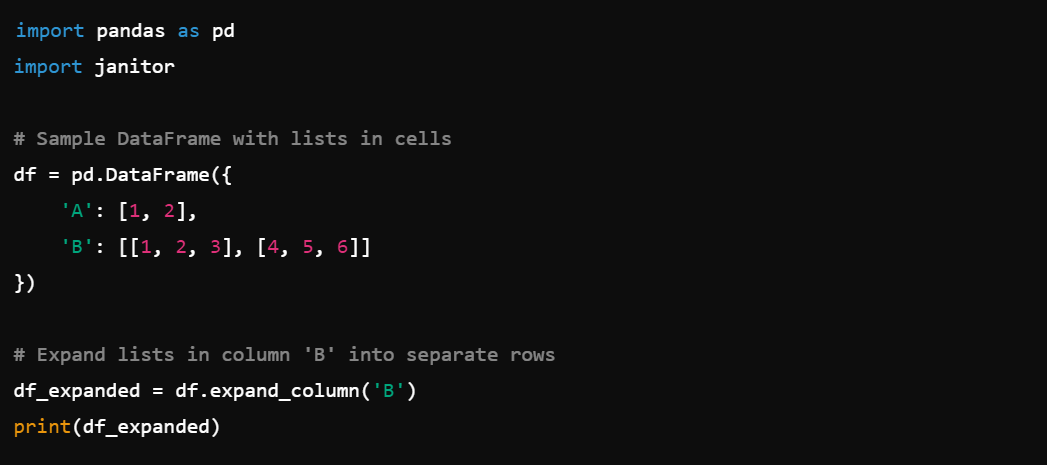
7. Concatenating DataFrames: Concatenate multiple DataFrames with df.concat().
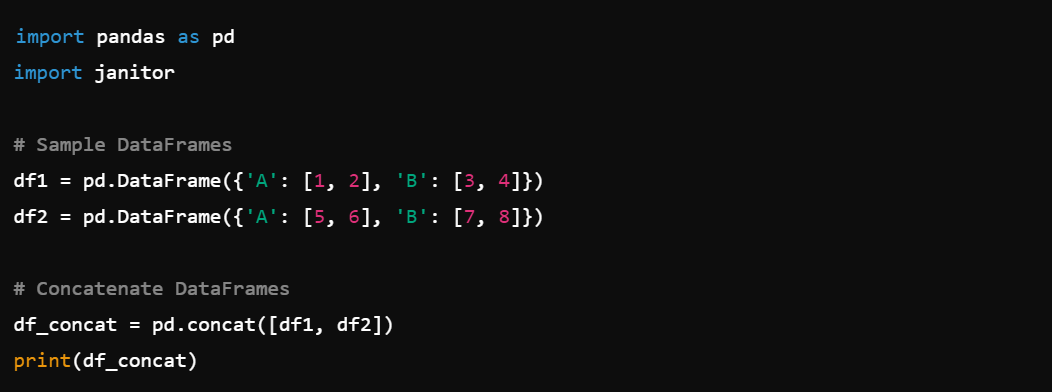
8. Moving Averages: Calculate moving averages with df.rolling().
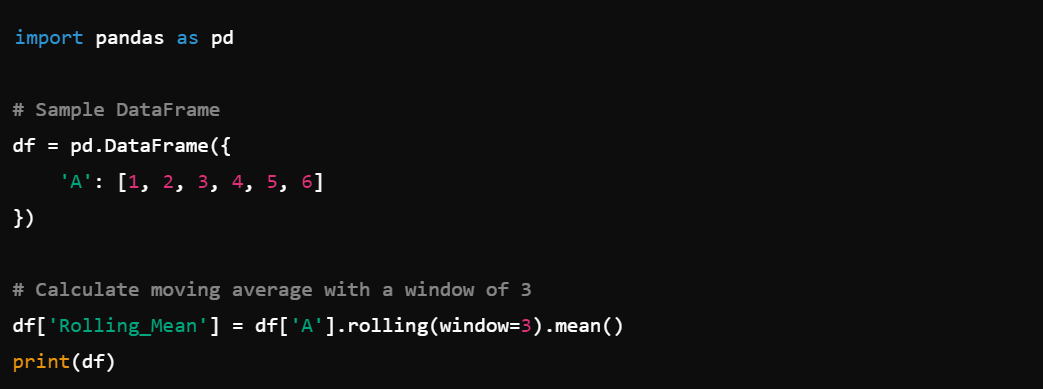
9. Conditional Column Creation: Create new columns based on conditions with df.case_when().
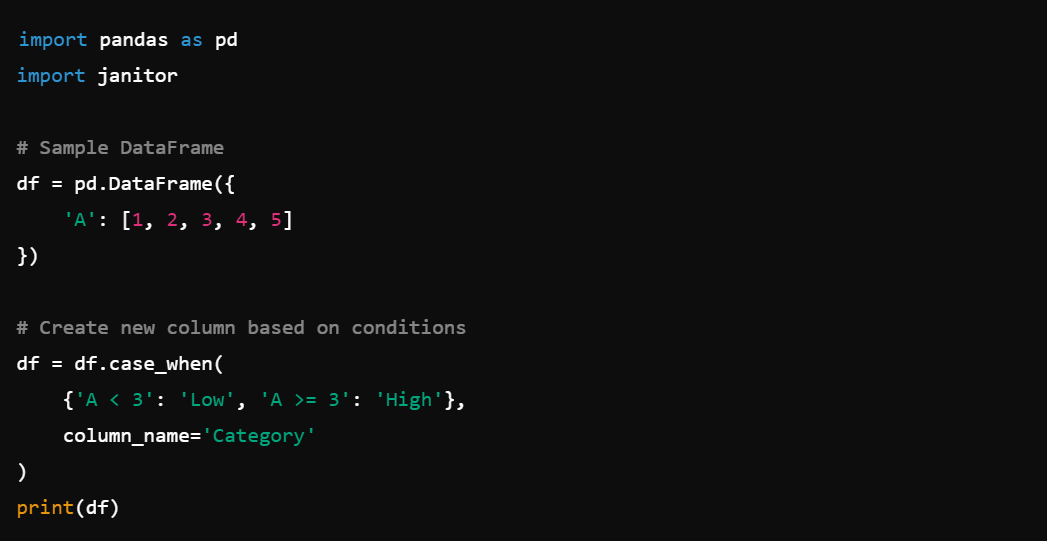
10. Data Normalization: Normalize data with df.normalize().
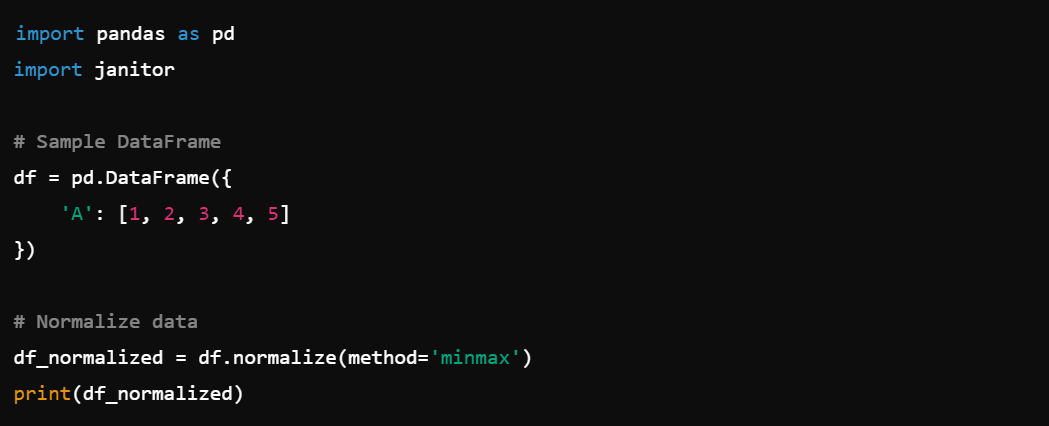
11. Label Encoding: Convert categorical labels to numbers with df.label_encode().
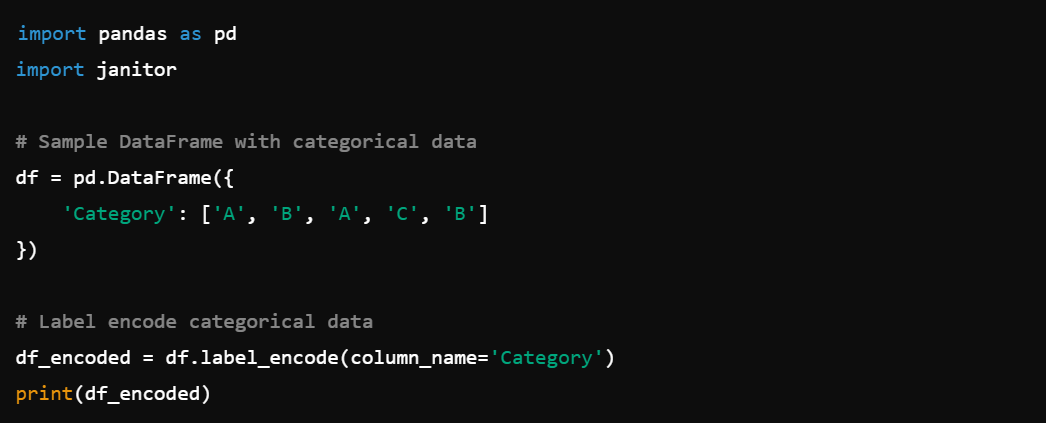
5. DataPrep
DataPrep is a library designed to speed up your data preparation workflows. Here are some of its capabilities.
