5 Tips to Prepare for a Data Science Interview
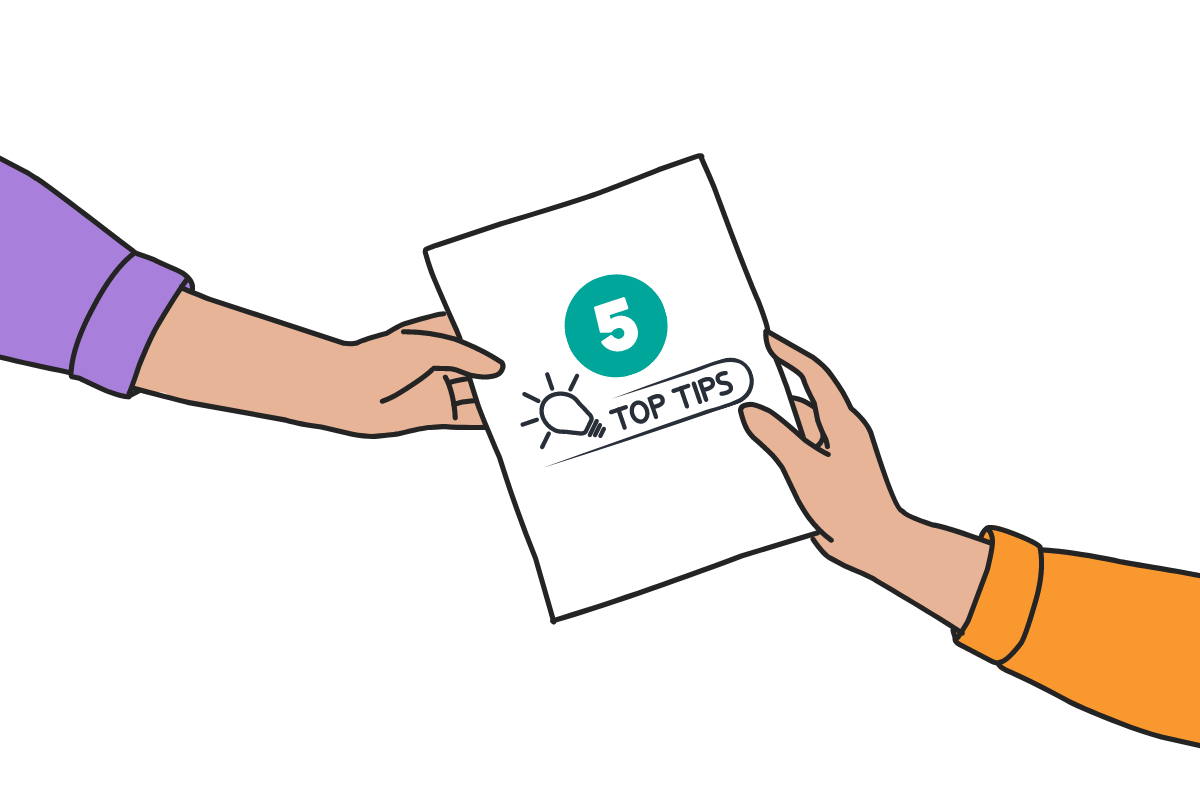
Categories
Are you wondering how to prepare for Data Science Interview? This data science interview preparation guide covers tips on topics covered during the interviews.
Data Science interview preparation is a big deal for everyone. Most of the candidates find it challenging to get through the recruitment process. Every interview is a new learning experience, even though you’ve appeared in many interviews. It can be a challenging situation because you will have to answer the baffling questions reasonably and satisfactorily. There are a wide variety of roles for which candidates apply in different companies. Therefore, they must be aware of the job roles and responsibilities for which they are applying. For example, if a candidate applies for a Data Scientist position, he must know that the employer will ask questions with lots of coding and algorithmic computing elements. These are the fundamental questions for which the candidate must be certainly prepared.
In this article, I will be giving you tips on certain data science interview topics like coding, behavioural questions, machine learning, modeling, statistics, and product sense. The goal of this data science interview preparation guide is to give you tips on how to successfully prepare for these topics because the interviewers will be testing you on these topics and it can be a very stressful situation. So, let’s start by first understanding the role of a data scientist.
What is the role of a Data Scientist?
A data scientist is an expert who gathers and analyzes large sets of structured and unstructured data. Therefore, they are also called data wranglers. All data scientists perform the job of combining various mathematical and statistical techniques. They analyze, process, and model the data, and then interpret it for deveoping actionable plans for the organization.