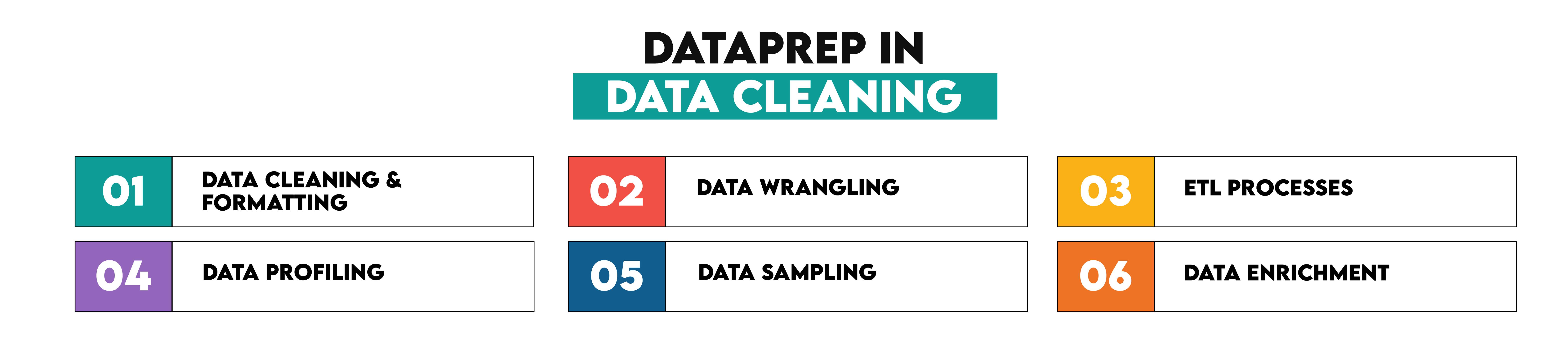
1. Data Cleaning: Use dataprep.clean to clean and format data, e.g., dataprep.clean.clean_missing().
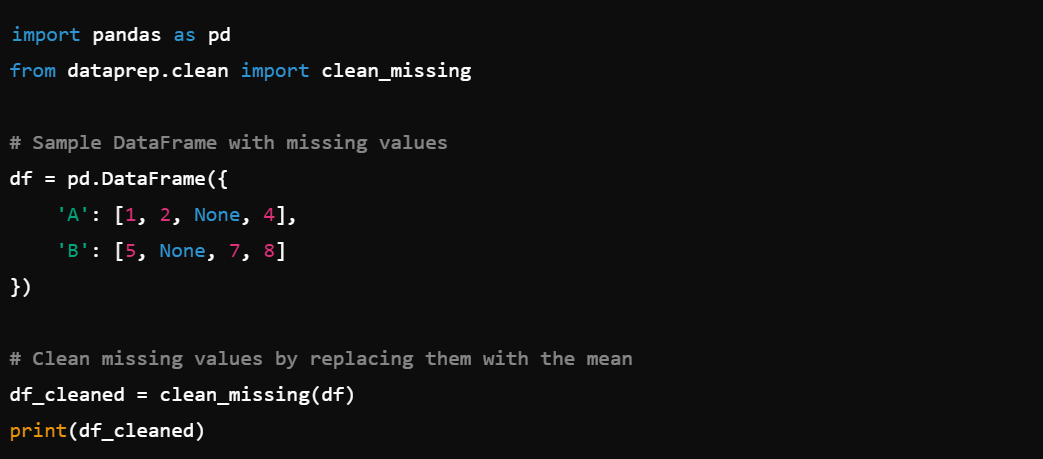
2. Data Wrangling: The dataprep.eda module helps in exploring and wrangling data efficiently, e.g., dataprep.eda.plot().
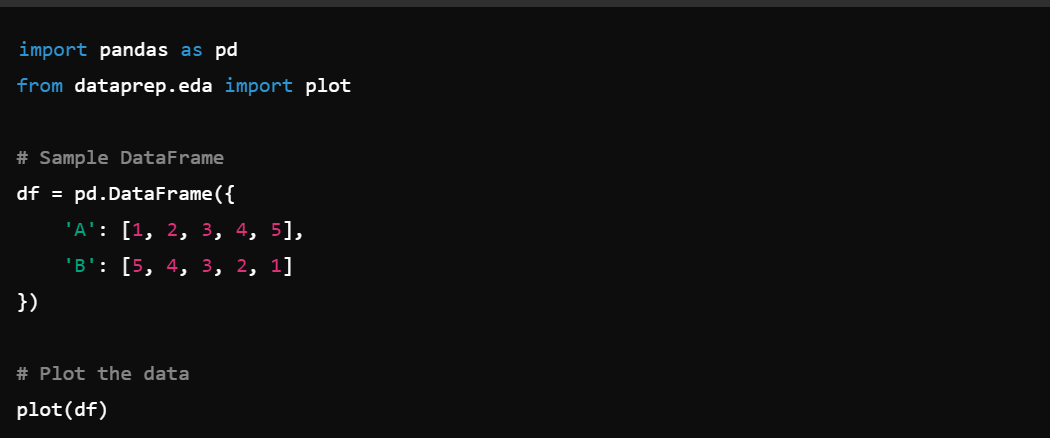
3. ETL Processes: Simplify ETL processes with dataprep.connector, e.g., connector.connect().
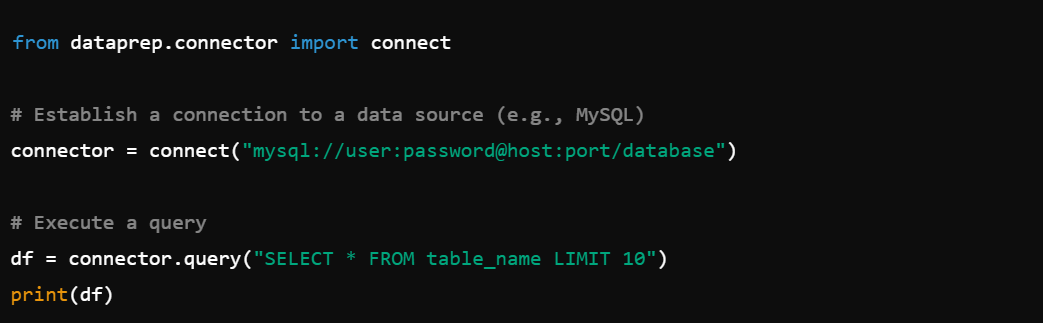
4. Data Profiling: Profile your data with dataprep.eda.create_report().
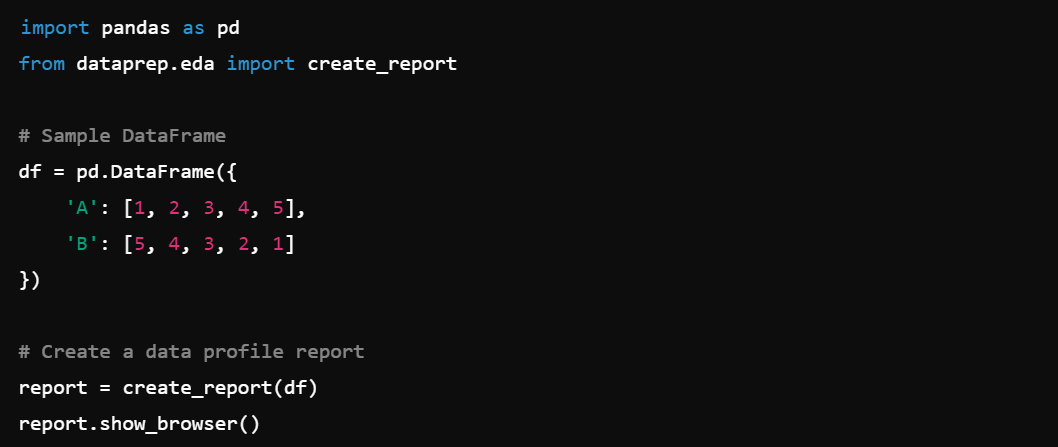
5. Data Sampling: Generate data samples with dataprep.eda.sample().