Data scientists are also analytical experts because they utilize their skills in technology to find the trends in data. They have to work closely with the business stakeholders to understand their goals and determine how they can achieve them. They design data modeling processes, create algorithms and predictive modes for extracting the desired data the business needs.
For gathering and analyzing the data, data scientists follow the below listed steps:
- Acquiring the data
- Processing and cleaning the data
- Integrating and storing the data
- Exploratory data analysis
- Choosing the potential models and algorithms
- Applying various data science techniques such as machine learning, artificial intelligence, and statistical modelling
- Measuring and improving results
- Presenting final results to the stakeholders
- Making necessary adjustments depending on the feedback
- Repeating the process to solve another problem
Data Scientist Categories
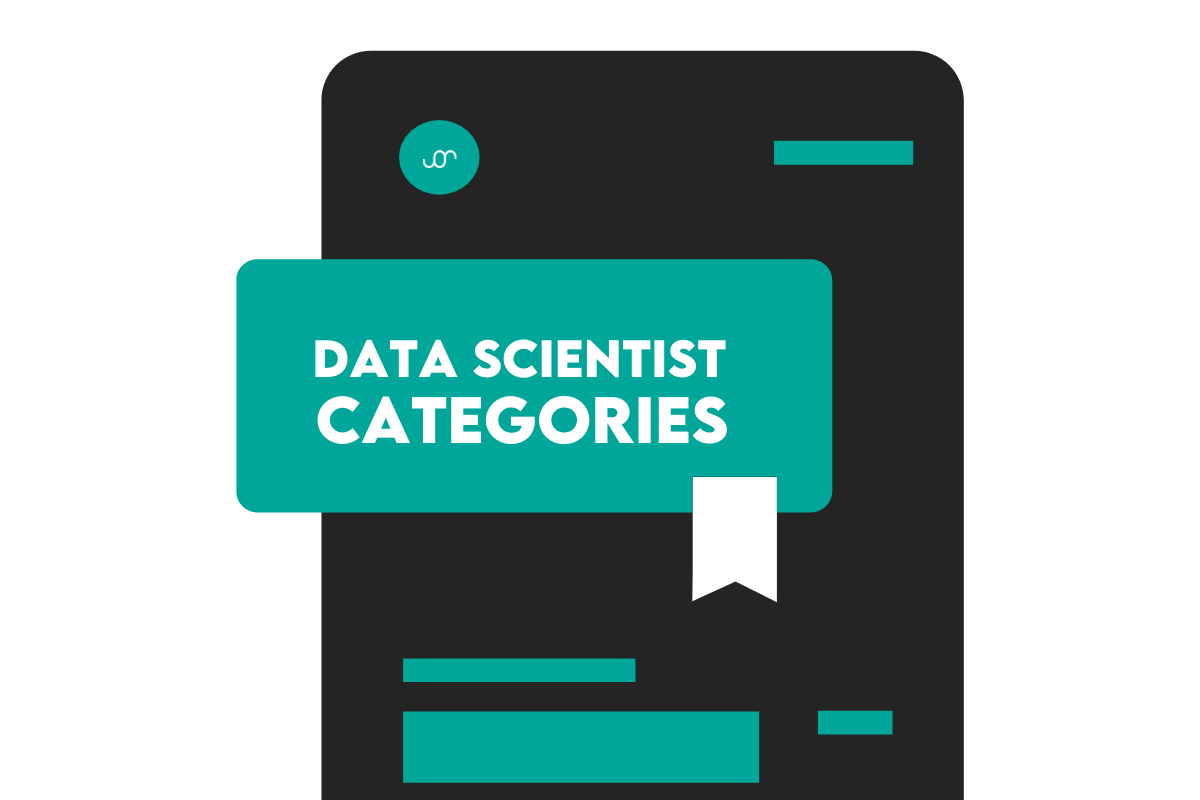
There are a number of data scientist roles which are mentioned as:
1. Data Analyst
Data scientists specializing in this domain typically have a focus on creating forecasts, providing informed and business-related insights, and identifying strategic opportunities. In short, they have a major focus on business intelligence. They create dashboards, devise solutions to various business-related challenges, and present data-backed findings to the company stakeholders in an accessible way. Therefore, they need data visualization tools like Tableau, and data warehousing skills are also required for creating forecasts.