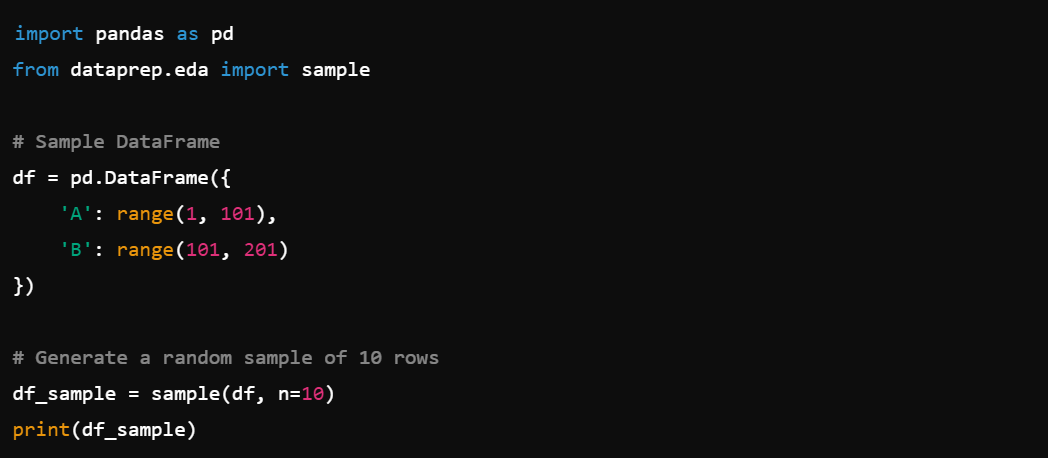
6. Data Enrichment: Enrich data by integrating additional datasets.
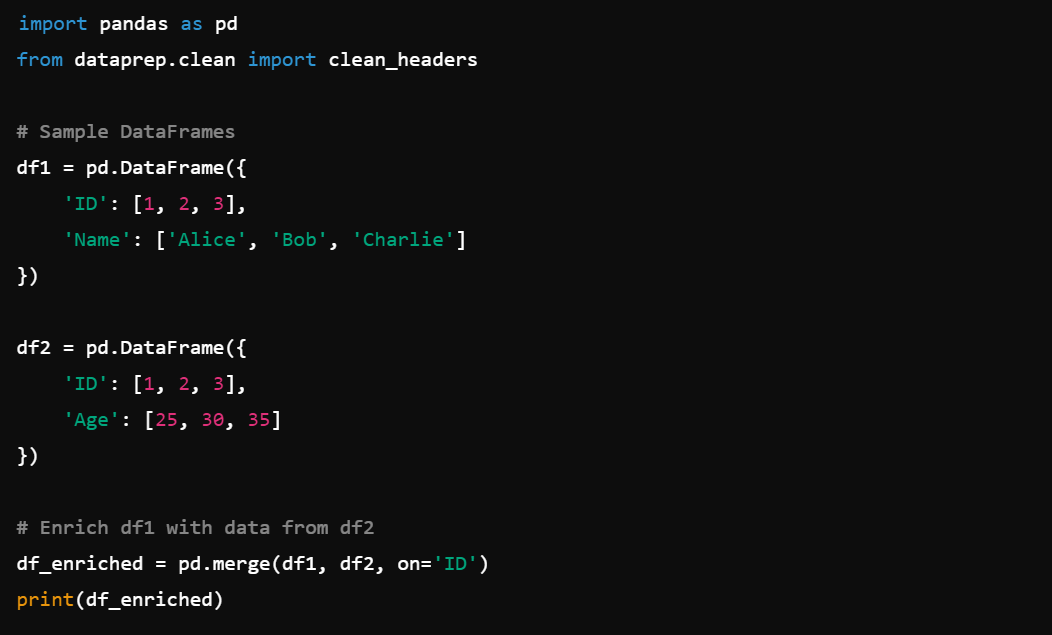
6. Great Expectations
This library focuses on data validation, testing, and documentation. It ensures your data meets quality standards before you proceed with analysis.
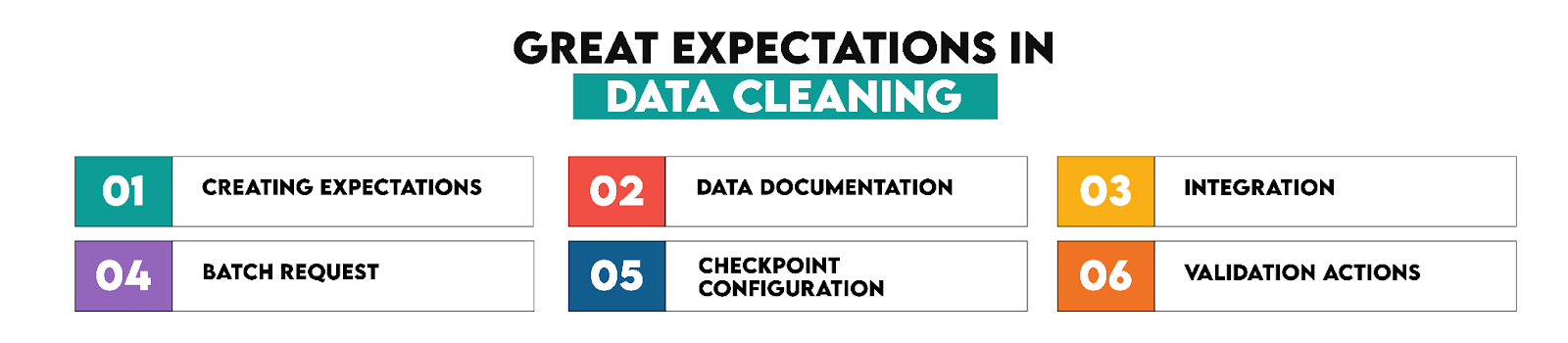
1. Creating Expectations: Define data expectations to validate data quality, e.g., expect_column_values_to_not_be_null().
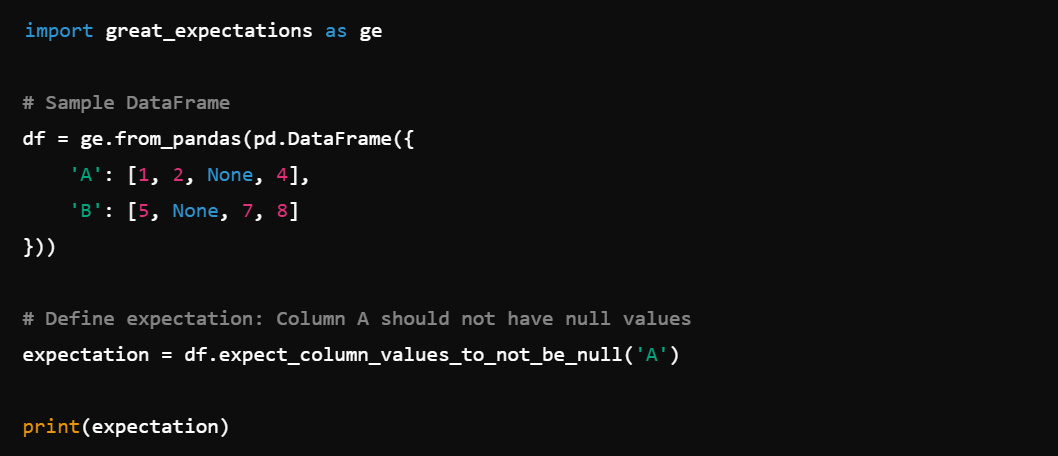
2. Data Documentation: Automatically document data expectations and validation results, e.g., data_context.build_documentation().