2. Data Science Generalist
It is the most popular role, and companies hire many data science generalists that dive into big data sets for:
- Building simulations
- Writing optimization algorithms
- Building experimentation systems
- Running algorithms and models to find actionable insights
- Making meaningful recommendations
- Offering feedback to the company stakeholders based on their findings
3. Machine learning Engineer
If we talk about big tech companies, then the role of a machine learning specialist usually requires graduate or Ph.D. qualifications in Natural Language Processing (NLP), Deep learning, or Computer Vision. The data scientists in this domain mainly focus on cutting edge research in areas like Deep Learning, NLP, streaming data analysis, video recommendations, and social networks, etc. to assist the company in the development of new algorithmic models that power the company's streaming services, Web Services, and other business parts.
4. Data Engineer
The Data Engineering team focuses on building products or tools used inside and outside the company. In addition, it builds out data pipelines, and its role significantly overlaps with the Machine Learning engineers.
5. Statistician
The job of the statistician is to deal with both theoretical and applied statistics for achieving the required business goals. He possesses key skills such as data visualization that can be inferred to acquire expertise in specific data scientist fields.
5 Tips to Prepare for a Data Science Interview
Let’s have a look at the following tips that a data aspirant must follow in order to successfully get through the data science interview:
Data Science Interview Preparation Tip # 01 - Practice Coding Questions
What are data science coding questions? These are the questions that require coding in any programming language to get the desired answer. You have to get through the coding interview if you are applying for a data science job.
Purpose of Coding Questions
Here’s why you are asked these questions:
- You know that data science is a technical field in which you have to collect, clean and process data into usable formats. So, the coding questions test not only your technical skills but also determine your thought process and approach you use to break down the complicated questions into simpler solutions. Therefore, preparing fundamental coding concepts are a must to ace the data science interview.
- These questions also test whether you use a logical approach to solve real-world problems or not. It’s true that there are multiple solutions to a single problem but the goal is to find the solution that is optimized in terms of run time and storage. So, you must be able to come up with the optimal solution to any real-world problem.
- The interviewer also evaluates your overall code quality by checking whether you consider all edge cases into your solution or not.
Practice Coding Questions
As you know now the importance of the coding questions, you must prepare yourself to solve them appropriately in a given amount of time. For this, you need to practice as many data science interview questions as you can to gain a better insight into different scenarios. Try to focus more on real-world problems. This way you will be able to break down complex questions into simple parts by logically coming up with an optimal solution. You can practice lots of problem statements from LeetCode, GlassDoor and our very own Stratascratch. Don’t get discouraged by the types of questions that may appear daunting to you at first sight. You will take time to prepare them but for that, you must have a good grasp of the basic programming concepts and machine learning algorithms. In order to achieve a more comprehensive understanding, you may also come up with multiple solutions to a single problem, compare their strengths and weaknesses to select the best possible approach.
Now let’s see a real question example from the StrataScratch platform.
Here is the question from Microsoft Interview.
Interview Question Date: November 2020
We have a table with employees and their salaries, however, some of the records are old and contain outdated salary information. Find the current salary of each employee assuming that salaries increase each year. Output their id, first name, last name, department ID, and current salary. Order your list by employee ID in ascending order.
Link to the question: https://platform.stratascratch.com/coding/10299-finding-updated-records
In this question, Microsoft asks us to find the current salary of each employee assuming that salaries increase each year.
The reason for finding this was explained that some of the records contain outdated salary information.
Here is our data frame, the name is ms_employee_salary.
id | first_name | last_name | salary | department_id |
---|---|---|---|---|
1 | Todd | Wilson | 110000 | 1006 |
1 | Todd | Wilson | 106119 | 1006 |
2 | Justin | Simon | 128922 | 1005 |
2 | Justin | Simon | 130000 | 1005 |
3 | Kelly | Rosario | 42689 | 1002 |
The expected output contains the id, first name, last name, department ID, and current salary.
Now, let’s start by exploring our dataset first. Let’s look at it closer by using the head method.
ms_employee_salary.head()
Here is the output.
id | first_name | last_name | salary | department_id |
---|---|---|---|---|
1 | Todd | Wilson | 110000 | 1006 |
1 | Todd | Wilson | 106119 | 1006 |
2 | Justin | Simon | 128922 | 1005 |
2 | Justin | Simon | 130000 | 1005 |
3 | Kelly | Rosario | 42689 | 1002 |
As we can see from the output, there are many different salaries exist for the same people. Mainly, the question asks us to find the maximum salaries of employees, because that means this one their final salary due to regular increases made.