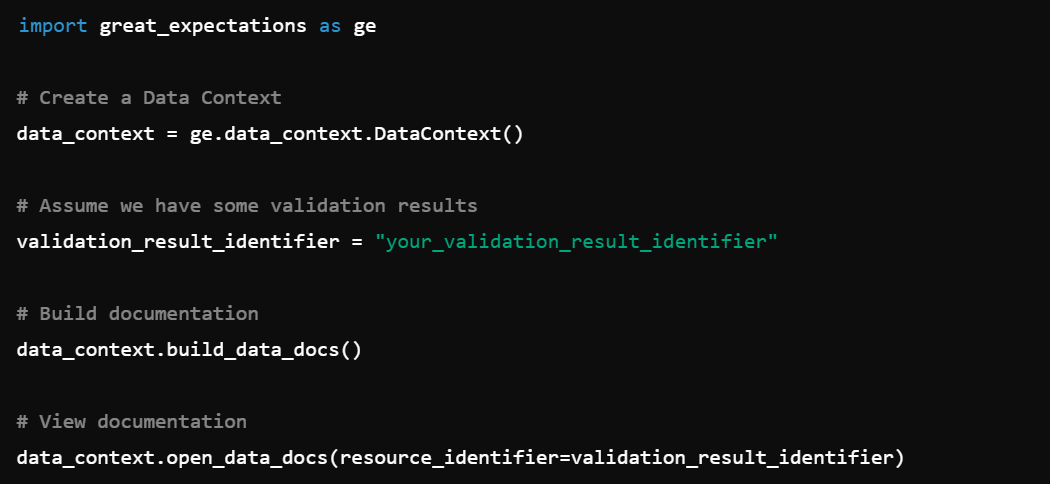
3. Integration: Easily integrates with existing data pipelines and tools, e.g., data_context.run_validation_operator().
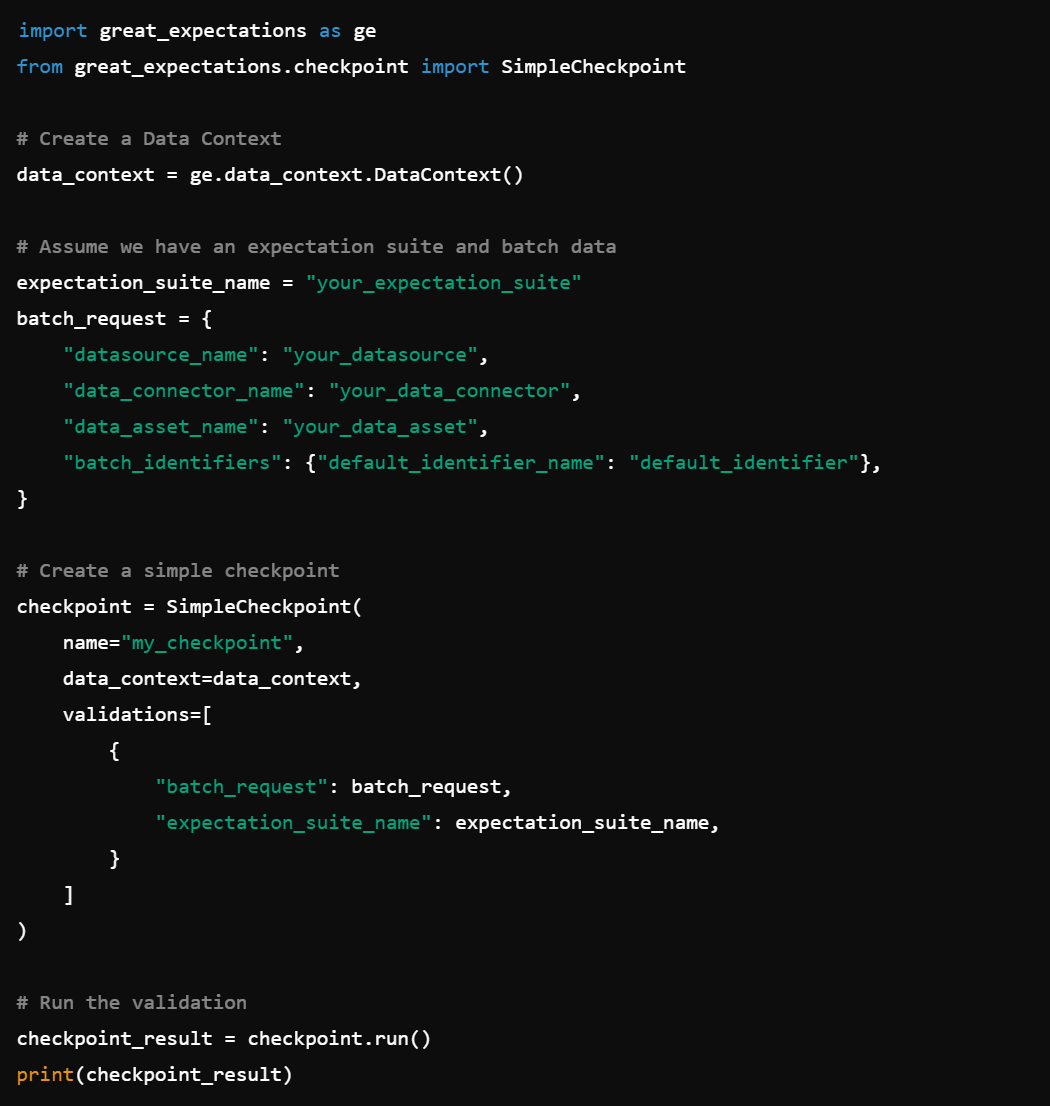
4. Batch Request: Validate data in batches with BatchRequest().
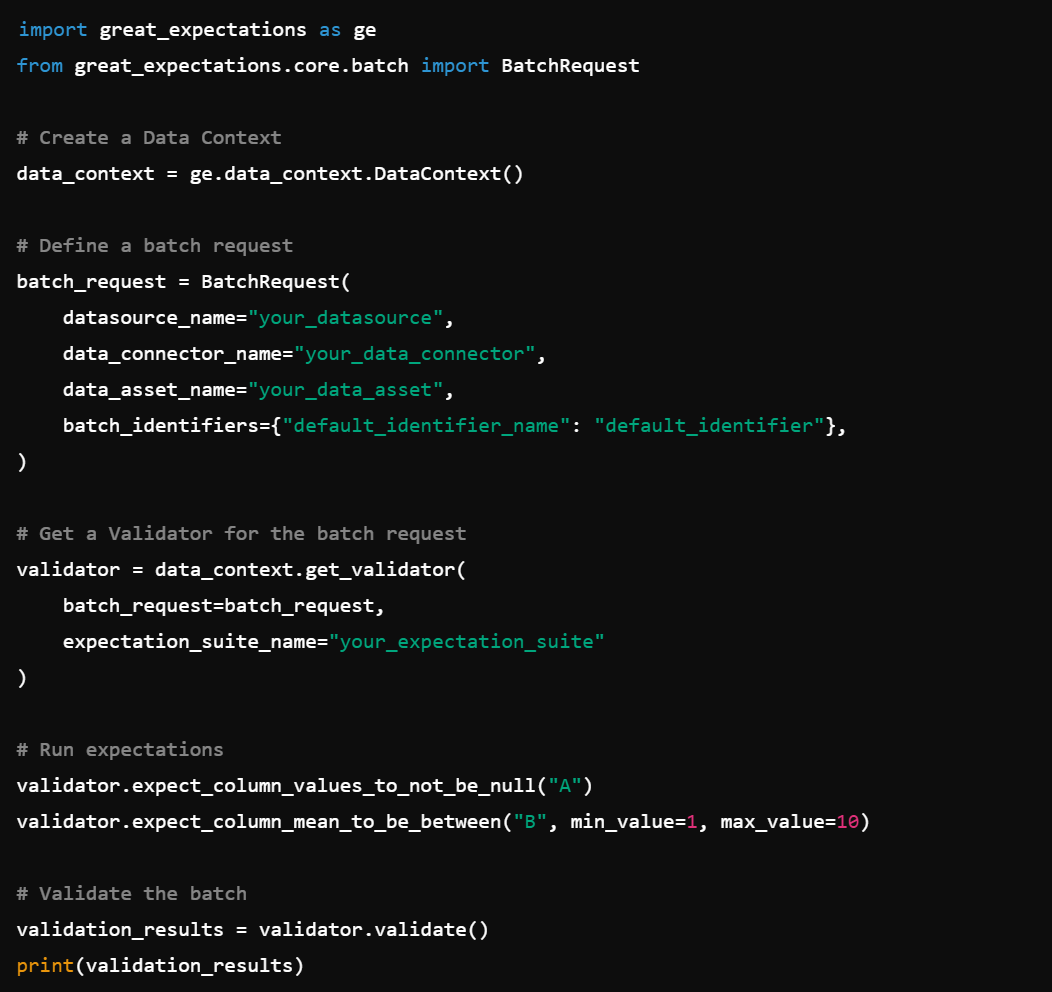
5. Checkpoint Configuration: Set up checkpoints for validation with Checkpoint().
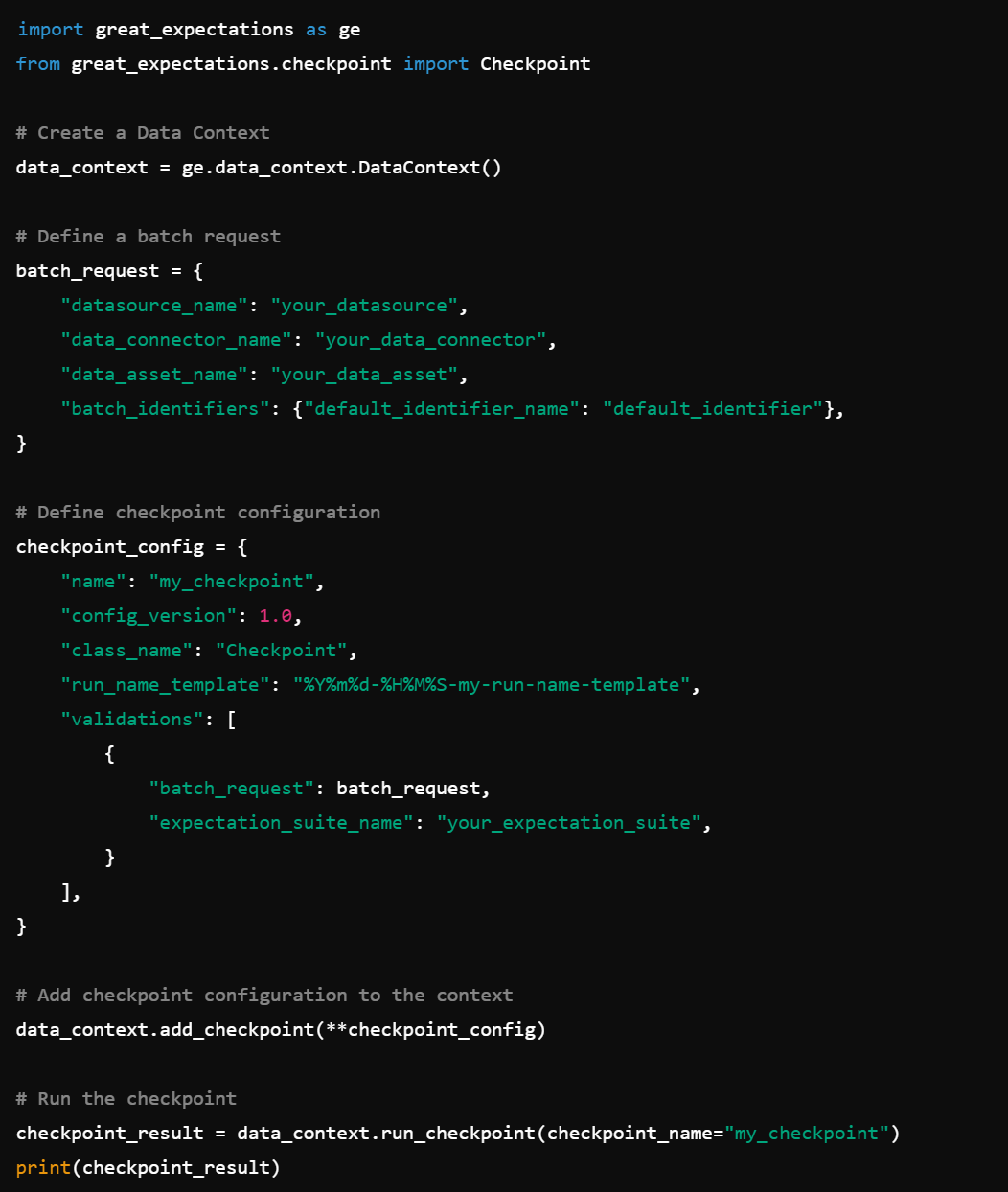
6. Validation Actions: Validate actions with functions such as StoreValidationResultAction(), StoreEvaluationParametersAction(), UpdateDataDocsAction().