First, let’s load the numpy and pandas to be able to do further analysis.
import pandas as pd
import numpy as np
To do that first, we should select first_name, last_name, salary, and department_id, since our question wants us to input these.
To do that, we can use the groupby() method as follows.
ms_employee_salary.groupby(['id','first_name','last_name','department_id'])
Yet, we should find the maximum value of salary, so should first select salary with bracket indexing and then max() method in Python to find the maximum salary.
ms_employee_salary.groupby(['id','first_name','last_name','department_id'])['salary']
Great, now, let’s reset the indexes. Since we use the groupby() method, our id set as our index. Let’s reset_index() and then sort_values() by id, to see id ordered DataFrame, as we saw before beginning.
import pandas as pd
import numpy as np
result = ms_employee_salary.groupby(['id','first_name','last_name','department_id'])['salary'].max().reset_index().sort_values('id')
Here is the output.
id | first_name | last_name | department_id | max |
---|---|---|---|---|
1 | Todd | Wilson | 1006 | 110000 |
2 | Justin | Simon | 1005 | 130000 |
3 | Kelly | Rosario | 1002 | 42689 |
4 | Patricia | Powell | 1004 | 170000 |
5 | Sherry | Golden | 1002 | 44101 |
As we can see, it matches with the expected output.
Communicate your thought process
What if you know how to solve a problem but don't know how to communicate it. Practice improving your communication skills because you must be able to explain your solution to other people to reinforce understanding.
You can follow the below preparation tips to effectively communicate your thought process to the interviewer:
- Conduct a mock interview with your peers as it will actually help you in better delivery of your concepts.
- In case you are not able to do that, you can conduct a session with yourself and practice in front of a mirror. You can also write down the main points you’ll be going to say in the interview.
- Finally, you can watch tons of mock interview videos of people in the Data Science community on YouTube. You can follow our very own channel as there’s a lot for everyone to learn.
Data Science Interview Preparation Tip # 02 - Practice Product Questions
No one is good at product questions unless they have seen them before. Product interview questions are the specific type of interview questions that aim to test your ability to understand how to build products and how you would respond to the natural life cycle of a product.
Are you aware of the significance of product interview questions? If not, then here’s the answer to this question. Actually, data scientists don’t work in isolation. They usually work with a project manager or a business based person and contribute directly to the product that is to be built. That is why you need to have a clear understanding of the product that needs to be built so that you can align the work you do and can actually implement it in the product.
The interviewers ask product questions because they are actually looking for the following five things:
- Analytical and Logical Thinking
If you have a product, you must be able to translate it into a way that can be solved with data science. So, the interviewers look for whether you are able to take the context that’s over there in the business side and can actually translate that into a problem that can be solved using data science.
- Product Sense
Product sense refers to your understanding of the product as a whole. It’s not about solving problems and getting stuck in the technical details rather it is about having a clear understanding of the context. You must know the purpose of the product you are building, why it is important to you, and how you can use this product to serve people.
- Communication
You must be able to communicate your thought process and understanding of the problem to the partners you are working with.
- Problem Solving Abilities
Problem-solving ability does not imply that you know what the problem is. It implies that you must know how you can use data science to solve the problem under consideration. So, you must be able to come up with a framework or an optimal approach to solve the problem and result in the production of a better product.
- Flexibility
You must be flexible because in the real industry environment as things pop up that never actually go as expected. So, this is the part where the interviewers test if you are able to adapt to these changes where they are going to throw you off.
How to Prepare Product Questions for Data Science Interview
Now, let’s have a look into how you can practice the product questions. In actual, it’s hard to find a lot of product interview questions and it’s even harder to find the solutions from all over the internet in data science. But their in-depth analysis reveals that these questions are similar to product management and management consultant questions. So, what you need to do is to look at some of the management consultant frameworks in a way that they approach business questions and apply that to a specific product. This is how you can answer product questions well in a data science interview.
Now let’s discover a product question from our platform asked by Yelp in an interview.
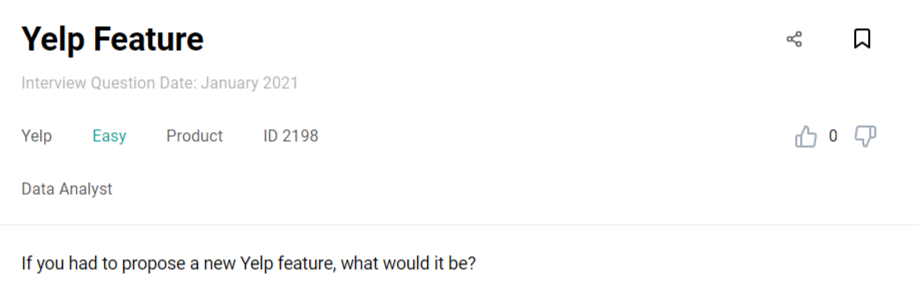
In this question, yelp asks us to propose a brand new Yelp feature.
Yelp is a go-to platform for people looking for local business reviews, particularly for dining options. While Yelp already offers many useful features, one feature that could be a game-changer would be price comparison.
Most of us would love to dine at a highly-rated restaurant, but budget constraints often hold us back. Therefore, integrating a feature that allows users to see menu prices for different restaurants and compare them would be highly valuable.
This feature would enable users to make more informed decisions and help them find the best dining options that fit their budget.
Data Science Interview Preparation Tip # 03 - Practice Behavioral Questions
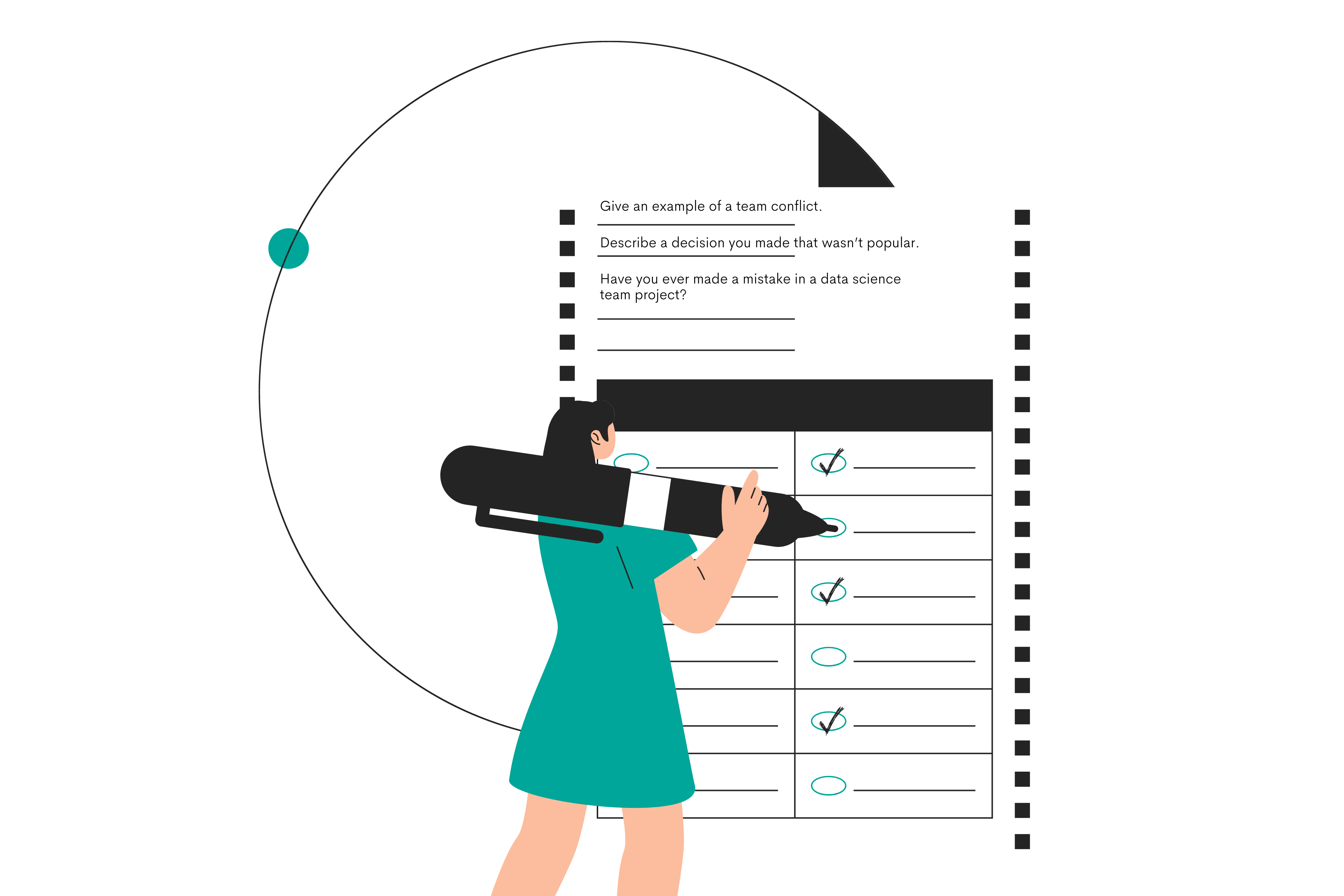
These questions intend to gain a better understanding of how you would respond to different workplace situations, and how you solve problems to achieve a successful outcome.