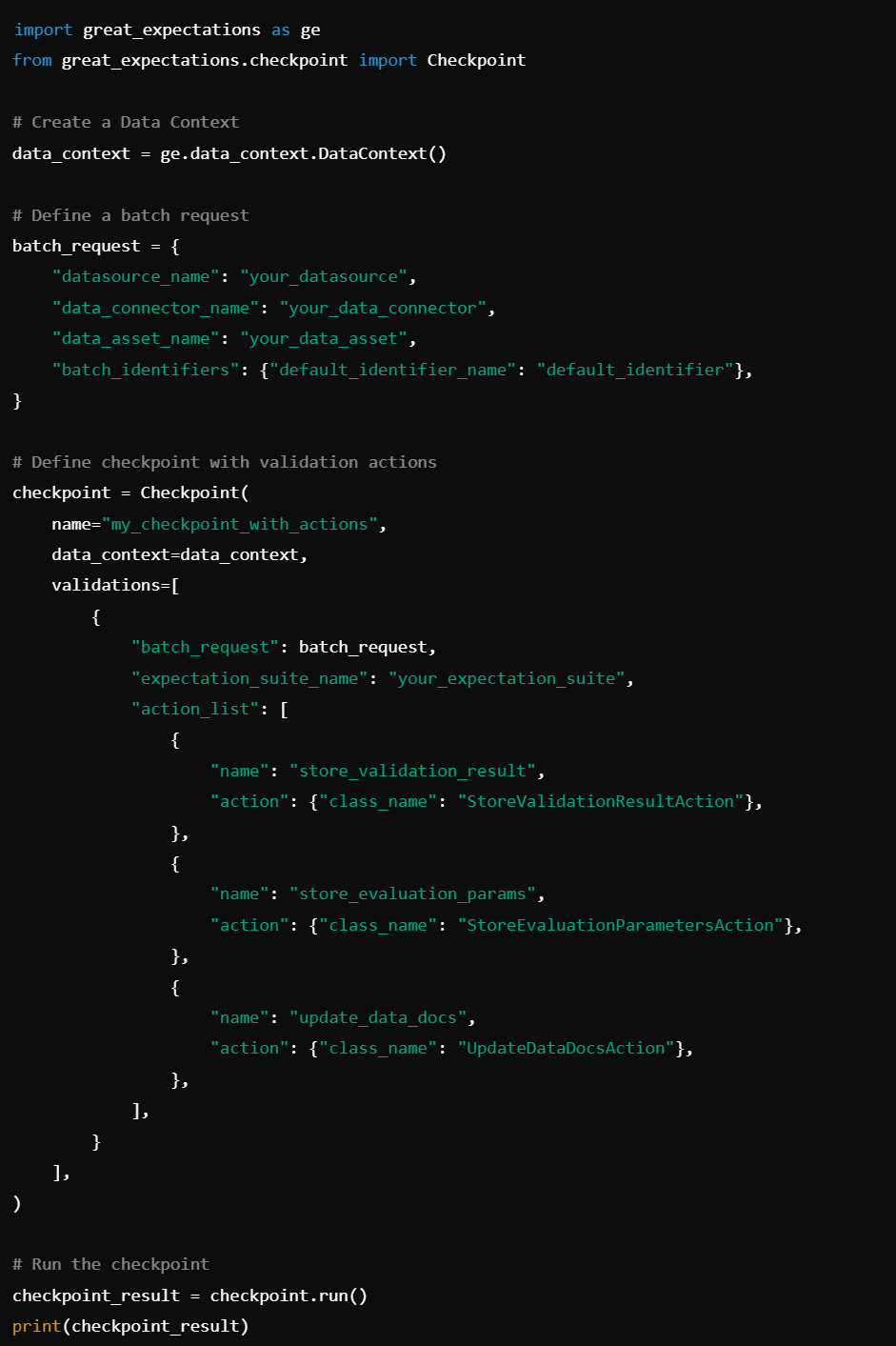
7. Pandera
Pandera is a library great for statistical data validation, maintaining data integrity and consistency.

1. Schema Definition: Define data schemas for DataFrames, e.g., pandera.DataFrameSchema().
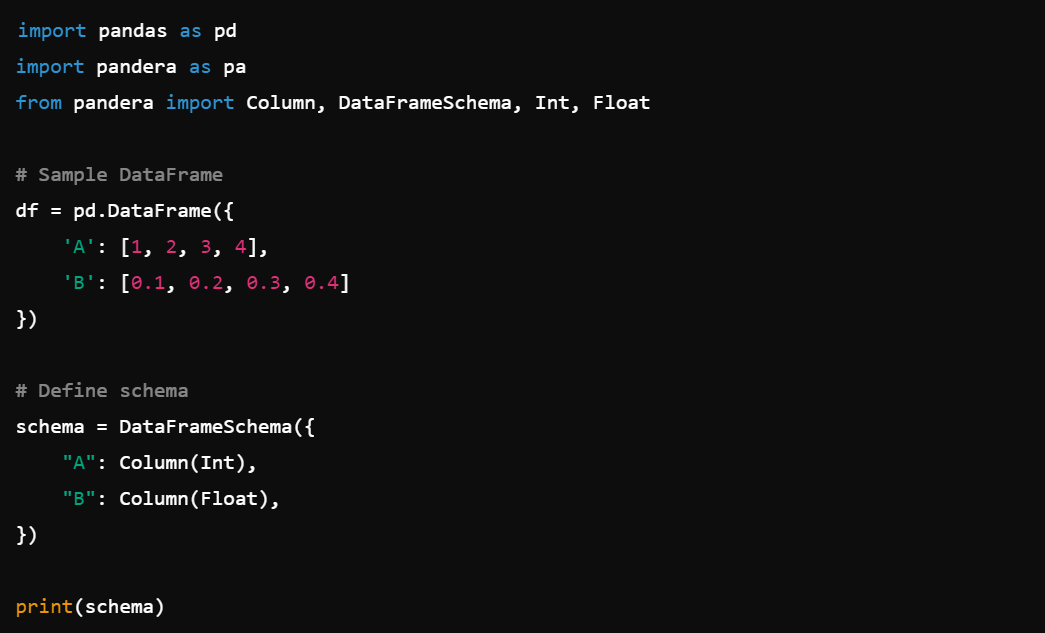
2. Data Validation: Validate data using predefined schemas, e.g., schema.validate().