The main thing that the interviewers present you with is some sort of question that allows you to showcase how you encountered a conflict and then how you resolved that. The purpose of these questions is to let the interviewer know whether you are the best fit for their team or not.
Below given are some of the typical behavioural questions that are likely to come up in a data science interview:
- How have you used data insights to persuade an opinion?
- Have you ever made a mistake in a data science team project?
- Give an example of a team conflict.
- Describe a decision you made that wasn’t popular.
- Give an example of how you worked in a team.
- How have you used data to elevate the customer experience?
A simple strategy to prepare and handle the data science behavioural questions is broken into the following two parts:
- Select and refine stories
You need to think about your past, what you’ve been through, and can come up with four to five stories that demonstrated some sort of conflict and also demonstrated some sort of resolution. It’s very important that you have your own personal story for answering the behavioural questions because if you are talking in a hypothetical situation like I would have done this, it’s not going to be as memory impacting on the interviewer. Also, they are not going to feel like you have the experience because you don’t have the story to showcase for the question asked.
- Implement Stories into STAR Framework
The second part is to implement the stories into a STAR technique to answer the question given. So, what is a STAR technique? STAR is how you set up a storyline in order to answer the question in a better and effective manner.
- S - Situation
First, start with a situation for the interviewers to understand what is the context of the storyline. - T - Task
Let the interviewers know about your roles and responsibilities in that storyline. - A - Action
Then, move into the actions and let them know what actions you took and what you did not take. - R - Result
Finally, the most important thing is the result. Let the interviewers know what type of beneficial result came out of your action.
So, at first, you need to have four to five stories ready to go and then you can use the STAR technique to practice implementing them for effectively answering the behavioural questions in a data science interview.
Data Science Interview Preparation Tip # 04 - Practice Machine Learning, Statistics, and Modeling Questions
They are generally non-coding questions but the interviewer is trying to test your technical knowledge on both the theory and implementation of these three types of questions. So the questions that the interviewer asks generally fall into one or two buckets:
- Theory part
- Implementation part
Focus on theory and learn how to implement it
So, do you know how to improve your theory and implementation knowledge? What I can suggest is that you must have a few personal project stories. By few, I mean that you should have two to three stories where you can talk in detail and in-depth about a data science project you’ve done in the past. Furthermore, you should be able to answer questions like:
- Why did you choose this model?
- What assumptions do you need to validate in order to use this model correctly?
- What are the trade-offs with that model?
If you are able to answer these questions, you are basically proving to the interviewer that you know both the theory and have implemented a model in the project. The project can be an academic project, a personal project, or any project that you’ve done in your recent job. So, some of the modeling techniques that you may need to know are:
- Regressions
- Random Forest
- K-Nearest Neighbour
- Gradient Boosting and more
Explain your projects to the interviewers
These are the common models that every data scientist must know and should have experience in implementing them. So, the best way to showcase your knowledge is by talking about your projects to prove to the interviewers that you’ve got your hands dirty and have implemented these models. Further, if you want to be an effective data scientist, then in addition to just implementing the models, you need to clean the data, build a data pipeline, interpret the results, and communicate the results to the stakeholders. So, if you prove to the interviewer that you know the entire data science process from end to end i-e; from obtaining the data all the way to explaining the results to the stakeholders and explain in detail exactly why you performed each step, then the interviewer would be definitely satisfied in knowing that you are able to complete data science projects.
Now, let’s discover a question asked by Amazon in an interview.
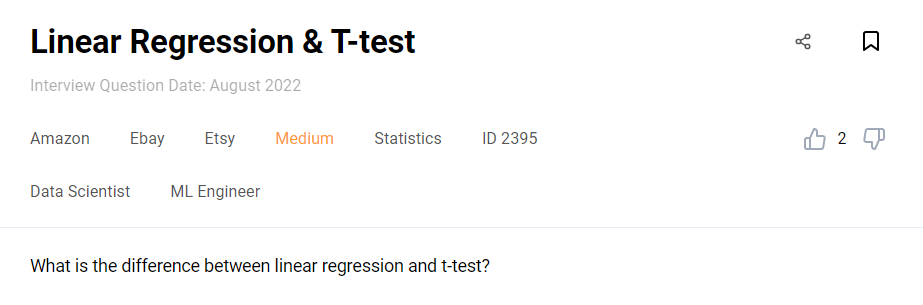
In this question, Amazon asks the difference between linear regression and t-test. "What is the difference between linear regression and t-test?"
Linear regression and t-tests are both statistical methods of data analysis, although they serve differently and have been used in different contexts.
Linear regression is a method for modeling the connection between two or more variables by fitting a linear equation. It is commonly used for predicting the value of a dependent variable based on one or more independent variables. Linear regression may be applied to continuous data, such as the link between age and income.
On the other hand, a t-test is used to find out whether the means of two groups of data are significantly different from each other. It is generally used to compare the means of a continuous variable between two groups, such as the mean longevity of men and women in a population.
In summary, linear regression is used to model the relationship between two or more continuous variables, while t-tests are used to compare the means of two groups of data.
Data Science Interview Preparation Tip # 05 - Doing General Preparation
How do you actually prepare for a data science interview? This is one of the major challenges because there are a whole host of problems everywhere on the internet and you have to follow an organized and structured process in preparing for your data science interview.
How to prepare for a long-term data science interview that’s two to three months out and short-term interview in terms of the night before?
How to prepare for a long-term data science interview?
For a long-term interview, I would suggest you break down the questions into several sections like :
- Machine learning models
- Statistical questions
- Data science questions
- Modeling questions
You have to clearly separate the questions like pre questions, post questions, and some videos and content in between that you can study. Then try the pre section, see how you do on them, where your weaknesses are, write some notes on them. Basically, the aim is to keep track of where you are weak, fast or slow so that you can get to know which part you need to practice more. If you are not keeping track of what you’ve studied and where you are weak, it’s going to be really hard for you to improve because you have no idea where to improve. So, focus on the questions you get wrong to know where you need to improve.
How to prepare for a short-term data science interview?
For a short-term interview, I would suggest you not to study because it’s the night before you need to relax. Get a full night's rest and have a good meal the next day. You need to be at your peak strength and if you’ve worked out really hard the day before, you’re likely just going to be very depleted and exhausted to give an interview. So, be relaxed and confident because that’s how you’re gonna perform at your best.
Points to Remember - An important part of this data science interview preparation guide
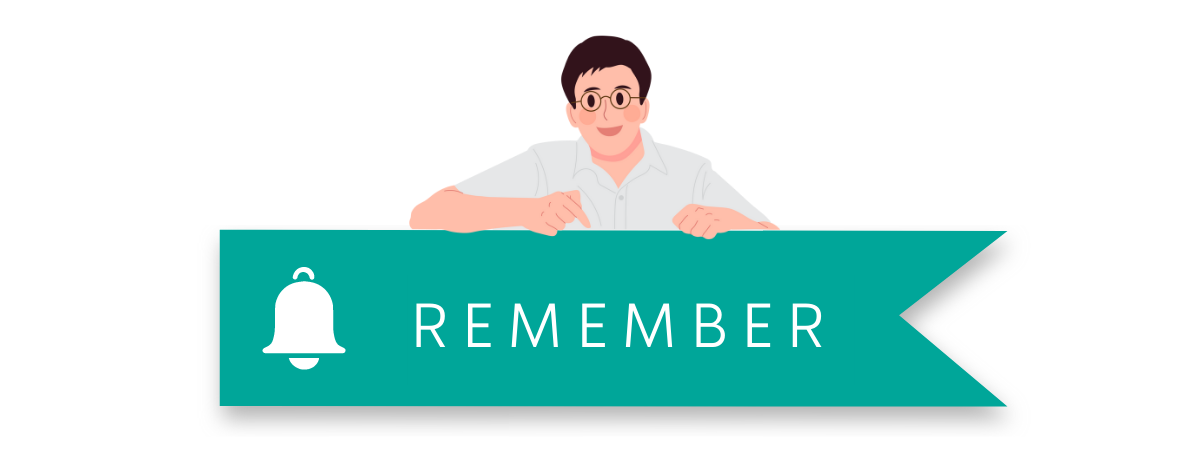
We have discussed some of the important data science interview preparation tips that can help you ace the data science interview. Now, we need to remember the following points at our fingertips before applying for our desired role.