3. Custom Validators: Create custom validation functions for complex data checks, e.g., pandera.Check().
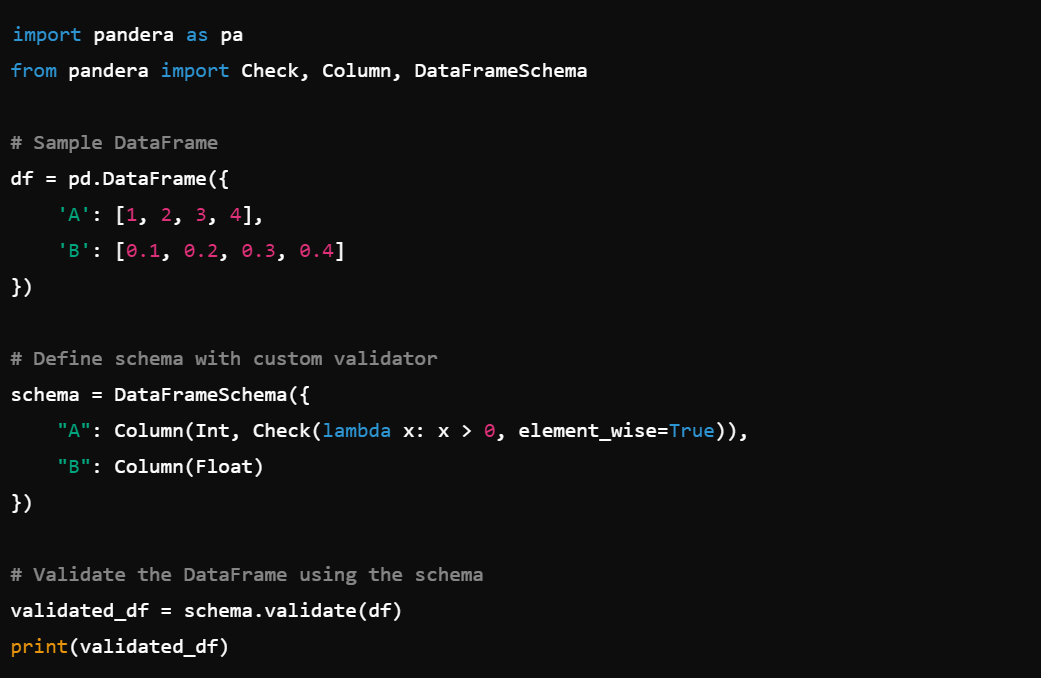
4. Schema Transformations: Transform data to fit schemas with schema.transform().
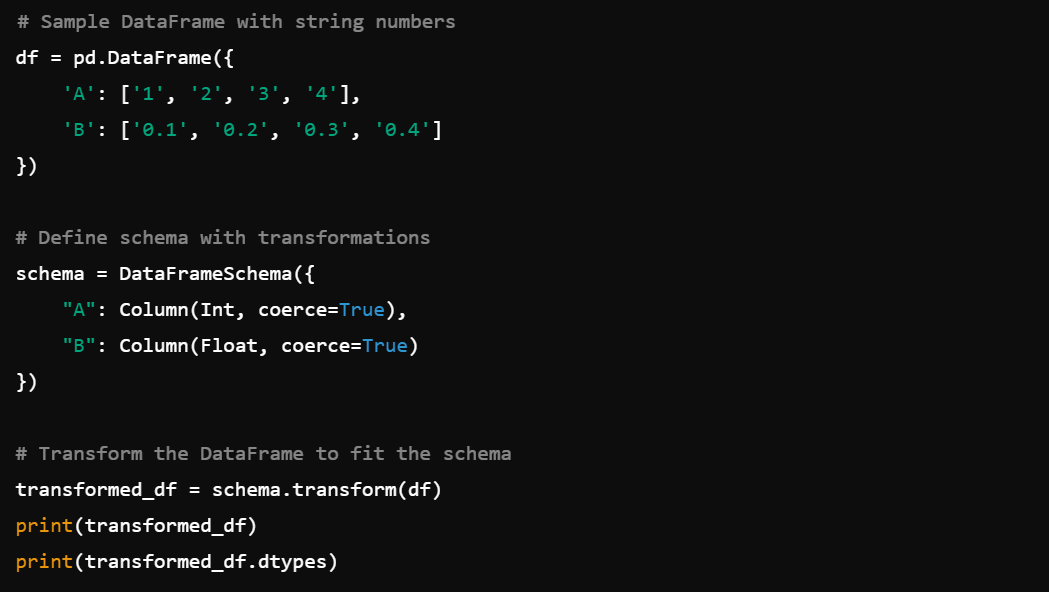
5. Batch Validation: Validate data in batches with SchemaModel.batch_validate().
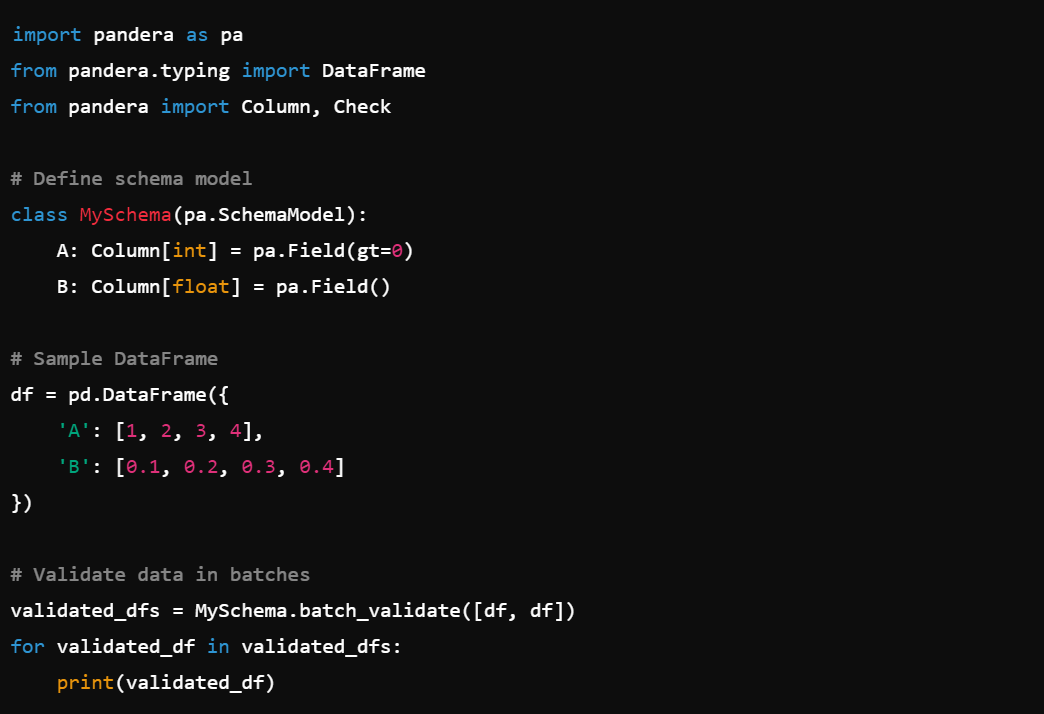
6. Conditional Checks: Apply conditions to schema checks, e.g., Check(lambda s: s > 0, element_wise=True).
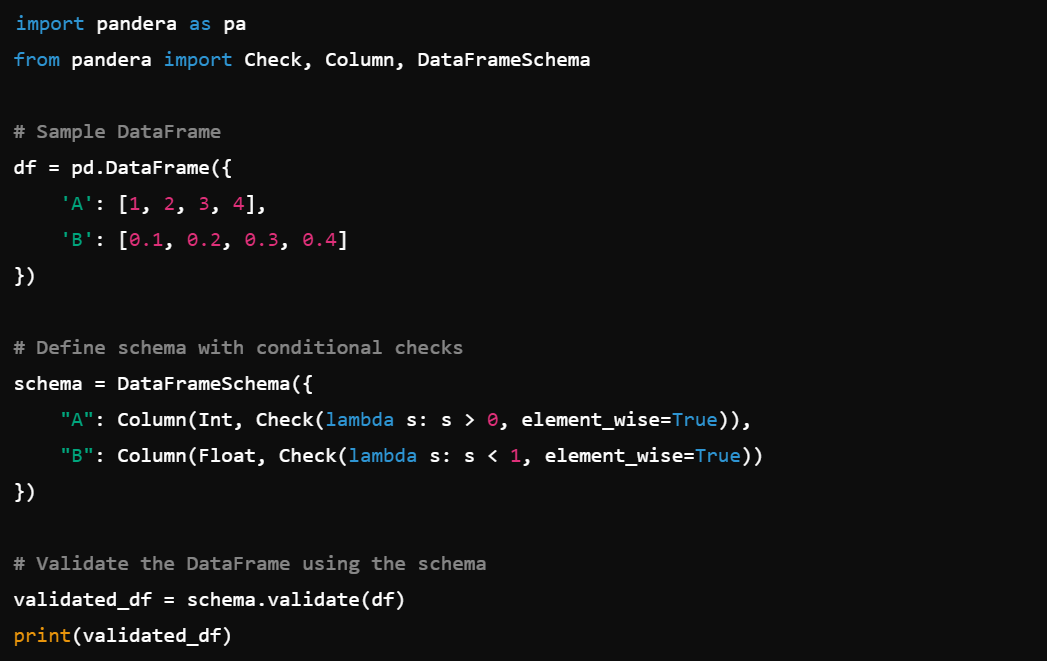
7. Column Coercion: Coerce column data types, e.g., Column(Int).
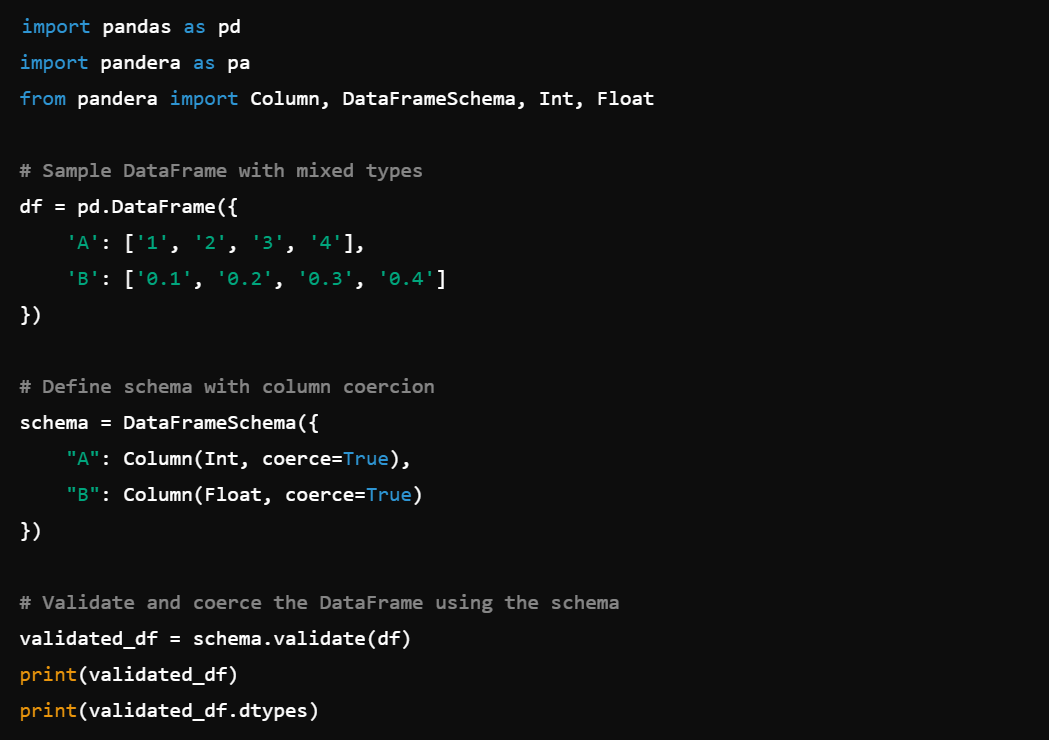
8. Error Handling: Customize error handling in validations, e.g., Check(lambda s: s > 0, element_wise=True, error='Values must be positive').