- For data science roles, companies care a lot about technical abilities. The candidate must remember to brush up on optimizing queries, memorizing as many machine learning algorithms as possible, and solving algorithms.
- The candidate must remember fundamental machine learning concepts, modeling, and business case questions. This is because employers might ask some vague questions in which the candidate will be expected to apply machine learning to a business scenario.
Conclusion
We have discussed how to crack a data science interview by showcasing leadership skills, professionalism, good communication, and technical skills. But if you come across a situation during the interview where the recruiter or the hiring manager points out your mistake, do not get shy or afraid to accept it. You are a human, and a human is a statue of mistakes, so accept your mistake as it will portray you as a mature person open to criticism and open to learning. Being stubborn and arguing around will not help because as much as your technical skills are important, your organizational behavior and soft skills matter equally when getting hired for a data science job.
We also recommend checking out our previous guide on how to write a perfect data science cover letter because a well-written data science cover letter can also help you stand out from others.
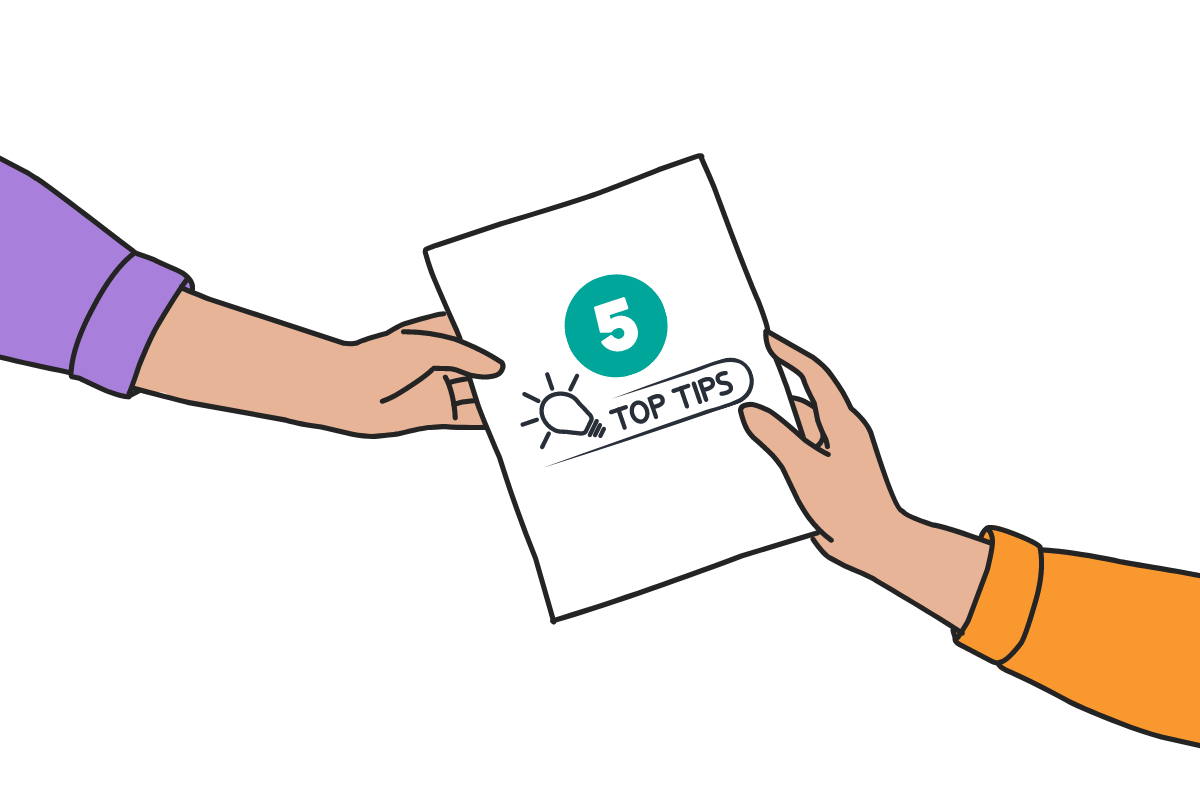