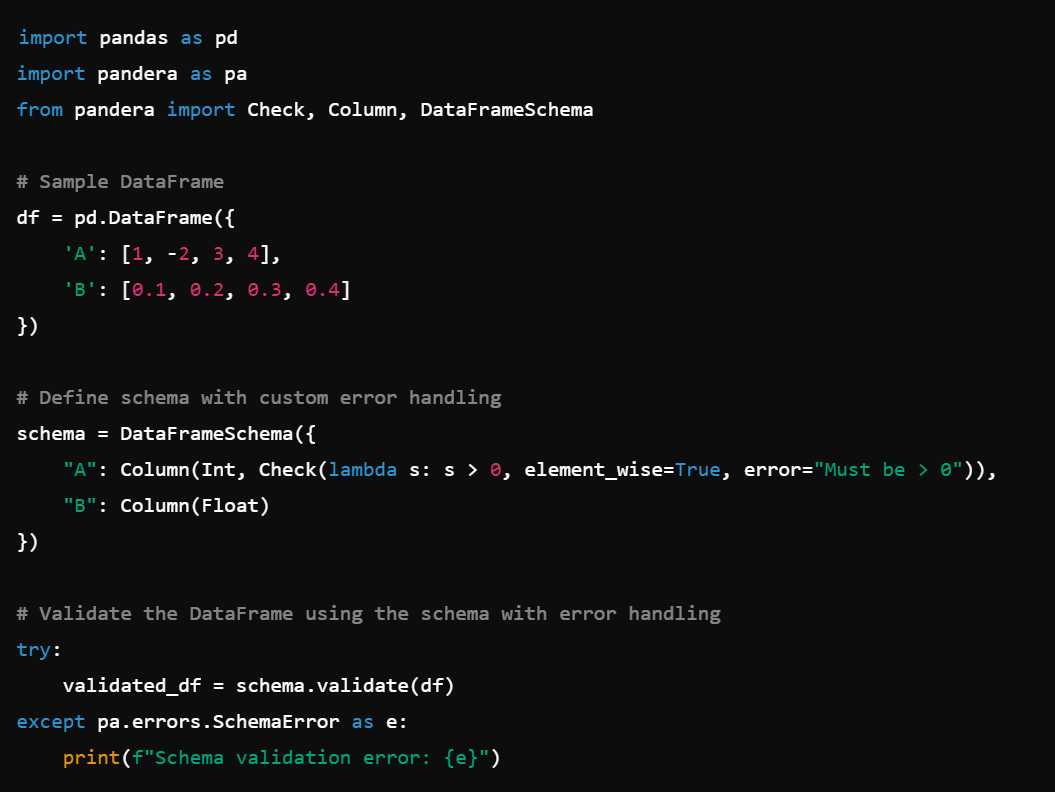
8. Dask
Last but not least – Dask. It is designed for parallel computing with large datasets.

1. Parallel Computing: Perform data cleaning tasks in parallel, e.g., dask.dataframe.
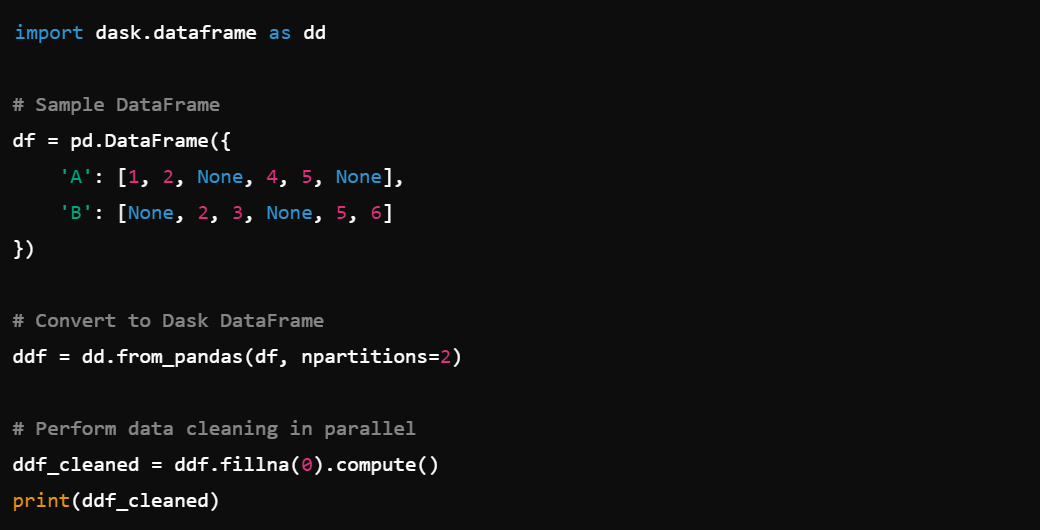
2. Big Data Handling: Efficiently handle large datasets that don’t fit in memory, e.g., dask.array.
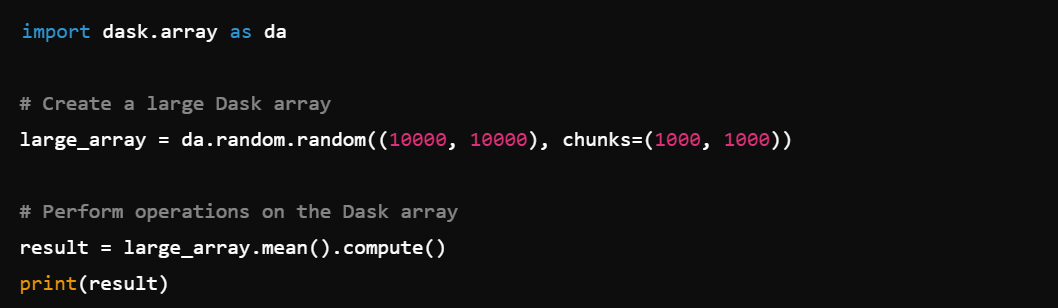
3. Integration: Works seamlessly with pandas for scaling data operations, e.g., dask.dataframe.from_pandas().
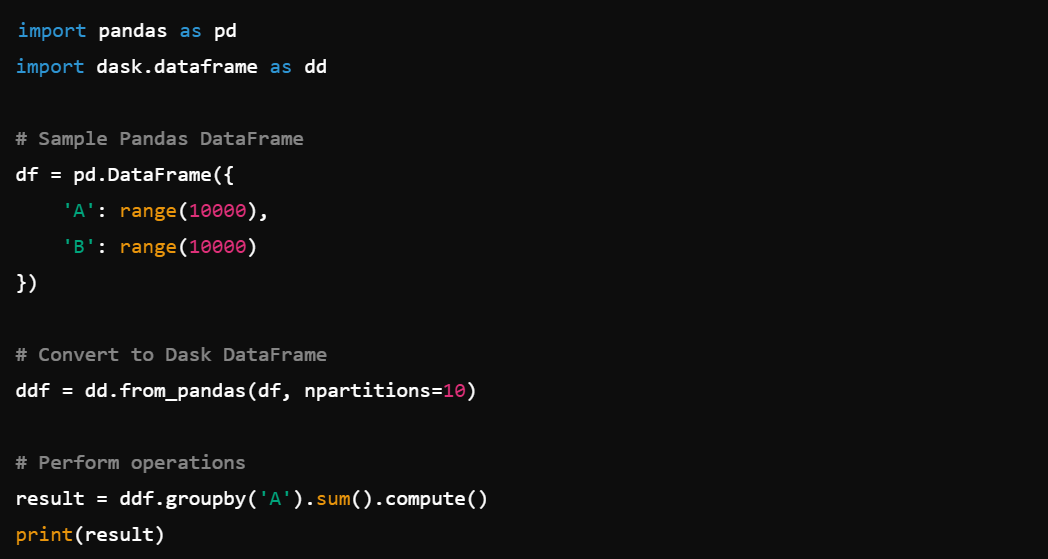
4. Delayed Execution: Use dask.delayed to parallelize operations, e.g., dask.delayed().
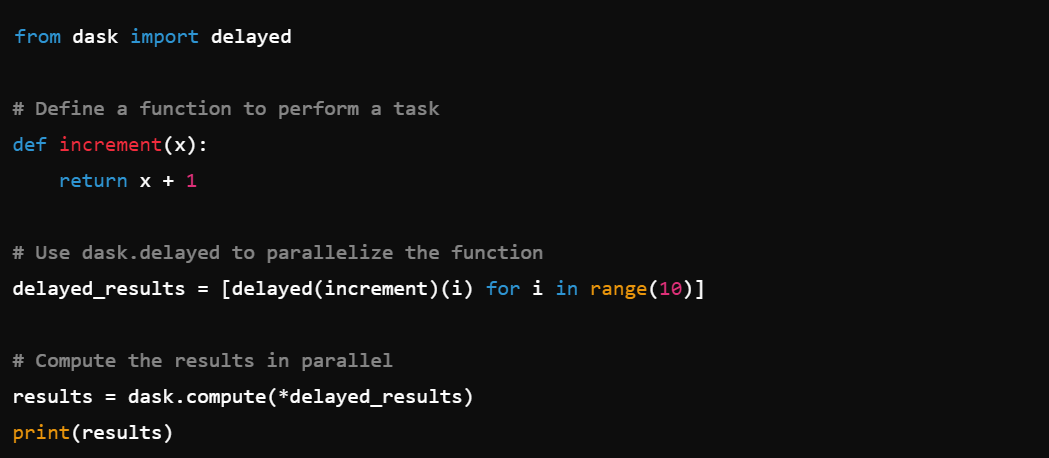
5. Task Scheduling: Schedule and optimize task execution with dask.compute().
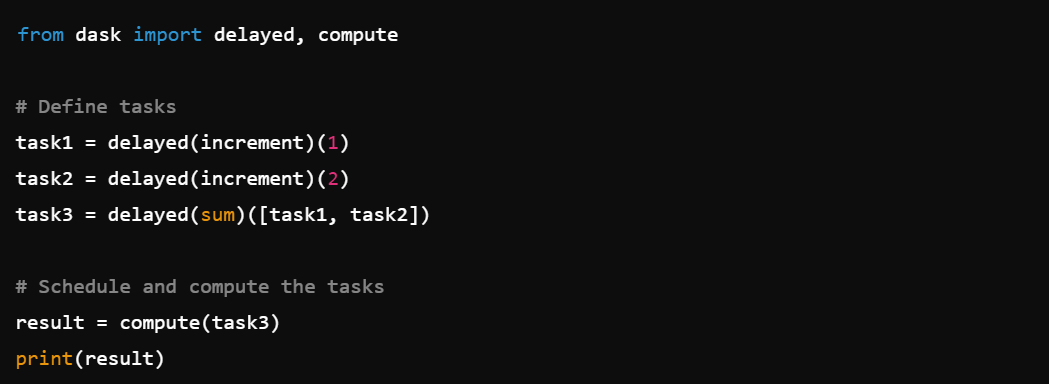
6. Array Operations: Perform operations on large arrays, e.g., dask.array.
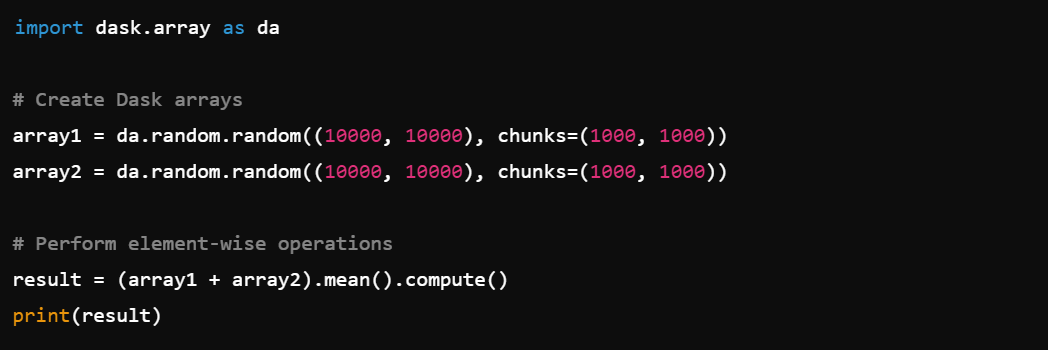
7. DataFrame Operations: Utilize dask.dataframe for handling large DataFrames, e.g., dask.dataframe.read_csv().
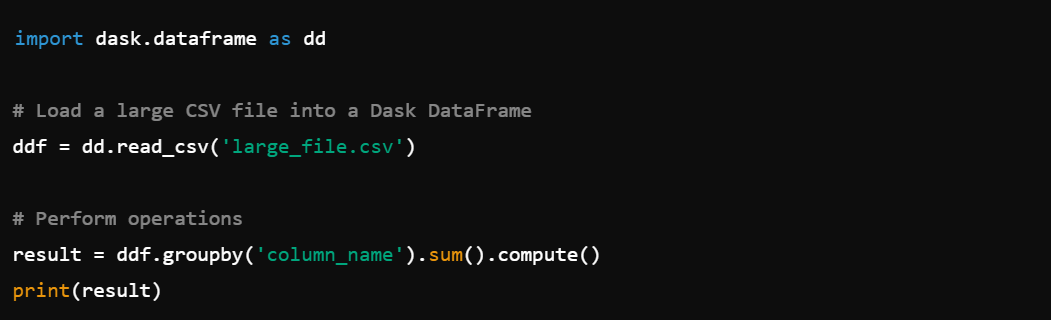
8. Bag Operations: Handle semi-structured or unstructured data with dask.bag.
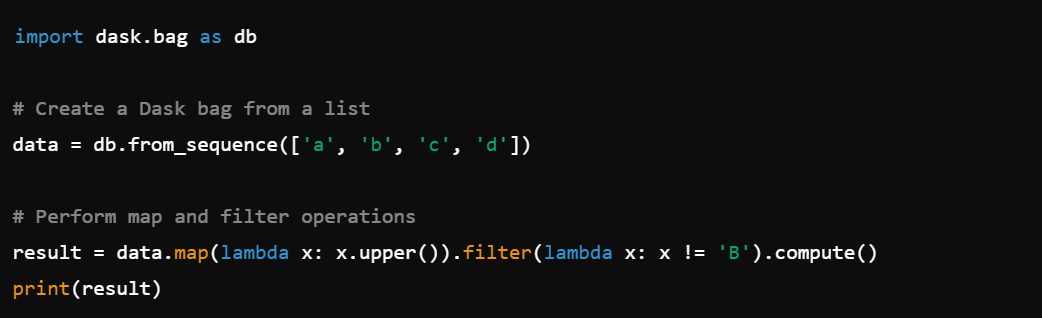
9. Machine Learning: Integrate with machine learning libraries, e.g., dask-ml.
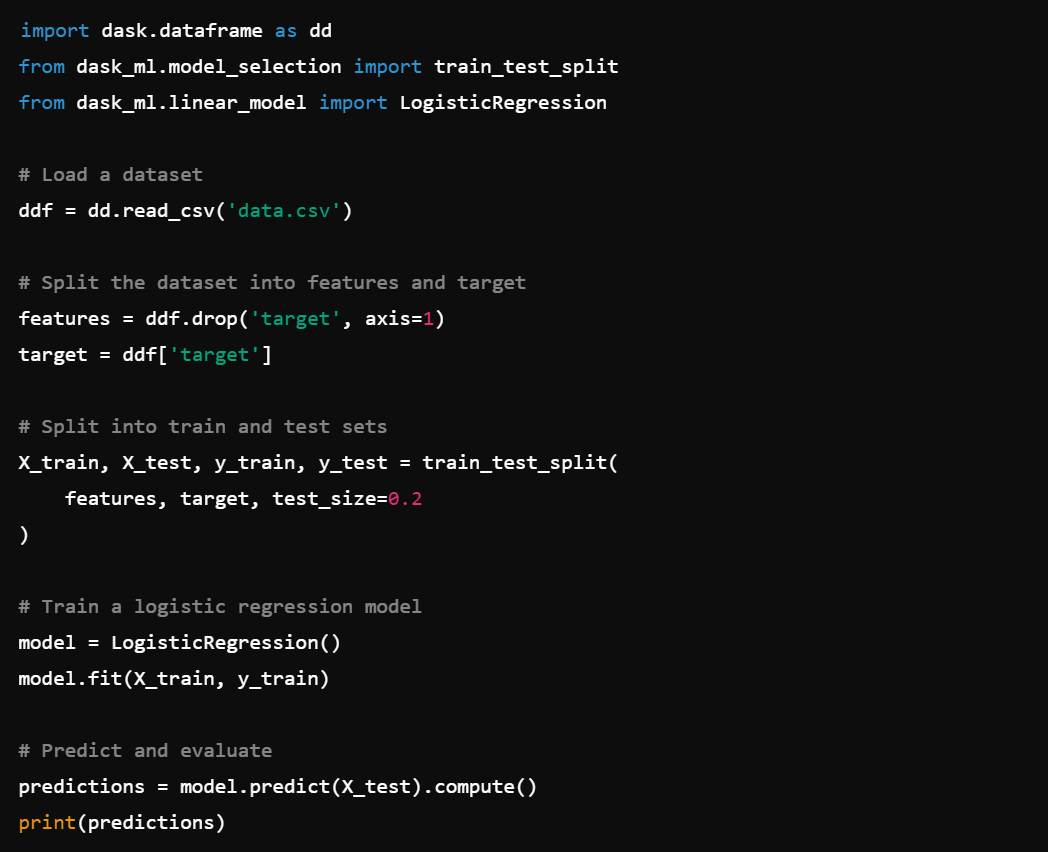
Conclusion
So there you have it – a plethora of powerful libraries to help you clean up your data. With pandas, NumPy, SciPy, Pyjanitor, DataPrep, Great Expectations, Pandera, and Dask, there’s something here for everyone.
Learn some of these libraries and enjoy your data cleaning!
Share